Enveda Biosciences: Unlocking Our Planet's Chemistry
The company's origins, evolution, and recent advances
Welcome to The Century of Biology! This newsletter explores data, companies, and ideas from the frontier of biology. You can subscribe for free to have the next post delivered to your inbox:
Enjoy! 🧬
Biopharma is a strange business. Drug candidates routinely fail in clinical trials at a rate that would be unacceptable in most other industries. Why should we accept this as the norm? What if we could produce medicines in such a reliable way that their clinical success was unsurprising? Where should we be searching for new medicines in the first place?
These were some of the existential questions about drug discovery that Viswa Colluru, the founder of Enveda Biosciences, asked himself while the idea for the company was still just a tiny seed in his mind. Over time, a combination of distinct experiences throughout Viswa’s life provided a rich and unique soil for this idea to germinate into something much more significant.
The core idea of Enveda is to revive the pharmaceutical industry’s focus on natural product discovery—the historical source of many of the world’s greatest medicines.
Natural products are chemical compounds produced by living organisms. They are the ingredients in Nature’s medicine cabinet. We’ve been successfully using these compounds to treat disease for a really long time. For over 2,400 years, people have used ingredients from willow trees for medicinal purposes. Through the lens of modern chemistry, we know that this is because willow trees produce an important precursor to aspirin—one of the most widely used drugs of all time. While it’s a remarkable story, it isn’t a unique one. Natural products are the foundation for many of our most potent medicines, such as taxol, metformin, artemisinin, and statins.
Despite this rich history, the pharmaceutical industry has moved away from natural products in recent years. While it was estimated that 30% of newly approved drugs between 2000 and 2020 were natural products or their derivatives, this number is in decline.1 This trend has several drivers. Isolating, screening, and characterizing natural compounds can be challenging. The reward for this hard work can be nominal if it isn’t possible to patent the original chemical matter that is discovered. As synthetic libraries of small molecules have emerged as the primary basis for modern drug discovery, dogma around the ineffectiveness of natural products has become widespread.2
This dogma is exactly what Viswa and the Enveda team seek to challenge. They believe that we should unlock our planet’s chemistry—making it possible to produce potent new medicines so effectively that clinical trial wins are no longer a surprise but the default outcome. To advance this mission, the company uses large-scale metabolomics and AI to accelerate the natural product discovery process dramatically. They have emerged as an extremely fast-moving and well-financed young company, having raised $175M since being founded in 2019.
This past week, Enveda published a preprint describing a new AI model for predicting chemical structures directly from mass spectrometry measurements. This is a really cool technology—no doubt about it. We’ll look at how this model can be used to explore the massive universe of chemical dark matter, but we’ll start by rewinding the story to the very beginning. How did Enveda get to where it is today, having started off with no IP, no core technology, and only $225,000 in funding?
Here’s where we’re going to go:
Enveda’s origins
Innovating with constraints
The early platform: mapping chemical knowledge
Living up to the name: enabling new chemical knowledge
Let’s jump in! 🧬
Enveda’s origins
Unlike most biotech origin stories, Enveda wasn’t launched from an academic lab or a venture capital fund. Instead, Enveda began its life as a set of astute observations inside the mind of Viswa Colluru, who at the time was a product manager at Recursion Pharmaceuticals. Recursion is a unique business: it is one of the first AI-driven drug discovery companies to gain substantial traction and go public.
The core thesis of Recursion is to use machine learning and high-throughput imaging and measurement of cells to transform the phenotypic screening process for new drugs. It has been a bold and capital-intensive approach, and we are in the early days of observing whether or not Recursion can successfully accelerate the discovery process. That said, Recursion has unequivocally achieved a scale of data generation and computation previously unseen within the pharma industry.
As an early employee at a drug discovery company trying to do things so unconventionally, Viswa soaked up several lessons. Over time, he’s distilled it down to three core insights:
Challenge dogma — If 90% of drugs fail in clinical trials, we clearly haven’t found the best possible way to discover drugs. Be willing to think differently.
Think from first principles — If you aren’t starting with existing assumptions, you should explore the entire space of possible solutions to hard problems.
Things that work in the lab aren’t guaranteed to work in people — this is the fundamental problem that all new discovery companies should try to move the needle on.
For Recursion, the core focus is to build new digital representations of biology. The hope is that these new representations of human biology can identify the best possible drug targets and provide more certainty around the behaviors of new drug candidates, thereby increasing clinical trial success rates.
Over time, Viswa started to flip this problem around on its head. What if we should actually create digital representations of chemistry to dramatically improve our selection of the molecules we’re screening in the first place?
When thinking about where in chemical space we should be looking, another aspect of Viswa’s background had a significant impact. Growing up in India, Viswa’s dad ran a small allopathic pharmacy that sold plant-based Ayurvedic medicines directly alongside prescription drugs. When he or his family members got sick, these natural products were often the first line of defense. He knew from firsthand experience the relief that plants could provide.
Hearing this part of Viswa’s story reminded me of one of my favorite quotes from the science fiction author Hugh Howey: “For me, true creativity is seeing the individual human like a filter, like a coffee filter. You push all this stuff through: popular culture, life experiences, upbringing, genetic makeup. What drips out is the way they distill all that knowledge and all those experiences.” For Viswa, the distillation of his childhood experiences in India and his time at Recursion was the idea for Enveda: a modern biotech platform for natural product discovery.
Over time, this idea began to grow into something more substantial. At the chemical level, natural products represent a distinct subset of the universe of possible molecules. Natural products are typically heavier than their synthetic counterparts, contain more oxygen and less nitrogen, and are more rigid. They are often orally consumable, despite defying many of the chemical rules used to produce oral drugs.3
Viswa consistently came across a distinct cognitive dissonance from drug makers in more than fifty discovery interviews. They agreed that natural products should be pursued further but knew it wouldn’t be possible to convince decision-makers within their organizations to take action. A renewed focus on natural products would be incompatible with the existing investments and tailwinds within most pharma companies.
Viswa realized that this was his alpha—the idea was contrarian enough to give him a real head start, meaning he could capture substantial value if he succeeded. This was actually a scary realization. Given that Viswa was in the United States on an employment-based visa, leaving his job to pursue a startup was associated with real risk. As he became physically uncomfortable with anxiety over what to do, he got advice from his sister, who told him, “Life doesn’t reward you for what you know; it rewards you for what you do.”
He decided to take the leap. With Recursion CEO Chris Gibson’s blessing, Viswa became the first member of the “excursion” crew and founded Enveda Biosciences.
Innovating with constraints
Without the benefit of spinning out with IP from a famous academic lab or being launched by a big VC, Enveda’s started with a humble set of resources. Viswa invested a chunk of his savings into the business to get it going. Martin Brenner, who had been impressed by Viswa during his stint as the CSO of Recursion, contributed an angel check. With additional investments from family and friends, Enveda started with $225,000 on its balance sheet.
How can you possibly start a biotech business with this little capital? Small angel rounds are common in software, where a small team can build a product prototype with no real operating costs. In biotech, where lab space, equipment, reagents, and Ph.D. level employees are prerequisites, it’s not uncommon to see companies start with big $50M rounds.
Constraints can engender creative solutions. To make the most of this initial money, Viswa knew he wanted to avoid two things:
Office space — Starting in 2019, before the pandemic, it was still unorthodox to think about building a distributed team, but it didn’t make any sense to burn Enveda’s first money on an expensive office lease.
The SF premium — San Francisco is a magical place for biotech. Still, the cost of hiring engineering or scientific talent from one of the most competitive regions would be too high at this stage.
Instead, Viswa reached out to several Contract Research Organizations (CROs) in India for quotes on some initial experiments. He was quoted for roughly $200,000.
Realizing that he couldn’t spend his entire pre-seed round on one set of experiments, Viswa got on a plane and flew to India. He decided to build out a lab site there and hire an early scientific team. By launching this initial operation, Enveda could run experiments at roughly 1/7 the cost and 1/5 of the time relative to the initial CRO quotes.
With a lab operation up and running, it was time to iterate on the earliest ideas within Enveda for efficiently exploring Nature's massive chemical space in the search for new drugs.
The early platform: mapping chemical knowledge
Scientists love maps, and with good reason. As I wrote when covering the completion of the human genome:
Maps are an essential tool for exploration. They provide a common set of coordinates, enabling navigation and direction. Another key feature of maps is their ability to keep track of where we have already charted, and where we have yet to explore.
To identify new natural compounds worth being tested, Enveda desperately needed a coordinate system to structure the existing landscape of chemical knowledge. For efficient search, you need a map of the known knowns and the known unknowns worth testing.
Viswa’s earliest idea was to start by developing algorithms to draw links between chemicals, the proteins within cells that they perturbed, and the disease modifications these perturbations produced. After scouring the literature and realizing he first wanted to try using knowledge graphs, Viswa knew that he needed help putting this idea into practice.
Here, one of the core tenets of Enveda came into place: people create all value. Enveda is willing to recruit globally for the ideal candidates with the skills required to advance its core mission. A central job for any founder is to attract top talent. New knowledge and medicines don’t appear out of thin air. As Joshua Boger of Vertex once said, “Pharmaceutical discovery and development is the most complicated activity that humans do.” By definition, a pursuit of this level of complexity requires a wide range of highly skilled humans operating in unison.
Given the lack of geographical constraints on hiring, Viswa managed to recruit Daniel Domingo-Fernández, a talented German computational biologist with expertise in knowledge graphs. With Daniel onboard, Enveda pushed forward on the first algorithms for their internal Knowledge Graph for drug prioritization.
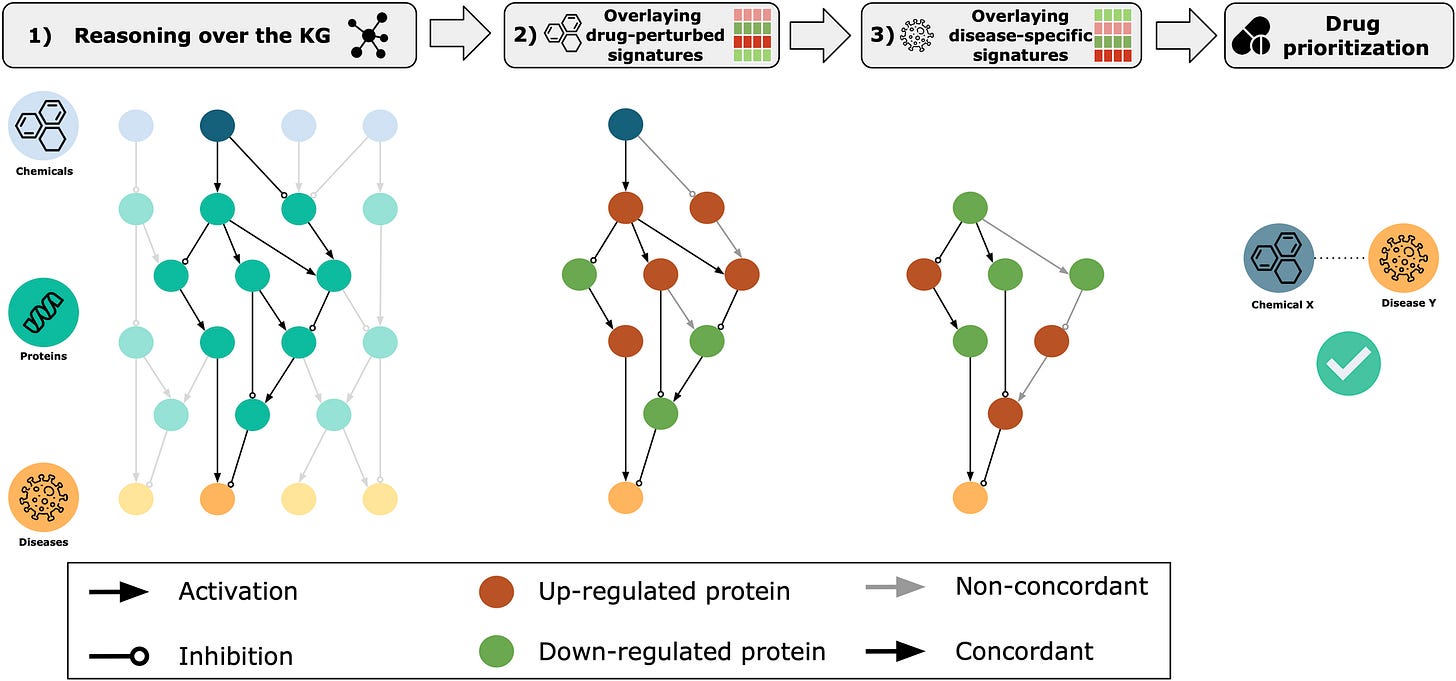
This type of knowledge graph can be used to draw connections between chemicals and their potential drug-like behavior. As Viswa frames it, knowledge graphs “provided a way to create a computable map of human ethnobotany and to represent chemical knowledge.” With this foundation in place, Enveda could turn it’s attention to uncovering new natural products to perturb the most promising nodes in the graph.
Enveda does this by exhaustively sampling the chemistry of plants.
The central sampling technology is tandem mass spectrometry (LC-MS/MS). Let’s break down how this works. You can't measure an intact sample if you want to distinguish individual chemicals. Plant samples are separated into their individual chemical components using liquid chromatography (LC). This works by dissolving the samples into a liquid solvent that flows through a measurement instrument with a solid stationary phase—chemicals remain in this phase for different lengths of time, making it possible to separate them.
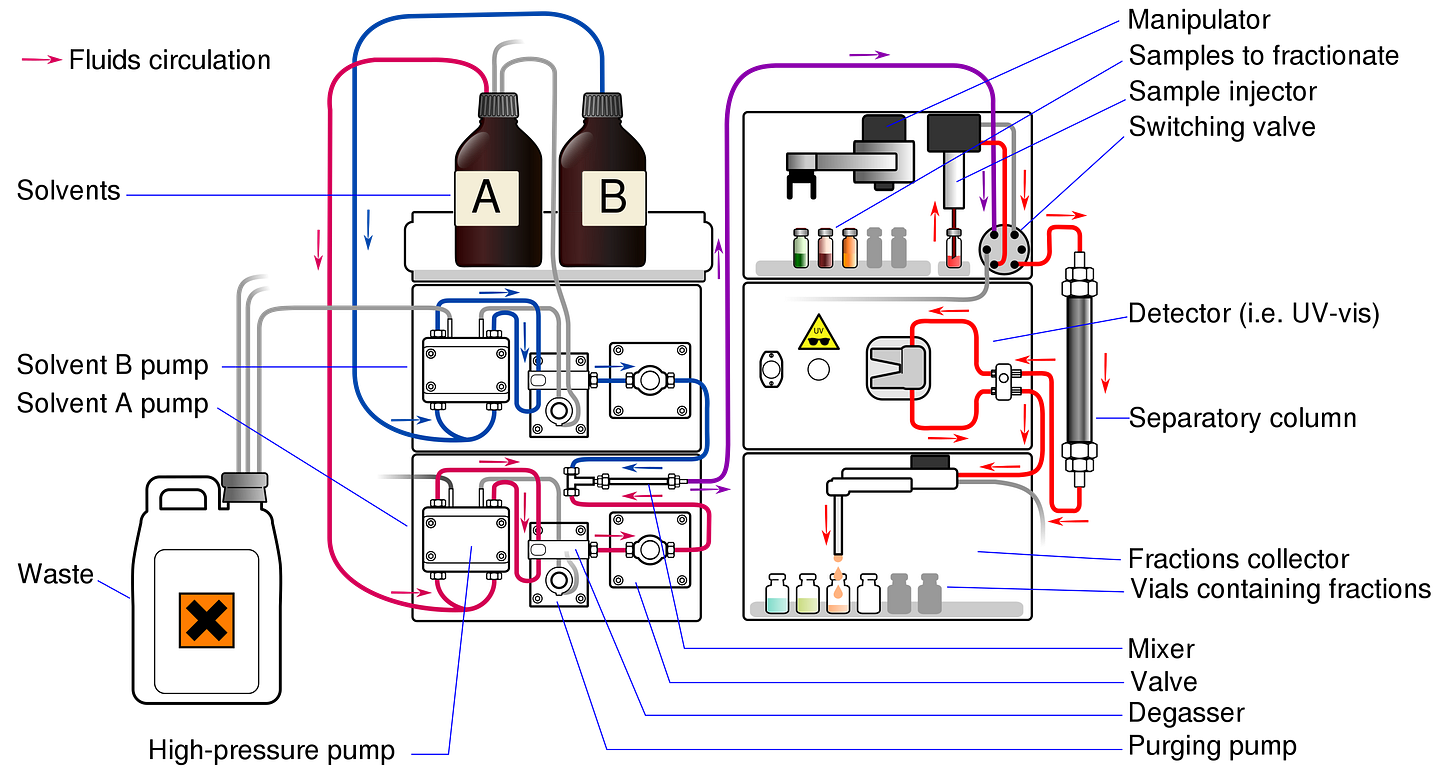
With the individual chemicals separated, it’s possible to actually measure them. This is done by the mass spectrometry (MS) instrument, which works by giving the molecules a chemical charge (ionization) and then separating them by their mass-to-charge ratio (m/z). To be more sensitive, a portion of the ions are fragmented and then measured again—hence the name tandem mass spectrometry (MS/MS).
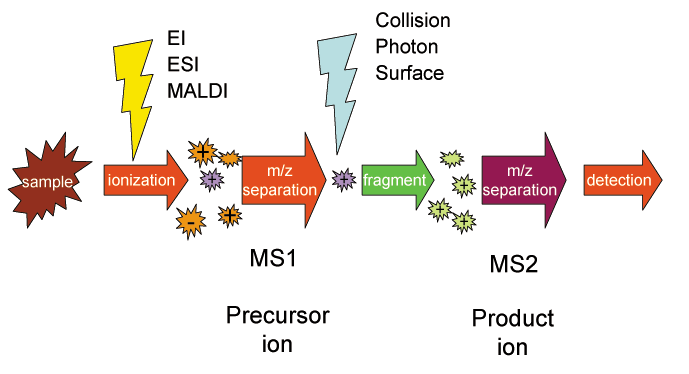
For the data to be useful, the raw mass spectra need to be converted into the actual chemical structures that were measured. It’s actually possible to do this mapping by hand because the unique composition of the m/z ratios narrows down the set of candidate structures. This process often requires integrating information from another chemical measurement technique called nuclear magnetic resonance spectroscopy. Outside of undergraduate organic chemistry labs, this spectra-to-structure task is usually done algorithmically.
Here, a major measurement bias is introduced. Nearly all algorithmic approaches for this task rely on reference libraries of known spectra-compound pairings. Essentially, you rely on a lookup search from an incomplete reference dictionary. All of the chemical matter outside this predefined space is referred to as chemical dark space because it evades detection. For reference, we’re talking about a discrepancy that spans several orders of magnitude. One of the primary natural product databases consists of slightly more than 400,000 structures—a blip in a chemical space thought to contain billions of unique molecules. Estimates vary, but we’ve sampled as little as 0.1% of the planet’s existing chemical matter.
This is a fundamental bottleneck if the entire goal for Enveda is to discover novel chemical compounds. This mission is even baked into the name of the business. Veda is the Sanskrit root word for knowledge. In conjunction with the en- prefix (short for enable), the founding goal is to enable the creation of new chemical knowledge. In doing so, this deeper chemical understanding would enable more widespread use of humanity’s existing knowledge of medicinal plants.
With this basis for the company, a crucial question emerged: how can we move beyond reference libraries and discover new chemical matter?
Living up to the name: enabling new chemical knowledge
If I were asked to describe the magic of deep learning in two words, I would choose function approximation. This sounds unsexy and a bit technical, but it’s extremely powerful. Historically, we’ve programmed computers by writing functions:
def add(a, b):
return a + b
This works well for simple tasks and affords tremendous precision. With modern programming languages and concepts, it’s possible to implement remarkably complex functions. Still, there are countless functions that are effectively impossible for humans to write. What does the written function look like to do superhuman image classification?
Thankfully, we’ve invented a new programming paradigm for tasks like this: deep learning. With enough data and the right model architecture, we can learn phenomenal approximations of highly complex functions like natural language generation and protein structure prediction.
In the preprint I mentioned earlier, we can see how Enveda has framed its mass spectrometry problem as a function approximation problem and achieved impressive results. While most approaches for resolving structures from mass spectra rely on databases, the alternative approach is to directly predict the chemical structures from the raw measurements. Enveda has created MS2Mol, a state-of-the-art Transformer model for making this type of de novo structure prediction.4
Why does this work? The critical insight here is that there is actually an underlying function between raw mass spectra and chemical structure that can be approximated. Remember, organic chemistry students are forced to do this type of mapping by hand. Here’s Enveda’s description in the preprint:
Natural language frameworks have been observed to be valuable for interpretation of mass spectra, since the meaning of mass fragments are contextually dependent on the presence and absence of other fragments, and the fragments are composed into an overall spectrum in a similar way to words in a sentence.
This is beautiful. Personally, I’ve found it remarkable how many problems in the natural sciences can be formulated as language translation problems. We’ve seen how Protein Language Models can approximate the complex language of proteins. Now, this new work provides further evidence that we can learn to approximate the underlying language of chemistry. Our universe reveals its secrets to us in many tongues.
Here’s what this looks like in practice:
Let’s step through this.
On the left, we have an example of a raw mass spectra plot, where the mass-to-charge ratio (m/z) is plotted on the x-axis, with signal intensity on the y-axis. The raw spectra are sorted by intensity and then processed into integer tokens representing underlying chemical fragments. These tokens are the vocabulary representation of the mass spectra—but just like in natural language processing, machine learning can’t work with a raw bag of tokens. Instead, the tokens get transformed into a big vector of continuous numbers that effectively represent the original information in a more compressed numerical format.5
This embedding gets fed into an encoder-decoder network that uses the BART architecture introduced by Meta AI for natural language processing. This model fuses two major building blocks for language modeling into a unified framework.
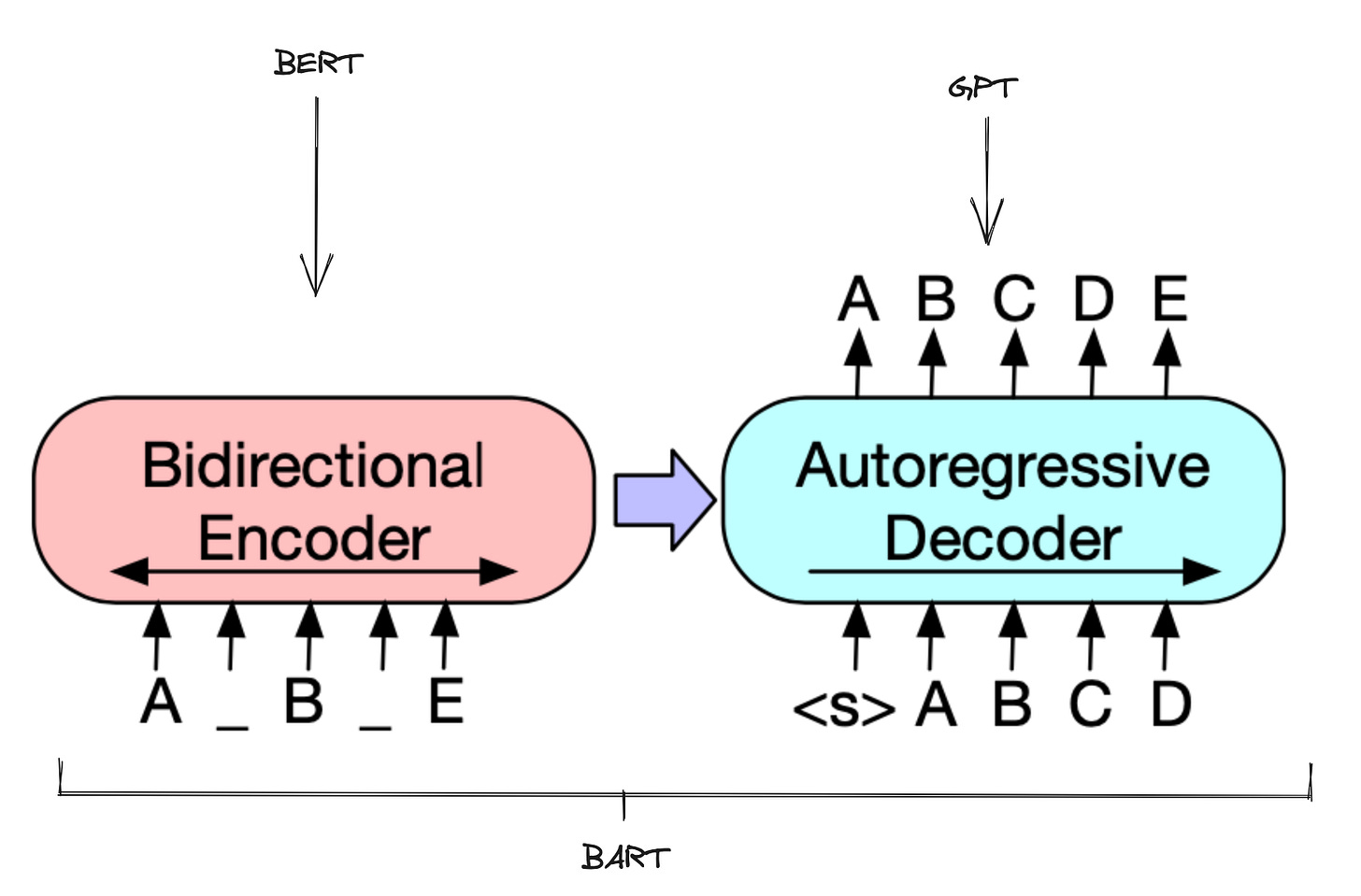
To infer the final structure, M2Mol uses an algorithm called beam search to produce 25 different predictions of the molecular formula and the chemical structure. At this stage, structures that violate the fundamental laws of chemistry can be tossed. A smaller model was trained to rerank the predictions, with the top-ranked structure serving as the final prediction.6
Another essential detail of this approach is the way that the actual output structures are represented. The output is a SMILES string, which is a way to represent a molecular structure as a sequence of characters:
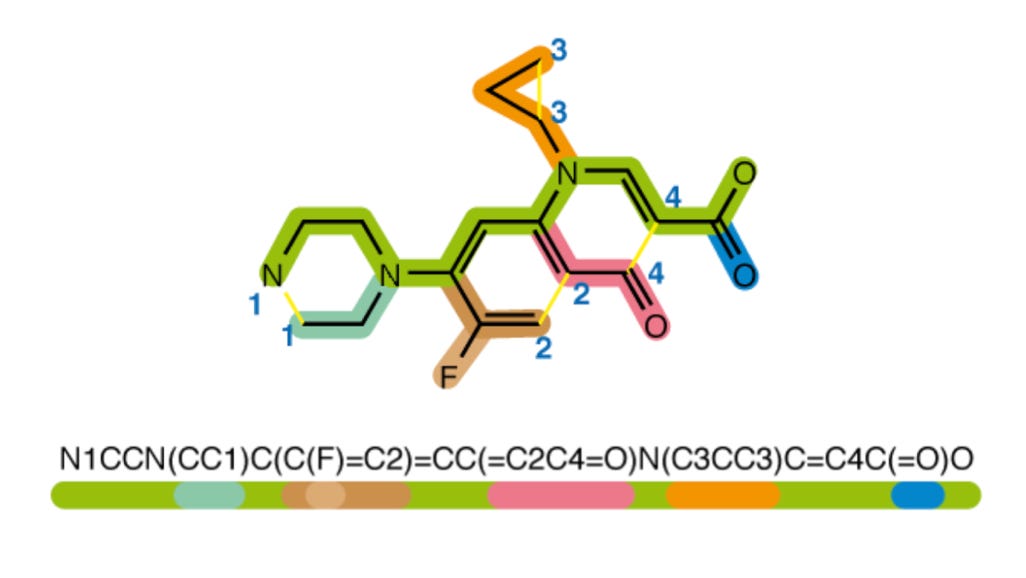
To simplify the character set that could be used to compose these strings, the MS2Mol model uses a technique called byte-pair encoding, which groups pairs of frequently neighboring characters into single tokens. This seemingly trivial detail dramatically improved model performance.
For computational biologists, the devil is in the details. While other groups have developed approaches for de novo structure generation from mass spectra, combining these clever components into a new framework produced state-of-the-art results.
As we can see, MS2Mol predicted a “close match” to the ground truth structure for 21% of spectra and a “meaningful match” for 62% of spectra.7 Clearly, these results are far from perfect, and very few results were exact matches to the ground truth structure. Despite its imperfections, this model can serve as a valuable tool for enhancing our ability to detect portions of otherwise completely undetectable chemical space.
As David Healey, VP of Data Science at Enveda and senior author on the new preprint, wrote in his excellent blog post on MS2Mol: “While an exactly correct structure is the ideal, dark chemical space is so big that even incremental improvements to this initial characterization stage can go a long way to telling us what the chemical space is and allows us to prioritize molecules for drug discovery purposes.”
The metabolic measurement and structure annotation steps comprise the first half of Enveda’s discovery platform:
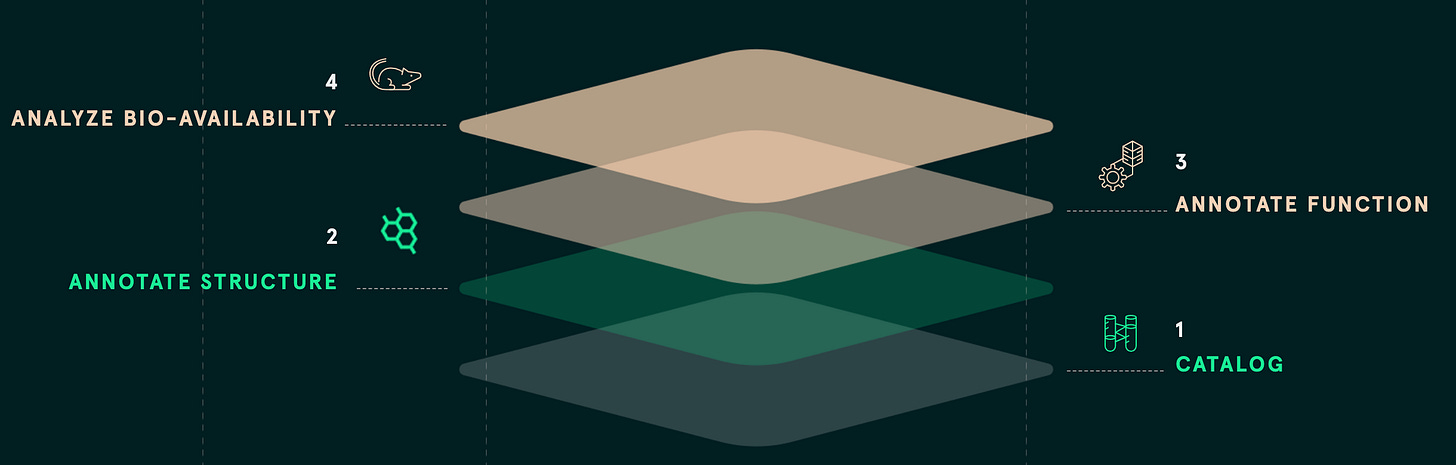
Extending beyond the earliest iterations on the computational Knowledge Graph, Enveda is building a custom high-throughput screening for in vitro and in vivo testing of the compounds in their chemical catalog. These subsequent empirical measurements help to rapidly test important drug characteristics such as its functional properties and where it ends up being distributed in the body.
The central purpose of this platform is to unlock our planet’s chemistry.
Enveda’s thesis is that their tech-enabled exploration of plant natural products will uncover a broad panoply of valuable medicines. We won’t have to wait too much longer to see clinical readouts for the company—the plan is to file their first three Investigational New Drug (IND) applications by the end of the year. After that, several additional drug candidates will be fast followers:
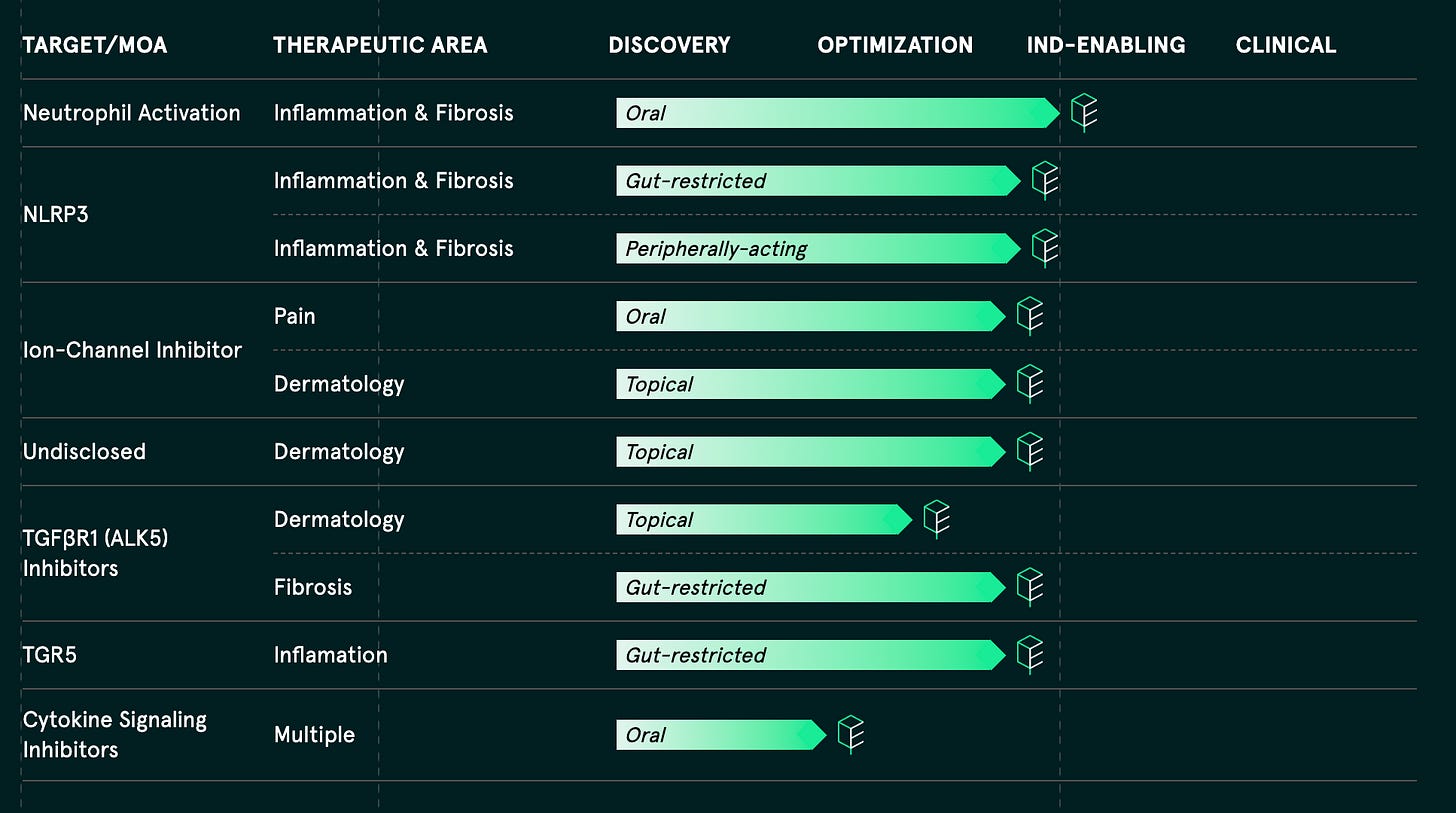
We can learn two things from looking at this pipeline:
The initial indications are clustered around pain, itch, inflammation, and dermatology—areas where natural products have historically shined. The goal is to play to the platform’s strengths early on. This is an interesting strategic pivot away from some of the more complex diseases Enveda initially targeted, with the goal of minimizing “biological risk, feasibility risk with large and complex diseases, as well as ultimately commercial risk.”
Enveda wants to be a full-stack pharma company. They don’t want to put all their focus into their top two assets—they aspire to be a generational drug company with their own portfolio of products. Previously, Viswa has said, “Our goal is to be a vertically integrated pharma company that predominantly spends its efforts building the technology to harvest assets for internal development.”
This helps explain why Enveda has raised so much capital early on and why they haven’t publicly announced any major pharma partnerships. The North Star of a company dictates the strategic decisions that it makes. The primary focus for Enveda is to invest in the best possible people, expand its platform’s capabilities, and create a broad internal drug pipeline.
Enveda’s story highlights many of the ways that biotech is evolving. The company started as nothing more than a good idea inside the mind of an early employee at a company using AI to accelerate drug discovery. With a small angel round of $225,000, Enveda de-risked their early ideas in the most frugal way possible—in some ways operating more like a founder-led tech company than a biotech company.8 Over time, they’ve raised capital to unlock very specific milestones. At each stage, the company’s momentum has compounded. Enveda has now snowballed into a company with more than 170 employees organized in a distributed archipelago around the world.
While Enveda’s growth, financing trajectory, investor pool, and computational focus highlight the growing TechBio trend, the company’s core thesis is fundamentally contrarian. Many people—myself included—think that the future of medicine will increasingly revolve around programmable biologic therapies. Even in the world of small molecules, Enveda sees things very differently.
The thesis is a blend of humility and hubris. Enveda believes that Nature may be a better drug chemist than us and that we run the risk of missing out on many potent medicines if we overlook natural products. Pursuing this direction also requires believing that their technology will enable them to learn the language of chemistry and succeed where others have failed.
It will be fascinating to see what this blend of Natural and computational intelligence will be able to produce.
Thanks for reading this essay about Enveda Biosciences. If you don’t want to miss upcoming essays, you should consider subscribing for free to have them delivered to your inbox:
Until next time! 🧬
It’s worth noting that natural product research consumed nowhere near 30% of the R&D budget for biopharma over this time period, which means the return on investment has been unusually good.
Even outside the world of small molecule discovery, natural products have to fight for airtime with the growing wave of biologics—peptides, antibodies, cell and gene therapies, and more.
If you’re a scientist wondering about the chemical details of the argument in favor of natural products, here’s an excerpt from a high-quality review that details their chemical properties: “NPs are characterized by enormous scaffold diversity and structural complexity. They typically have a higher molecular mass, a larger number of sp3 carbon atoms and oxygen atoms but fewer nitrogen and halogen atoms, higher numbers of H-bond acceptors and donors, lower calculated octanol–water partition coefficients (cLogP values, indicating higher hydrophilicity) and greater molecular rigidity compared with synthetic compound libraries. These differences can be advantageous; for example, the higher rigidity of NPs can be valuable in drug discovery tackling protein–protein interactions. Indeed, NPs are a major source of oral drugs ‘beyond Lipinski’s rule of five’”
I’m not going to explain the details of Transformer models here. I’ve unpacked some of the concepts in previous posts, but if you’re excited about AI and this is a new concept, The Illustrated Transformer article is hard to beat for a starting point.
As for the Transformer, it’s worth checking out the Illustrated Word2vec if you want to go deeper on embeddings.
To be more useful for drug hunting, there’s also an additional model that predicts the confidence of the M2Mol output—the highest confidence predictions can be used for further analysis.
These labels were based on creating a mapping between standard metrics for chemical similarity with a set of molecules annotated by experts for how useful they would be for resolving the actual structure.
Sometimes, the old becomes new again. When Robert A. Swanson and Dr. Herbert W. Boye first pitched the idea for Genentech to Kleiner Perkins, they asked for $500,000. While Tom Perkins was excited by the idea, he initially gave them only $100,000 to run the essential experiments needed to “eliminate the white-hot risk” before expanding further.