Genome-informed cancer therapy
A new machine learning platform for detecting DNA alterations in cancer
Cancer, perhaps, is an ultimate perversion of genetics—a genome that becomes pathologically obsessed with replicating itself.
- Siddhartha Mukherjee
Introduction
As we transitioned from sequencing the first human genome to sequencing a multitude of genomes, cancer was an obvious research target. For decades prior to the completion of the Human Genome Project (HGP), it had been known that DNA mutations drive cancer development. Two years after the completion of the HGP, a “Human Cancer Genome Project” was proposed. This ultimately took the form of the The Cancer Genome Atlas, which aimed to develop an expansive catalog of cancer sequencing data—with the goal of establishing a more comprehensive global understanding of cancer genetics. Cancer genomics has arguably been one of the biggest success stories of the post-genome era, as it has genuinely transformed how we understand and treat cancer.
Something incredible has happened as DNA sequencing costs have continued to decline at an absurd rate. Sequencing has transitioned from being exclusively used as a research tool to being an increasingly important part of patient care. It has been estimated that 60-90% of cancer deaths are due to metastasis—the stage of disease where cancer is spreading to secondary sites from where it originated in the body. In other words, more advanced stages of cancer are far more lethal. Based on this, many researchers and companies have been working towards the “holy grail” of cancer care: an accurate and non-invasive screening tool using DNA sequencing to detect cancer at its earliest stages when it is much easier to treat.
If you’ve been around biology and biotech long enough, this may sound too familiar to the Theranos horror story, but these new approaches are based on legitimate technology (sequencing) and a crucial and reproducible scientific observation: cancer cells shed DNA into the bloodstream.
This circulating tumor DNA (ctDNA) can be isolated from blood plasma, which provides a substrate for detecting cancer at its earliest stages. In addition to its potential use as a screening tool, ctDNA profiling has been used in the clinic to monitor cancer progression and treatment response to inform decision making. While this has primarily been done with targeted panels—where specific regions/genes are deeply sequenced—an exciting recent paper from the Landau Lab in New York proposed a system for more broad whole-genome sequencing (WGS) of cell-free DNA that showed serious improvements.
Less than two years after that publication, the same group has released an impressive preprint entitled “Machine learning guided signal enrichment for ultrasensitive plasma tumor burden monitoring” that demonstrated 300x improvements (!!) in important aspects of the previous platform. They showed various ways that this could significantly improve the clinical utility of this approach.
This study was jointly led by Adam Widman, Minita Shah, and Nadia Øgaard, and the senior authors are Nicolas Robine, Claus Lindbjerg Andersen, and Dan Landau.
Key Advances
In order to understand this technology, it is important to understand its goal. Currently, blood plasma sequencing has been used to inform treatment for patients who have a high tumor burden, meaning that cancer DNA comprises a high fraction of the total DNA in a given blood biopsy. There is another point in cancer care where sequencing could be informative. It could also be used to detect and analyze minimal residual disease (MRD) present after initial treatment. MRD detection could “enable precision tailoring of treatment, offering treatment intensification or de-escalation based on MRD status.”
The key technical challenge that makes this difficult to achieve is the lower tumor fraction (TF) present in residual disease compared to before treatment. The major advance in the last paper from this group was that they were able to detect minimal residual disease (hence the name MRDetect) with much greater sensitivity relative to more targeted panel-based approaches. With a broader sequencing strategy, there was a greater amount of signal.
When integrating information across the entire genome, there is also a lot more noise. In this new study they doubled down on developing a robust machine learning framework to effectively tackle this problem, and to robustly identify true signals. So what are the signals we’re looking for? This platform detects two types of DNA changes: 1) single nucleotide variants (SNVs) which are changes to individual bases, and 2) copy number variants (CNVs) where regions of the genome are repeated. SNVs from the reference genome are likely to be cancer mutations, and variation in copy number is a way to detect aneuploidy—the loss or gain of a chromosome—which is a very common occurrence in cancer genomes.
A primary challenge in accurately detecting SNVs is determining whether a variant is a real measurement or a sequencing error. Based on this, the previous framework used a machine learning classifier to distinguish between real SNPs and sequencing errors. Now, they re-built their framework from the ground up with the goal of adding relevant information that would make it easier for the model to identify true signal—instead of purely focusing on removing noise.
As can be seen above, they worked to create entirely new data representations of the DNA fragments being classified, and architected an ensemble model using a convolutional neural network (CNN) for the fragment and a multilayer perceptron (MLP) to incorporate additional useful features for prediction.
Just like for SNVs, the team went back to the drawing board in order to think of new ways to detect CNVs. To start, they created a denoising approach to remove patterns in the data caused by technical artifacts (both from sequencing and computational analysis) as well as biological background noise. They also detected changes in allele frequency and accounted for expected differences in fragment sizes coming from cancer compared to non-cancerous cell-free DNA.
They trained three separate models—one for each of the inputs above—and fed each one into their new classifier: MRD-EDGE (Enhanced ctDNA Genomewide signal Enrichment). This work is obviously very technical. It uses complex biological feature engineering and machine learning.
The key take-home message is that this new framework takes DNA sequencing data collected from blood plasma as input, and uses it to detect cancer SNVs and CNVs—which can be used to inform treatment.
Results
There are two types of results in this paper. The first set of results evaluates model performance. How well does SNV detection work? How well does CNV detection work? The other set of results evaluates the clinical utility of this approach. How useful is this framework for informing treatment?
The improvement in SNV detection was impressive:
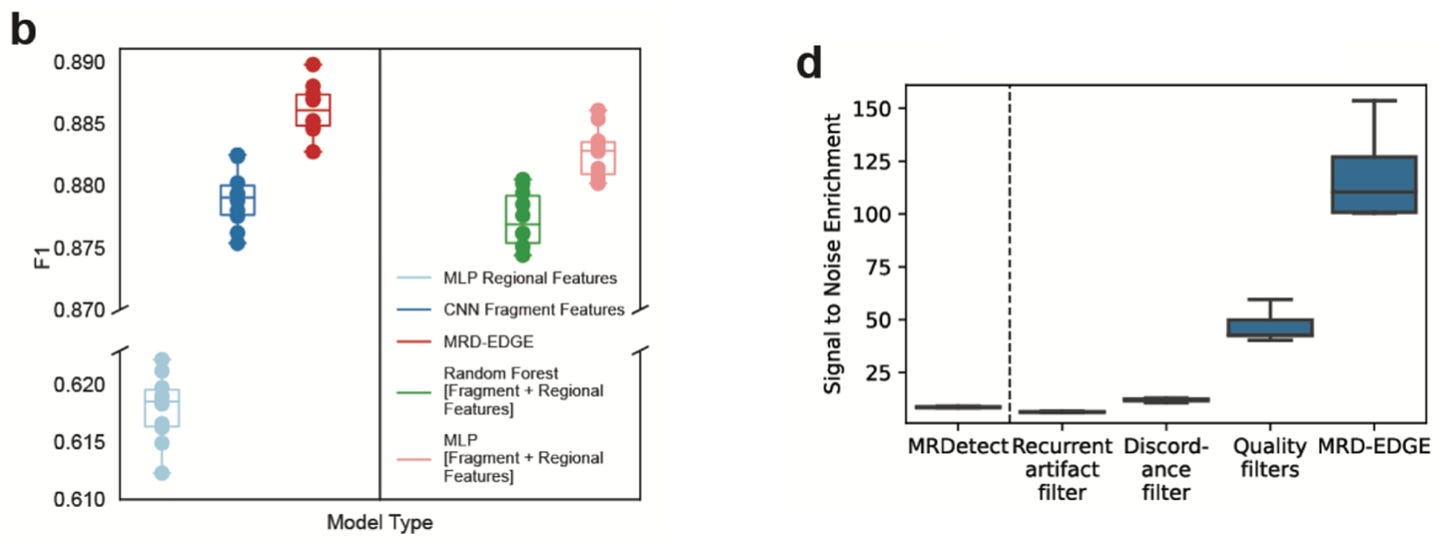
Their new model has a high accuracy for SNV classification, and showed greater than 100-fold improvement in signal-to-noise enrichment relative to their previous work. This improvement resulted in much better performance for low tumor fraction (TF) fragment detection. In their simulation analysis, they saw “higher performance even in the parts per million range and below.” Again, this all corresponds to a much more sensitive ability to detect lower frequency cancer DNA fragments, even after initial treatment.
What about CNV detection? Also very good.
The new model had a far lower error rate in their analysis across all tumor fraction ranges. This result is really interesting, so it’s worth putting into appropriate context. When initially published, MRDetect “demonstrated a 2 order of magnitude improvement in sensitivity compared to leading CNV-based ctDNA algorithms” but the main drawback was that “we required substantial aneuploidy (>1Gb altered genome) to detect TFs of 5*10-5.” Now, less than two years later, they only need a fifth of the amount of altered DNA (200Mb) to achieve the same sensitivity. This technology is rapidly improving.
So far, we’ve looked at feature engineering, machine learning architectures, accuracy metrics and error rates. How does this type of detection actually work for clinical cases? For their case study, they analyzed plasma collected from a set of patients on an immunotherapy protocol. The patients received an immune checkpoint inhibitor (durvalumab) with or without radiation therapy (SBRT). After the initial treatment, the tumors were surgically resected. Let’s see what this looks like for two of the patients without radiation:
For the top patient, their ctDNA levels fell below the detection threshold. 29 months out, they are still cancer-free. For the bottom patient, their ctDNA levels remained detectable. There was detectable disease recurrence 12 months after surgery. This is a demonstration of how this type of post-operative detection of minimal residual disease could be used to dial up or dial down treatment to better prevent disease recurrence. It also demonstrates the importance of all of this intensive methodological work. High accuracy and sensitivity are of the utmost importance when making these types of clinical assessments.
There are a lot of other great results worth checking out in this study, including an analysis of the amount of ctDNA present in precancerous colorectal adenomas.
Final Thoughts
Science fiction is full of medical devices such as the tricorder from Star Trek, or the Medici dreamed up by William Gibson. They typically resemble a common archetype where there is a unified approach to measurement, computation, and treatment based on the results.
In many ways, I think that the technology demonstrated in this type of study has the potential to be even cooler than that. Just think about it: ultra high-throughput DNA sequencers could be used to detect tiny traces of cancerous DNA fragments circulating in our blood, informing our dosing and continuation of sophisticated therapies that are coercing our own immune cells to target cancer.1
Thanks for reading this highlight of “Machine learning guided signal enrichment for ultrasensitive plasma tumor burden monitoring.” If you’ve enjoyed this post and don’t want to miss the next one, you can sign up to have them automatically delivered to your inbox:
Until next time! 🧬
The rate of improvement for cancer liquid biopsies is astonishing. Two questions:
1. Based on your description of MRD and its lower tumor fraction, it seems technically more challenging than cancer screening?
2. In your view, what company(ies) shows the most promise in translating this type of research for commercial use in the clinic?
Great dive into the details.
I think doing a better job detecting residual disease would be a big advance if it lets less patients get treated (eg is more specific than current standard of care.) Doing really early detection of cancer before overt disease is also a big deal.
I wonder how the clinical trials for this stuff would look.