Welcome to The Century of Biology! This newsletter explores data, companies, and ideas from the frontier of biology. You can subscribe for free to have the next post delivered to your inbox:
Enjoy! 🧬
It’s been seventy years since the image of DNA’s double helical structure entered our collective consciousness. In the subsequent years, we’ve cracked the genetic code, invented powerful DNA sequencers, and discovered molecular machines capable of manipulating and programming nucleic acids.
All of this has wide-ranging consequences for life as we know it, but one application is immediately obvious. If some human diseases are caused by genes, can we use the tools of modern genetics to find out which genes are broken and ultimately perform molecular surgery to correct them?
The answer is yes, but it’s hard. In total, the FDA has approved thirty-three cell and gene therapy products for clinical use. Of these medicines, only six work by delivering a functional copy of the defective gene directly to cells in vivo—inside the body.1
While this number isn’t zero, there are thousands of known human genetic disorders, including hundreds of disorders with well-described underlying mutations. What causes the disconnect? Why aren’t there hundreds of approved gene therapies?
There are several reasons. Resources for clinical development are finite, and some of these diseases are so rare that they are less prioritized by drug developers. Still, there is a looming technical challenge that explains a non-trivial amount of the discrepancy:
Delivering gene therapies safely and specifically inside the body is a really hard problem.
Imagine this problem from a gene’s-eye view. You’re a gene that’s just been injected intravenously into a patient with a neurodegenerative disease. You need to make your way from the bloodstream to the brain. The problem of crossing the blood-brain barrier is stressing you out. Assuming you overcome this obstacle, you need to enter the diseased cell type where the defective gene is causing problems. You need to do all of this while evading the immune system, which evolved to seek out and mitigate foreign nucleic acids. Not only that, you need to avoid ending up in other tissues or organs, causing more problems for the patient. It’s a hard job, and you’re starting to wonder why you signed up for it in the first place.
One of the main strategies for shuttling genes into the nucleus of cells in the body is to use viruses. After all, this is what viruses evolved to do. Still, using viruses presents several challenges. They didn’t evolve to be highly specific therapeutic vectors, and there is a constant arms race to avoid immune detection.
To circumvent these problems—and to mitigate the potential risks associated with using a virus—gene therapy developers turn to the tools of molecular engineering. For the most widely used viral vectors, scientists strip out the original viral genome, replacing it with the therapeutic payload. This avoids risks associated with unwanted viral replication. Next, the viral protein shell—called a capsid in virology—needs to be engineered to target specific cells in the body. This is easier said than done.
Dyno Therapeutics was founded with a singular purpose: to solve the problem of in vivo gene delivery by cracking the capsid design problem.
Today, we’re going to dive deep into Dyno’s strategy for doing this. Dyno is a fascinating company. It is riding the tailwinds of exponential progress in genomics, synthetic biology, and AI in the pursuit of solving a Holy Grail problem for gene therapy. To do this, Dyno has created a purpose-built and somewhat contrarian platform strategy—they focus entirely on the problem of solving in vivo gene delivery instead of making their own gene therapies.
Dyno has moved quickly on this vision. Since their seminal publication and company launch in 2019, they’ve announced four partnerships with leading drug makers and have raised a $100M Series A led by Andreessen Horowitz to scale their design efforts. Recently, they’ve also announced two of their first off-the-shelf gene delivery products. This progress hasn’t gone without notice—Dyno has been ranked as one of the top emerging biotech companies to watch.
In order to understand all of this, I’ve spoken with many of the company’s co-founders and investors, visited their labs, read every paper they’ve published, and reviewed countless private and public materials on the company.
Studying Dyno’s thesis and initial efforts in detail reveals many lessons. We’ll examine new measurement technologies, the challenges of biological sequence design, how to pick problems worth solving, and what the horizontal biotech platforms of the future might look like.
The ultimate puzzle
If there’s one thing Eric Kelsic loves, it’s a good puzzle. At first, this fascination was as literal as it could be. As a precocious high schooler in Colorado, he became obsessed with Karakuri boxes—which can only be opened after solving a three-dimensional puzzle, often involving hidden mechanisms or a clever twist. He quickly tired of solving existing boxes and started a small business marketing his own inventions.
Kelsic’s love for tinkering and engineering led him to the sunny campus of Caltech for college. Nestled in Pasadena, Caltech looks and feels like a Temple of Science. Unlike the entrepreneurial hubs of Boston and the Bay Area, companies are few and far between. Scientific knowledge is front and center. And out of all of the sciences, Caltech’s impact on physics has been the most formidable.
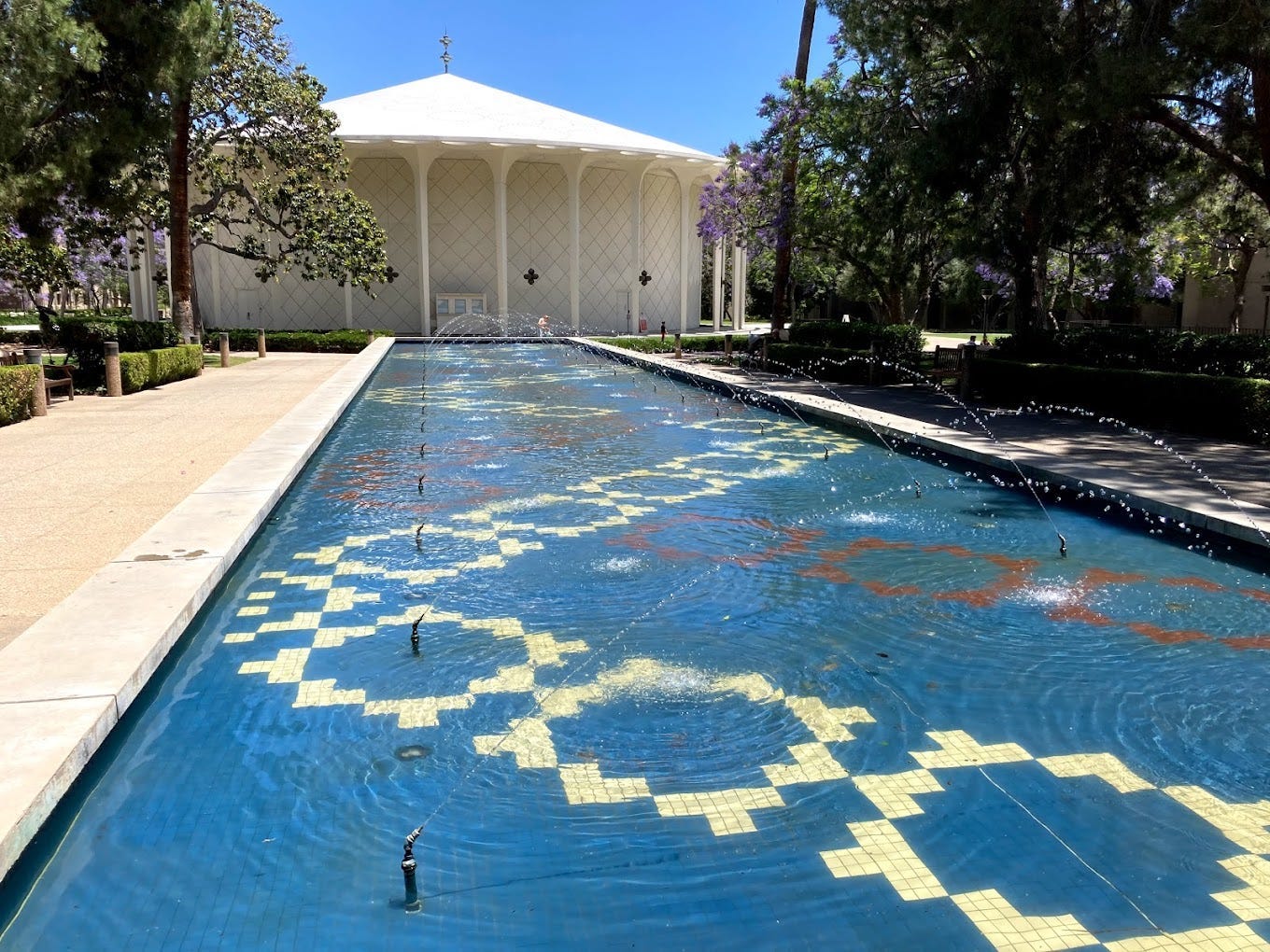
Under the leadership of Robert Millikan—one of the greatest experimental physicists of the 20th century—Caltech’s warm campus served as a central meeting hub for many of the field’s greatest minds. Paul Dirac, Erwin Schrödinger, Werner Heisenberg, Hendrik Lorentz, Robert Oppenheimer, Niels Bohr, and Albert Einstein all paid visits to the “Millikan Institute” for varying lengths of time. After World War II, Caltech’s prowess in physics only continued, with Murray Gell-Man and Richard Feynman laying the foundation for the Standard Model of particle physics as faculty members.
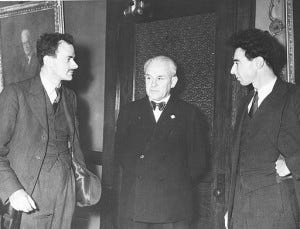
Kelsic heard Feynman’s echoes. Excited by the pursuit of “getting to the bottom of things,” he decided to major in physics. Caltech also offered Kelsic his first taste of basic research. He was completely hooked. Enthralled by the need for open-ended problem-solving, Kelsic proceeded to wander through a variety of research fields, including materials science, cryptography, and complex systems theory.
Over the course of the 20th century, the quantitative and systematic thinking from physics began to spill over into the field of biology. Again, Caltech served as a central hub for a brewing scientific revolution. Many of the foundational results in the early days of genetics and molecular biology—including Thomas Hunt Morgan’s pioneering work in genetic mapping using fruit flies, Theodosius Dobzhansky’s foundational contributions to evolutionary theory, Linus Pauling’s seminal advances in the nascent field of structural biology, and Max Delbrück’s careful examination of the physical structure of genes—all happened at Caltech.
Like in physics, all of this activity caused many of the era’s great biologists to make a pilgrimage to this highly productive Temple. Jacques Monod, Jim Watson, Seymour Benzer, and Sydney Brenner all either visited Caltech or conducted their primary research there. Even the great resident physicists—including Feynman—spent time dabbling in molecular biology.2
Over time, Kelsic honed his taste in the types of research problems that he was drawn to. He began to hear the echoes of Delbrück. A course in biophysics fascinated him. Unlike high school biology—which consisted of unappealing rote memorization—this field seemed ready to get at the fundamentals of living systems. An additional bioengineering course with Christina Smolke sealed the deal.
Whereas the frontiers of physics had become concerned with extremes—the smallest possible things in the universe, the conditions of black holes, the behavior of matter at the coldest and hottest temperatures—biology was full of fresh problems. The notion that living systems are molecular machines filled Kelsic with excitement.
Attempting to understand—and perhaps one day design—these machines felt worth devoting his life to. In other words, he realized that it is The Century of Biology.
As he prepared to graduate, Kelsic had a choice. He had a job offer as a programmer at a startup. He also had the opportunity to join the Smolke Lab as a technician. Hearing Kelsic wax poetically about the beauty of molecular machines, the startup founder rescinded Kelsic’s offer. At this point, it was clear to the rest of the world that Kelsic was destined to make his impact in biology.
After a year of research, Kelsic headed across the country to start graduate school at Harvard. He was drawn to the Department of Systems Biology, which seemed to have the right balance between experiment and theory. After his first-year research rotations, Kelsic found his home in Roy Kishony’s lab.
Like Kelsic—and the founders of molecular biology before him—Kishony had originally trained as a physicist. He became obsessed with evolution and the problem of antibiotic resistance and decided to hop fields to focus his research efforts on finding a solution. Kelsic was drawn to Kishony’s style of doing “extremely simple experiments but on a really large scale.”
A beautiful example is one of the projects Kelsic contributed to in the lab. The setup was simple. A giant agar dish was prepared with regions of varying concentrations of antibiotics. The concentration was the strongest at the center of the dish. Microbes were plated on the far edges. Over time, they crept closer and closer to the center. With a single elegant experiment, the landscape of evolved resistance to antibiotics was directly visible.3
As his PhD progressed, Kelsic had to design his own thesis project. A few emerging technologies caught his eye. First, the Church Lab at Harvard had developed a technology called Multiplex Automated Genome Engineering (MAGE). While the antibiotic resistance experiment relied on random mutations to occur, MAGE repurposed a bacterial system called Lambda recombination to introduce billions of engineered variants into microbial cells. Critically, the variants were encoded in short stretches of synthetic DNA—called oligonucleotides, or oligos—that can be made at a massive scale in parallel. If the agar plate experiment was Evolution, this was Super Evolution.
Second, the high-throughput DNA sequencing cost decline was well under way. Sequencing was no longer a government mega-project—individual labs were using the new technology in increasingly creative ways. MAGE was powerful, but until then, it was prohibitively expensive to measure which variants were actually being introduced—and which were disappearing or being selected for—at each round of perturbation. Kelsic wondered: would it now be possible to comprehensively sequence a MAGE experiment, providing a glimpse of the underlying evolutionary fitness landscape at each step of the experiment?
The idea for MAGE-seq was in place. Using clever molecular biology, Kelsic devised a way to use MAGE to introduce every possible codon substitution into an essential E. coli gene, and then used high-throughput DNA sequencing to measure the fitness of each variant across the different “pools” of mutants.
In the initial study, Kelsic used MAGE-seq to probe the relationship between RNA structure and codon usage. While it may provide insights for gene design in the future, it’s a fairly academic problem. But buried in the supplement was one of the core insights that would lead to Dyno’s founding.
While this may look like a somewhat confusing heat map to the casual observer, Kelsic could see the underlying principles of biochemistry popping right out at him. This map shows the amino acid substitution at each position in the E. coli gene infA. Below the map, a PCA analysis showed that only a few principal components were necessary to explain much of the variance in the data. On closer examination, each principal component seemed to correlate directly with an underlying biochemical rule. For example, PC1 was most strongly associated with residues that were buried inside the three-dimensional structure of the encoded protein.
Put more simply, a small number of biochemical rules were highly predictive of which amino acids could be tolerated at each position in the gene.
Kelsic realized he was on to something. This experiment was an example of a multiplexed library. Each variant in the pool could be identified and tracked using DNA sequencing.
Despite the technical complexity under the hood, the experiment itself was simple. Swap every codon with every other possible codon. See what happens. Like with the agar dish, deep principles fell out of a simple experiment. Both studies used a simple idea at a massive scale to offer a glimpse of the evolutionary landscape Darwin could only theorize about.
It was clear to Kelsic that MAGE-Seq—and other technologies like it—could have a massive impact on the field of protein engineering. One day, we might be able to understand what a protein does just by looking at its 3D structure on a computer. But that day is far away. In the meantime, we have tools to test potentially billions of designs empirically using the awesome power of genomics.
Multiplexed libraries offer a data-driven way to explore and engineer complex protein systems.
As 2015 began, Kelsic tucked this idea in the back of his mind and prepared to defend his PhD. When thinking about his next steps, the allure of startups tugged at him. He and a few friends had earned runner-up at the Harvard President’s Challenge for Social Entrepreneurship. While their genome browser startup fizzled out, the experience had been a highlight of grad school. As the former captain of his college soccer team, Kelsic relished being a part of a tight-knit group working towards a common goal.
With this interest in mind, Kelsic reached out to George Church. Between his Darwinesque beard, his lanky 6-foot-5 frame, and his lab’s seemingly endless stream of inventions, Church is a towering figure in the world of biotech.4 One of the many quirks of the Church Lab compared to its peers is that it also tends to churn out more young biotech CEOs than academic professors.
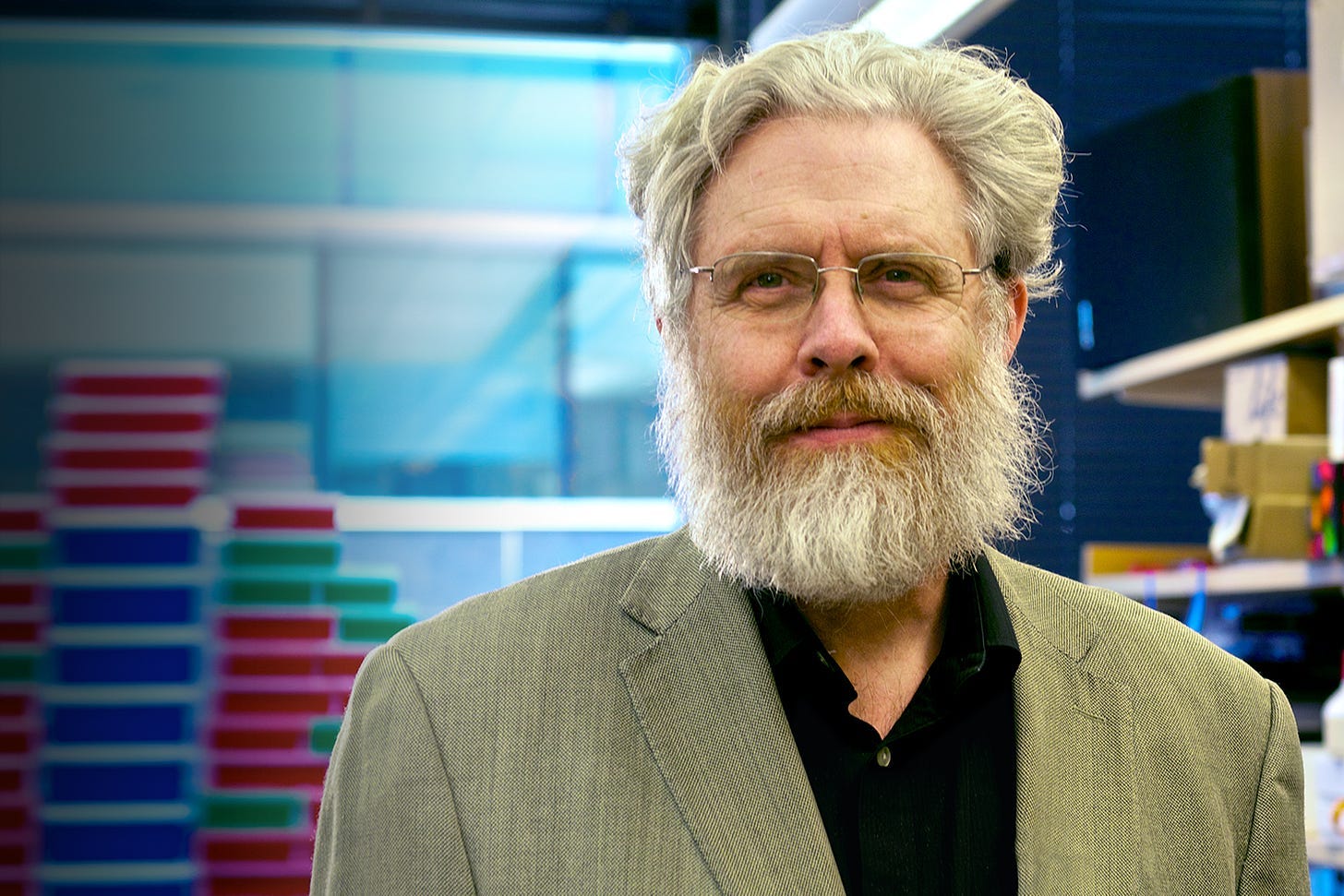
MAGE was one of the Church Lab’s many inventions, and Kelsic wanted to learn enough about this type of large-scale DNA synthesis to incorporate it into his growing toolbox for multiplexed experiments.
Kelsic pitched Church on a plan: he would come to do a short six-month postdoc, share his experience in analyzing multiplexed experiments, and pick up some DNA synthesis experience. After gaining more industry exposure, he would join a startup. Church was okay with this idea but told Kelsic he should still go through the normal postdoc interview process and meet members of his group.
During this process, a few things clicked into place. Kelsic got first-hand exposure to the ambitious postdocs spinning out companies. Ambition is contagious. As Paul Graham puts it: “Probably most ambitious people are starved for the sort of encouragement they'd get from ambitious peers, whatever their age.” He also got exposed to the problems that Church Lab members working on gene therapy were facing.
Scientifically, in vivo gene delivery seemed to be a beautiful applied problem for multiplexing. Again, the predominant method of gene delivery is to harness viral capsids, and out of all viruses, the adeno-associated virus (AAV) is one of the most widely used.5
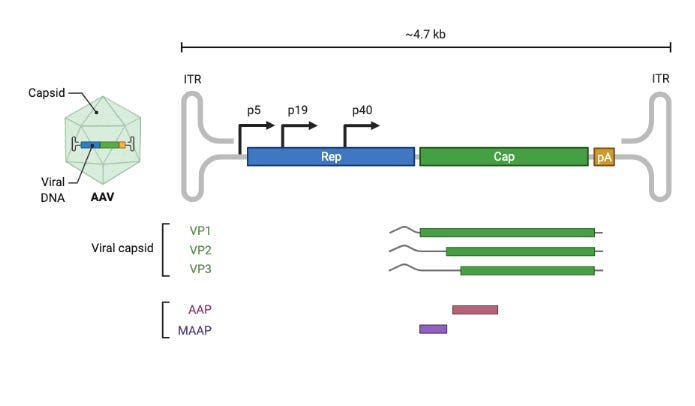
There are several reasons why AAV is widely used. It is a fairly benign virus—unlike SARS-CoV-2, for example—and is able to infect both dividing and non-dividing cells. Importantly, its capsid shell is a compact icosahedral structure encoded by a small set of proteins: VP1, VP2, and VP3.
Still, there are looming problems for AAV gene delivery. Even for a small capsid and genome, there’s a lot we still don’t know about AAV biology. This makes it hard to predictably engineer new AAV capsids that target specific cells in the body and can be readily manufactured. It’s especially hard to optimize for both properties simultaneously.
Much like the essential E. coli gene that Kelsic had previously mutated with MAGE-seq, he realized it should be possible to generate rich data describing the underlying fitness landscape of AAV. This landscape could potentially be used to design a large number of highly specific new vectors with many improved properties.
Around the time Kelsic intended to end his postdoc, he recalls coming to a crucial realization: data-driven AAV design felt like a once-in-a-lifetime idea. If it was, he wasn’t going to join a biotech startup—this could be the basis of an entirely new startup.
Several factors made this idea particularly compelling:
Until very recently, this wouldn’t have been possible. Multiplexed libraries are a byproduct of the Carlson Curves—both DNA sequencing and DNA synthesis have gotten much cheaper over the past 20 years. Without both inputs, the type of large-scale experimental AAV screening Kelsic was contemplating would have been a non-starter. The Why Now? for this opportunity couldn’t be more clear.
AAV engineering would require complex multi-objective optimization. It would be hard to imagine another solution to this extremely challenging problem without these new technologies.
On the business side, Kelsic wanted to gain more conviction that this was actually a problem worth solving. If he designed new highly potent AAV vectors, would drug makers actually want to use them?
Kelsic started by meeting with Church Lab alumni working on gene therapies in industry and gradually spoke with more and more companies. Each company he spoke with conveyed a strong interest in better AAV vectors. In parallel, he took courses at Harvard Business School on commercializing science and learned about making business decisions using the Case Method.6
This is one of Kelsic’s main points of advice for aspiring founders: make something people want. It’s not enough to have fancy new technology. Value is created by solving real problems that people need solutions to. You know you’ve found a good problem when people are excited about your solution regardless of how you found it.
Kelsic realized that he had stumbled on the ultimate puzzle. Like the world of Karakuri boxes from his childhood, AAV capsid design is a challenging and open-ended three-dimensional puzzle. But if he could make a dent in solving this puzzle, it could potentially transform the lives of countless patients around the world.
But what would a solution to this problem entail?
Machine-guided design
After many fruitful years as an inventor himself, George Church now serves as a hyper-connector for the next generation of molecular wizards. As Kelsic refined his project ideas, Church put him in touch with his talented graduate student Pierce Ogden, who was also obsessed with using multiplexed libraries for therapeutic applications.
Another critical connection via Church was with Sam Sinai. Originally studying to be a physician in his native Iran, his life changed when his family's green card application was approved after 13 years. While his original goal was a career in medicine, Sinai harbored dreams of pursuing fundamental research. After gaining acceptance to MIT, he moved across the world to begin his studies anew.
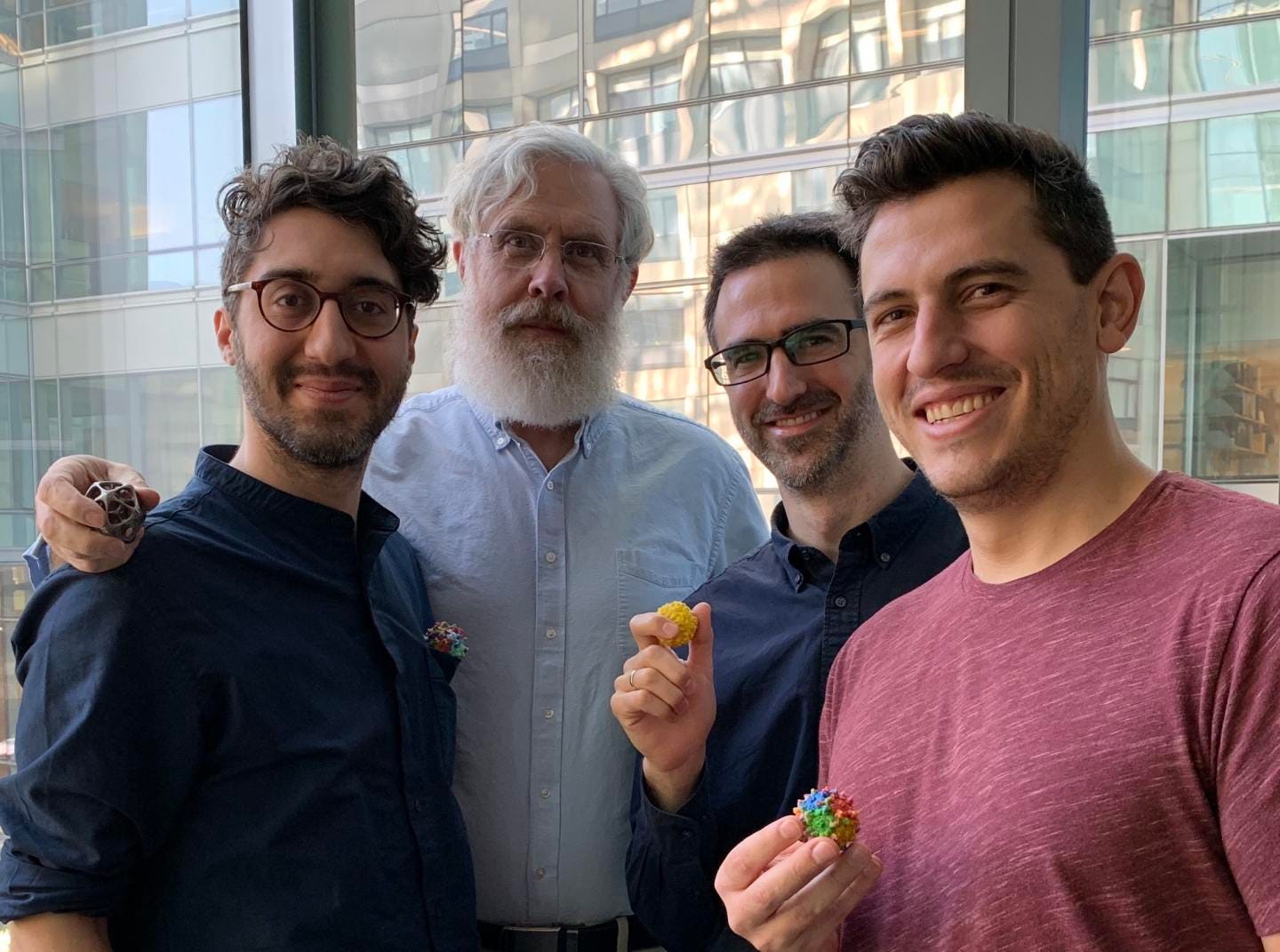
In Iran, Sinai had entered medical training directly after high school—receiving little exposure to other scientific disciplines. At MIT, Sinai worked his way through the heavily quantitative first-year general curriculum. Here, he fell in love with math, physics, and computer science.
While the competitive and rote nature of American pre-medical education grated against him, quantitative research proved to be a better fit for his deeply inquisitive and philosophical nature. While majoring in computer science, Sinai did multiple research projects at the interface of AI, neuroscience, computation, and biology. In his graduate studies at Harvard, he embarked on a quantitative research program studying the origins of life.
At a small conference on this topic, Sinai crossed paths with George Church. By this point, Sinai had begun to form a distinct quantitative view of Evolution. To Sinai, “Evolution is a search algorithm,” and he felt that the “structure of its solution space” held the key to understanding the origins of life. He asked Church if his lab was pursuing any high-throughput screens of RNAs or viruses—as the simplest molecular systems capable of self-replication. Church quickly connected him with Kelsic.
Starting in early 2016, this small group pursued two parallel research tracks. Ogden and Kelsic pushed hard to create multiplexed AAV libraries in the lab. Simultaneously, Kelsic and Sinai explored ideas for using machine learning algorithms to computationally generate new capsid designs to test.
Pieces of this work started to come together. In 2017, they released a short workshop paper at Neural Information Processing Systems detailing their early results. This work demonstrated an early use of variational autoencoders (VAEs)—a type of generative model that sparked some of the excitement around what we now call “generative AI”—for predicting the impact of mutations in proteins.
At the bench, Ogden and Kelsic achieved a key breakthrough. They had devised a way to use DNA barcodes to keep track of each mutation they introduced into the AAV capsid sequence. Achieving this at scale required a clever solution. Each mutation would be synthesized in an oligo containing a unique DNA sequence that could serve as a molecular identifier during sequencing. However, after the initial synthesis, the variant and the barcode would be separated from each other in the final plasmid construct.
DNA barcoding is an underrated innovation. Think about it: DNA stores information. If you can print DNA cheaply, why not use it as an experimental bookkeeper to tag the molecules you’re interested in keeping track of? In this way, it’s possible to introduce a massive number of unique mutations and to track their distinct consequences in a single DNA sequencing run. Instead of building a giant robotic laboratory, millions—or even billions—of experiments can be run in parallel at the molecular level.
Using their multiplexing strategy with DNA barcodes, they created the first map of every single codon substitution, insertion, and deletion of AAV2, one of the variants of AAV that is already used for approved gene therapies.
Around this time, Kelsic evaluated whether it was the right time to start the company. Motivated by his business school studies and additional reading, he deployed a fairly widespread model for assessing risk, asking three questions:
Is there market risk? Through his customer discovery with gene therapy developers, Kelsic knew he was solving a real problem. If a company could make greatly improved AAVs, people would want them.
Is there technical risk? In the academic setting, Kelsic and his colleagues had demonstrated an ability to test enormous numbers of AAV variants, and shown optimized delivery in mice. He knew drug makers would want to see data in nonhuman primates but felt comfortable about the risk associated with making this leap in a company setting.
Is there team risk? Well, there wasn’t a team in place quite yet.
Kelsic started to round up a tight-knit team of co-conspirators to incorporate a company. He reached out to Adrian Veres, who was a talented undergraduate researcher in the Kishony Lab while Kelsic was working on his PhD. With a shared background in physics, they had quickly hit it off in the lab—and they both saw the beauty and potential of pooling screening experiments. Kelsic also knew he was a good teammate after collaborating with him during the President’s Challenge.
When Kelsic reached back out, Veres was working to complete the rigorous MD/PhD program at Harvard. The proposition of being able to marry his expertise in pool screening with his commitment to advancing medicine hooked him.
Veres remembers Kelsic reaching out to him and saying, “Adrian, I think I’ve found the ideal application for multiplexing.”
Not all leaders are particularly generous with credit, but Kelsic is. Even though he’d now spent four years as a postdoc meticulously researching this market opportunity and refining a complex technology in the lab, he was receptive to anybody who could make a big difference for the company joining him as a co-founder. Beyond Veres, Sinai wrapped up his PhD and accepted a co-founder role as well. Church joined as a scientific co-founder, along with Tomas Björklund, a Swedish professor whose AAV prowess made Kelsic strongly prefer him as a collaborator rather than a potential competitor.7
The fundraising process accelerated when Kelsic decided that he would serve as CEO, giving investors a clear understanding of who they would be supporting. Polaris Partners, a storied Boston biotech venture firm, led the Seed round along with CRV, another leading firm that was originally founded in 1970 to help commercialize research from MIT. KdT Ventures, an up-and-coming biotech firm founded by physician-scientist Cain McClary, also participated in the round.
At this point, Alan Crane, a biotech veteran and Entrepreneur Partner at Polaris Partners, joined as co-founder to help actively guide the company. Dylan Morris from CRV joined the board of directors. With this structure in place, Dyno’s leadership was now a blend of brilliant young first-time technical founders, leading scientific co-founders, and more experienced biotech operators. According to PitchBook data, the Seed round was a $9M raise at a $20M pre-money valuation.
In 2019, a year after incorporation, the Harvard team’s massive AAV2 study landed in the pages of Science Magazine. The study laid out the basic structure of a multiplexed library for AAV engineering.
Let’s consider how this works. Imagine you want to ask a simple question: how does a variant impact the amount of capsid that is produced? You’ve got a pool of synthetic DNA, where each variant is associated with a unique barcode.8 Before making a capsid, the DNA needs to be transformed into circular pieces of DNA called plasmids. You can calculate the fraction of plasmid barcodes and capsid barcodes. Finally, take the ratio of this frequency and that of the AAV with no mutations—the wild-type (WT) virus. This metric tells you if a mutant is being selected for.
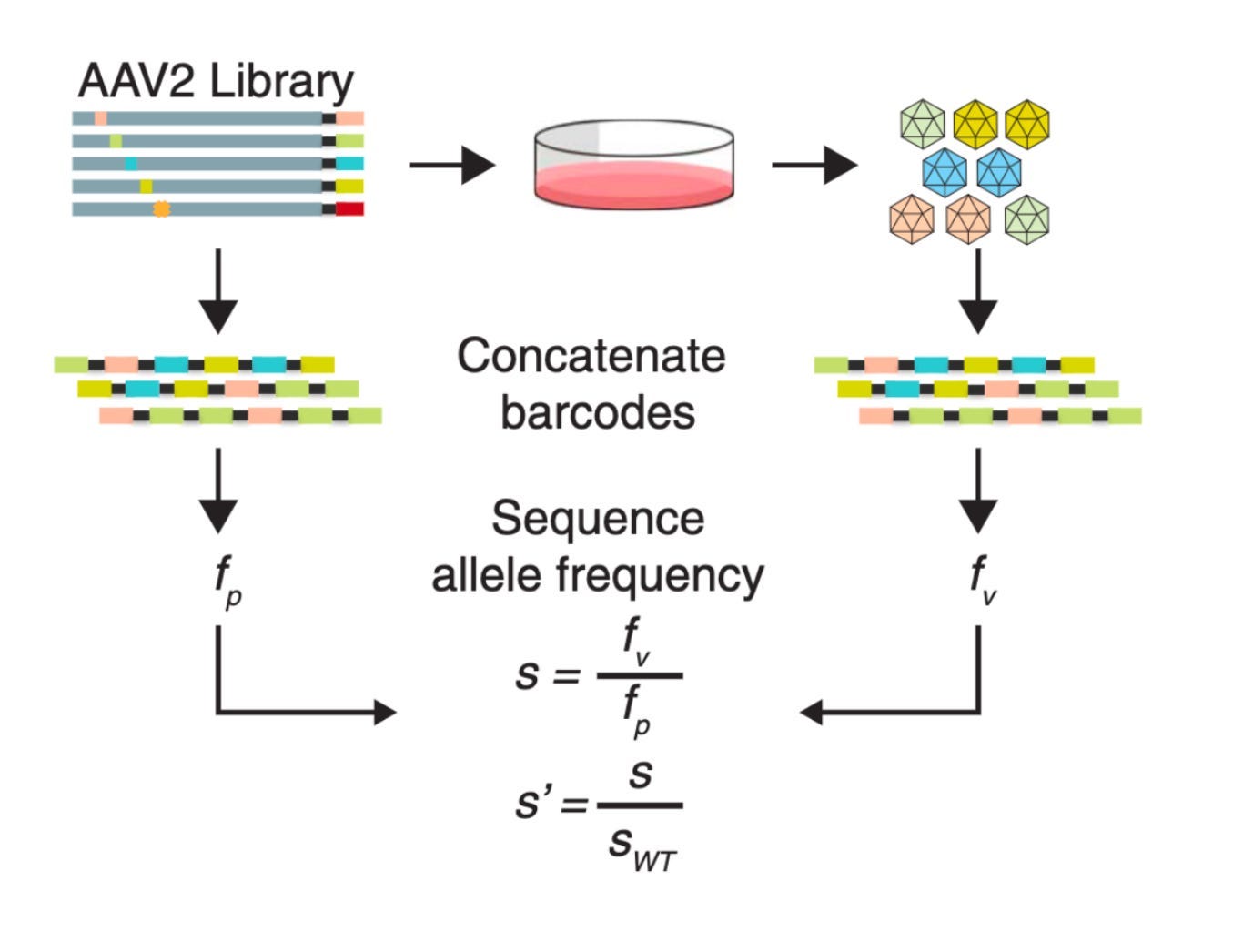
Much like the heat map we saw earlier, this rich data provides a glimpse of the underlying fitness landscape for the virus. Immediately, a few observations pop out from the data. Most mutations negatively impacted fitness—as shown in blue. Amidst a sea of blue, a smaller handful of variants positively impacted production. Without this detailed empirical map, it would be incredibly hard to guess a priori exactly which mutation would be blue and which would be red.
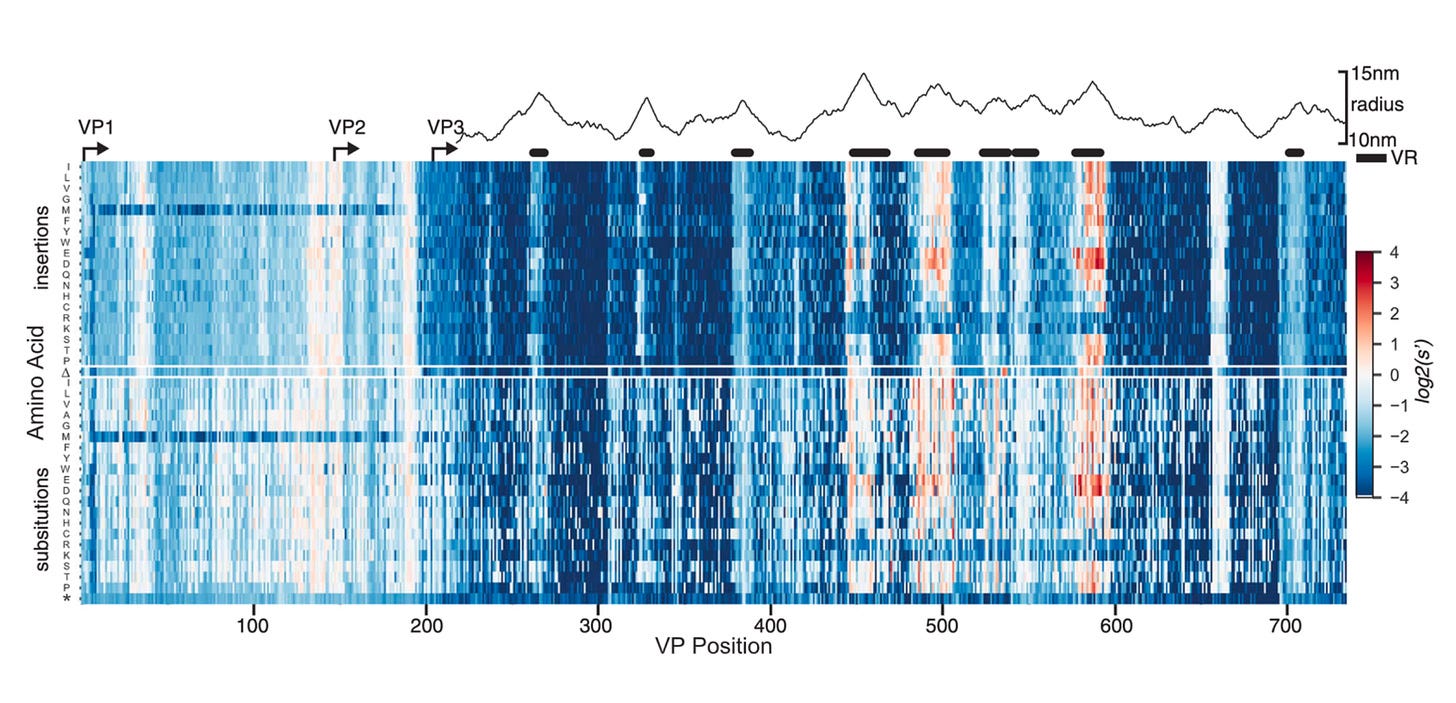
The squiggly mountainous graph above the heat map shows the distance from the radius of the viral capsid. Some direct rules seem to pop out. Variable regions (VR) farther away from the radius inside the VP3 reading frame seem to tolerate mutations the best.9
Again, using the power of multiplexed libraries, biological rules are dropping out of the data.
A lot happened in this first major study. An entirely new AAV protein was discovered, and the multiplexing strategy was adapted for in vivo experiments in mice to track where different viral variants ended up in the body. But perhaps the most important result of the study was an empirical demonstration of Kelsic’s central hunch. This data can be used to computationally predict the fitness of variants, unlocking a design paradigm that is far more effective than testing random variants by brute force.
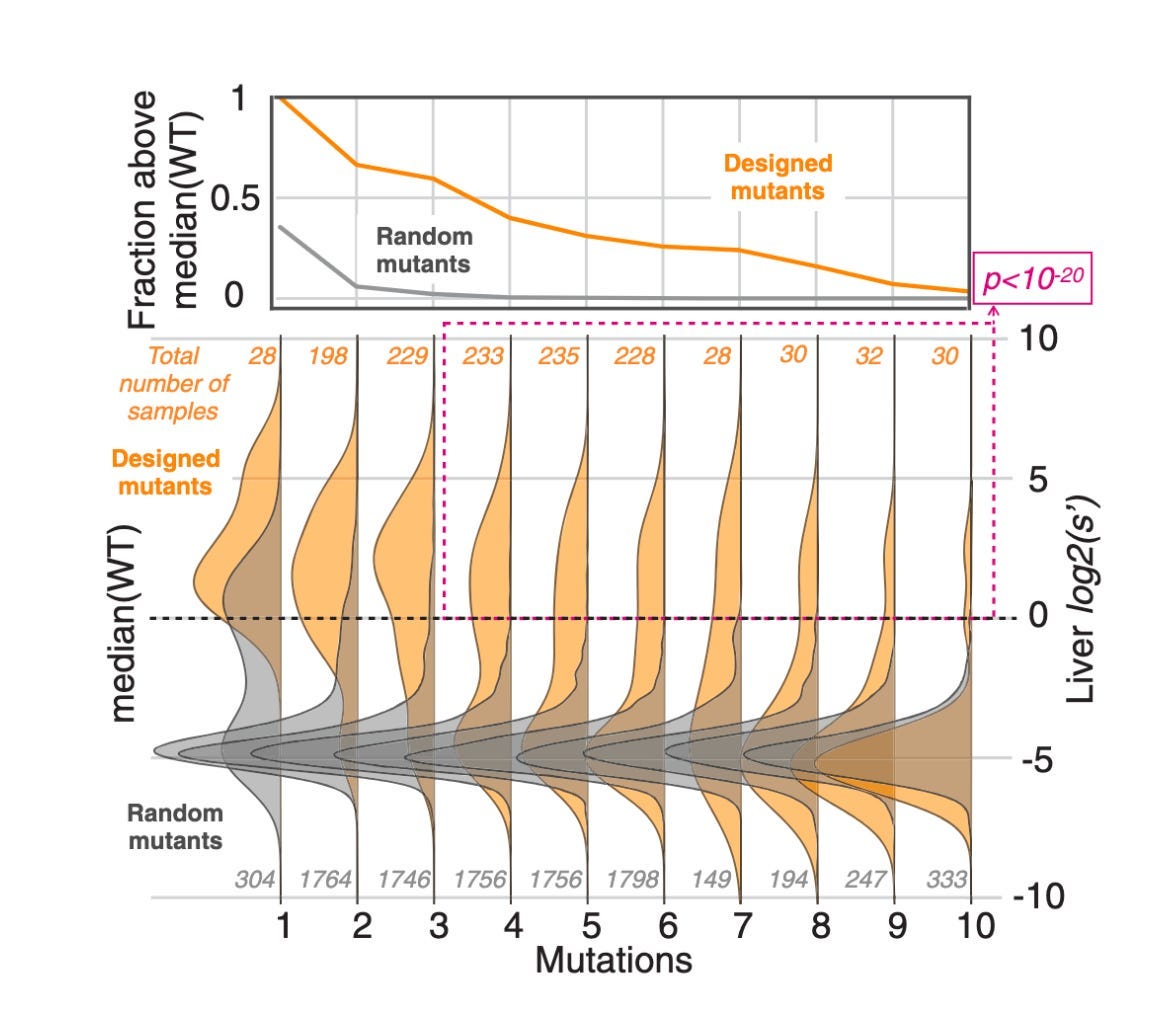
Above, you can see an experiment testing AAV variants with an increasing number of mutations. While the likelihood of finding an enriched random mutant with more than two or three variants was basically zero, a simple statistical model of the data was capable of designing capsids with up to ten mutations.
In nature, there are hundreds of mutations between AAV serotypes. For any design strategy to produce truly novel capsids in the AAV fitness landscape, it would need to be capable of introducing far more than a handful of mutants. These early data points were highly encouraging.
In an accompanying report in Cell & Gene Therapy Insights in the same year, Kelsic and Church wrote that “machine-guided workflows suggest the possibility of Super Darwinian Evolution (SDE), which we define as any exploration strategy that beats a Darwinian approach of random mutagenesis and selection on a given fitness landscape.”
Darwinian Evolution is responsible for creating every life form on Earth. All of the microbes and multicellular creatures and the intricate webs of connection between them. The flowers, and the bees that pollinate them. The generations of carbon-based life forms that coalesced into viscous oil, and the descendants of apes that now harvest this oil from deep below the ground for energy.
Now, we can synthesize DNA and sequence it. We can use computers to analyze this data. And we can navigate the fitness landscape, designing new biological sequences more efficiently than Evolution could.
But from here, a key challenge remained. Looking for beneficial variants—especially in combination—is a herculean search problem.10 Kelsic and Church wrote that “it is still unknown what algorithms for SDE will be best suited for AAV and for other proteins as the complex structure of protein fitness landscapes is not well understood.”
In 2021, the team published a more definitive answer to this question in Nature Biotechnology. Through a long-standing collaboration with Google Research, they learned that richer machine learning models could generate better AAV designs. With more sophisticated models, a “closed-loop” platform for capsid design was in place. The parallel research tracks of multiplexed libraries and machine learning had converged into a single integrated system—or, as Church would say, “ML squared.”
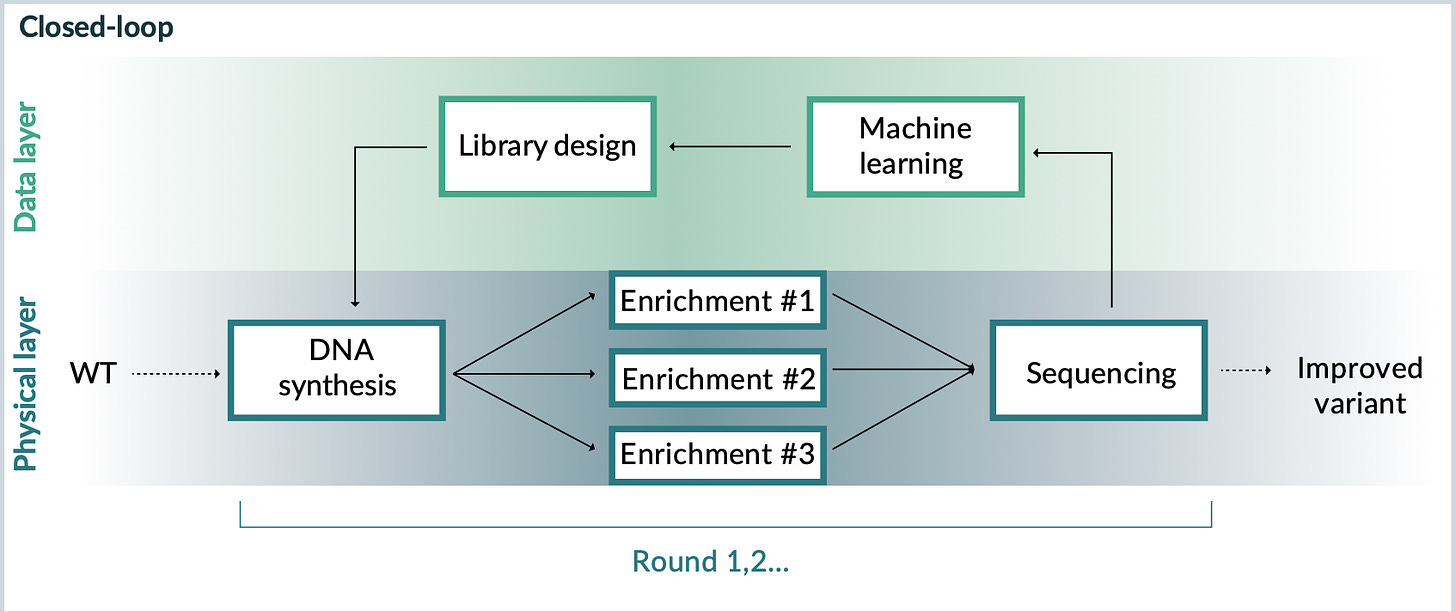
From these publications, it’s possible to reason about what’s happening behind the scenes at Dyno. With more resources, Dyno is able to iterate through this workflow at an industrial scale. They can move from millions of designs to billions of designs. Each step in the process can be refined and improved.
Running a platform at this scale can lead to “an embarrassment of riches,” as biotech veteran Steve Holtzman would say. In theory, Dyno could develop far more capsid designs than they could possibly clinically test on their own.
Beyond rethinking virus design, Dyno decided to take a first-principles approach to the design of their company strategy. They needed a way to get as many of these improved capsids into the clinic as possible—and to be rewarded for it.
Capsids-as-a-Service
In rock climbing, sometimes a large dynamic movement—or dyno—is required to reach a new hold. This type of leaping transition is considered one of the most difficult moves in climbing. Like its namesake, Dyno Therapeutics aims to make challenging leaps across the AAV fitness landscape in search of new peaks of performance.
From the viewpoint of a therapeutics investor, Dyno’s commercialization strategy would be obvious. All value in biopharma stems from making clinical assets. As a platform, Dyno could initially partner with Big Pharma companies to piggyback on their resources and deep clinical development chops. Dyno would make a leap and find a new peak with a lead program, and the pharma company would commercialize it. This type of deal would likely favor their pharma partners, but it would be a meaningful stepping stone on the way toward an internal drug pipeline.
That’s just the way things work.
Kelsic felt differently. As he built the foundation for Dyno in the Church Lab, he saw several gene therapies rise and fall based on clinical failure. If Dyno took the same path and decided to be a full-stack gene therapy company, their future would likely hinge on a single clinical readout. To get to that readout, they’d need to finance a trial with highly dilutive VC dollars.
All of this seemed detrimental to the type of company that Kelsic ultimately wanted to build. The whole point of Dyno’s platform is that it should enable a huge number of leaps to new peaks in the fitness landscape. And importantly, the platform should continually improve with each capsid design project. It’s not about a single gene therapy—it’s about unlocking the potential of gene therapy as a therapeutic modality.
Recently, a video of NVIDIA CEO Jensen Huang talking about exactly this type of progress in biology went viral. He said, “For the very first time in human history, biology has the opportunity to be engineering, not science. When something becomes engineering, and not science, it becomes less sporadic, and exponentially improving. It can compound on the benefits of previous years.”
What does this type of compounding progress look like in practice? Think about Dyno’s key inputs. DNA sequencing costs are continuing to fall. The same is true for DNA synthesis. The same is true for compute. Dyno gets to ride these external tailwinds. Each new capsid design project should be cheaper and should produce data that accelerates the next project. This is what compounding looks like.
In order to get as many cycles around this flywheel as possible, Dyno has attempted to externalize clinical risk. Their goal is to have a laser focus on capsid design and to partner with as many drug makers as possible. Much like a rising tide lifts all boats, Dyno aims to push the entire field of in vivo gene therapy forward with better clinical results. This happens in two ways:
Capsid engineering research collaborations. Dyno partners with gene therapy companies to develop new capsids for their programs. Here, it’s all about starting with requirements for a specific program and then working backward to design a capsid to meet those requirements. Revenue comes in the form of upfront payments, followed by milestone payments and royalty revenue from program success.
Ready-made capsid licensing. Separately, Dyno uses its platform to develop capsids for use across a wide range of disease areas and indications independently. The idea is for companies to license these capsids as an off-the-shelf product for new programs. With their growing expertise in delivery, these capsids may ultimately offer solutions to problems that drug makers don’t even realize they have yet.
At no point does Dyno intend to develop wholly-owned gene therapies. It’s an “Intel inside” model, as Steve Holtzman would put it. Like the chip manufacturer whose revenue flows from powering countless devices in the market, Dyno aims for its capsids to be used for every AAV gene therapy product in the clinic.
Historically, this has been a challenging business model in biotech.
Consider therapeutic antibodies—arguably the greatest class of bioproduct of all time.
These large Y-shaped proteins excreted by B cells in the blood can specifically bind to regions of a protein surface called antigens. The first companies to successfully harness antibodies to bind to therapeutic targets—such as Genentech, Amgen, and Regeneron—have become massive generational companies.
As the technology became commoditized, more partnership-centric antibody companies such as Genmab and Adimab—and their more recent counterparts such as AbCellera and Absci—have emerged. All of these companies are an order of magnitude smaller than the first companies that pursued a path toward becoming vertically integrated pharmaceutical companies that market wholly-owned antibody drugs.
The reason for this is straightforward. In the world of therapeutics, all value comes from clinical assets. Public market investors value biopharma companies based on the discounted future cash flows of their drug products and little else. Owning the majority—or entirety—of a blockbuster drug generating over $1B in annual revenue is what gets rewarded. Partnership-centric companies receive a much smaller slice of the revenue streams and get valued for much less as a result.
There are a few compelling reasons to think that the story might be different for Dyno.
To start, the therapeutic payload and delivery vector for a gene therapy product are uniquely orthogonal. The gene being delivered and the capsid it rides in are separate design problems. In the most extreme case, as Veres pointed out to me, “You could imagine a Dyno vector demonstrating successful delivery in a clinical trial that ultimately fails because of the payload.”
Because the vectors are such a distinct component of each product, it’s possible to work on multiple therapeutic programs in the same area. Importantly, the deal structure of Dyno’s early partnerships directly enables this. For example, even though Dyno has already partnered with Roche for delivery to the Central Nervous System (CNS), they are still able to work with additional partners for any CNS indication. If Dyno produces sufficiently valuable off-the-shelf capsids, it’s very possible that each product could generate multiple revenue streams.
Over time, all of these revenue streams would start to add up. The central thesis for Dyno is that this strategy could grow to rival—or even surpass—the value of a more consolidated and wholly-owned gene therapy pipeline. Imagine a world where hundreds or even thousands of AAV gene therapies enter the clinic, each using a Dyno capsid. The potential is enormous.
Two major market forces threaten to thwart Dyno’s growth. First, drug makers could prove to be reluctant to part with any of their future cash flows. They could work to develop their own in-house infrastructure for improved capsid design. Second, if Dyno continues to successfully land valuable partnerships, this market signal could spur a dozen fast-followers to enter the fray.
Kelsic is confident that Dyno has focused on a sufficiently challenging problem. Capsid design is hard enough that pharma companies haven’t been able to crack it on their own so far. With the compounding data and modeling advantages of their platform over time, any competitor would have to contend with Dyno’s daunting Scale Economies.
Additionally, the product space for AAV design is realistically finite. There is likely a discrete set of distinct capsids with the properties a viable therapy would require. According to Kelsic, “Most of the delivery challenges in gene therapy could be solved with less than 100 engineered capsids.” With their platform up and running, Dyno aims to leap across the AAV fitness landscape faster than anybody else, generating an IP moat that would require any partner to pony up if they want the best possible capsid.
So far, this strategy seems to be working. Out of the gate, Dyno landed partnerships with Novartis for eye delivery, Sarepta for muscle delivery, and Roche and Spark for CNS and liver delivery. This traction helped them ink their $100M Series A round led by a16z at a $500M post-money valuation, according to PitchBook data. Since then, Dyno has already landed a new partnership with Astellas for another set of muscle delivery projects.
More recently, Dyno has started to roll out its own off-the-shelf products.
Each year, thousands of researchers and entrepreneurs convene from around the world for the American Society of Gene and Cell Therapy (ASGCT) Annual Meeting. The meeting provides a snapshot of progress from the top labs and companies in the field. In May of 2023, the Dyno team traveled to Los Angeles to share some of their latest results at the conference.
Towards the end of the conference, Kelsic presented on the main stage. In standard fashion, he warmed up the crowd with an overview of Dyno’s platform. From here, most companies share some new data on their internal programs. Instead, Kelsic transitioned into a full-fledged product launch.
Dyno was ready to take the wraps off of their first set of licensable capsids. And out of these capsids, Dyno bCap1 was the major story. Introducing their new product nomenclature, this was the first version (1) of Dyno’s capsid (Cap) for brain delivery (b). Right out of the gate, the data indicated that bCap1 had the potential to be the field-leading capsid for CNS delivery.
Compared to the AAV9 serotype, bCap1 has 1x production efficiency, 10x less off-target liver delivery, and a 100x improvement in brain transduction. Critically, as a private company, Dyno had successfully made the transition from their initial academic results in mice into non-human primates—the gold standard model for preclinical development.11
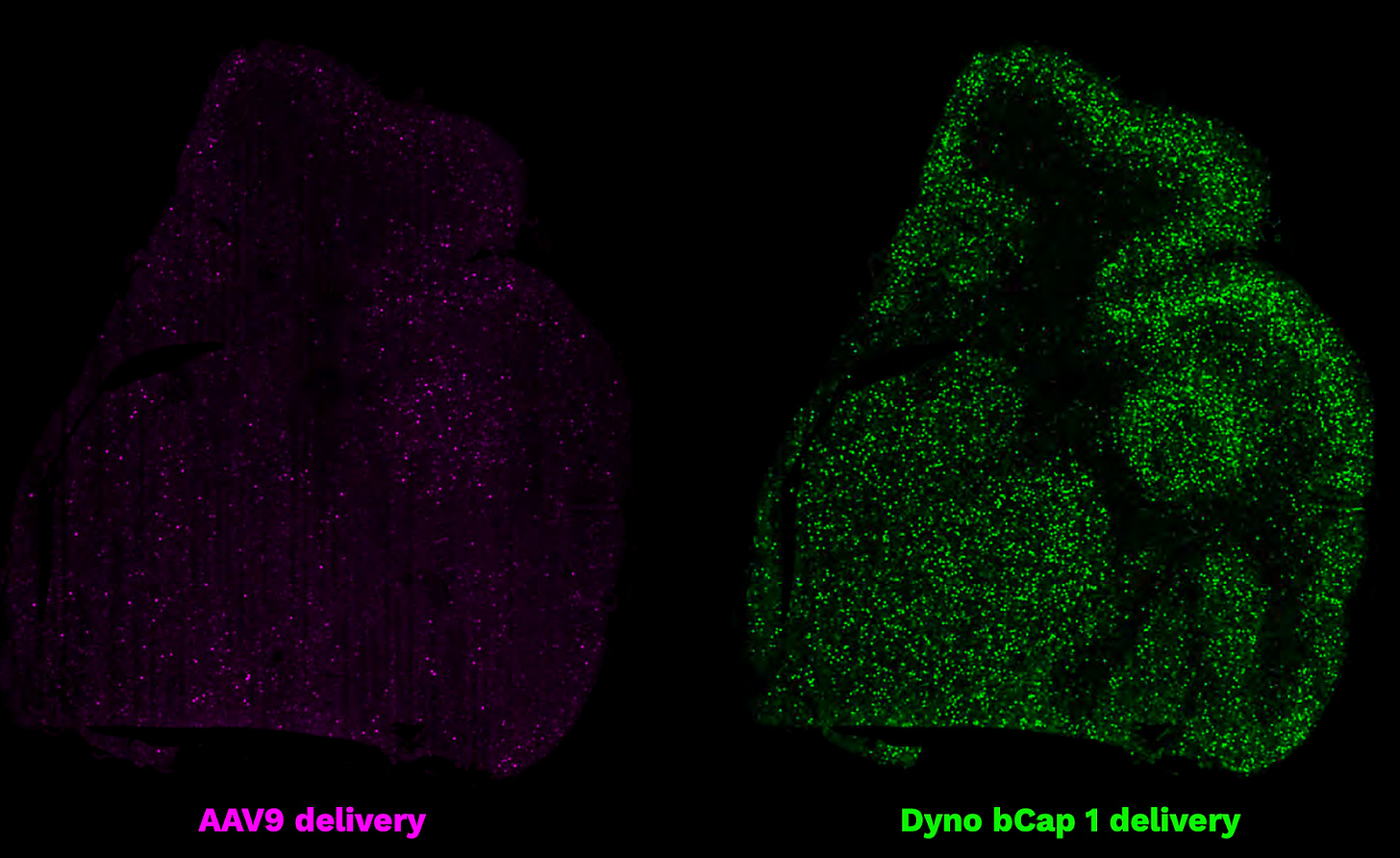
Later in 2023, Kelsic took the stage again at ESGCT in Brussels—the European equivalent to ASGCT. It was time for another product launch. Dyno unveiled eCap1—another capsid with field-leading potential, this time for the eye. Relative to the AAV2 serotype, eCap1 has 1x production efficiency and an 80x increase in retina transduction. Dyno also offered a detailed molecular dissection—cell type by cell type—of how eCap1 performs in different distinct therapeutic contexts.
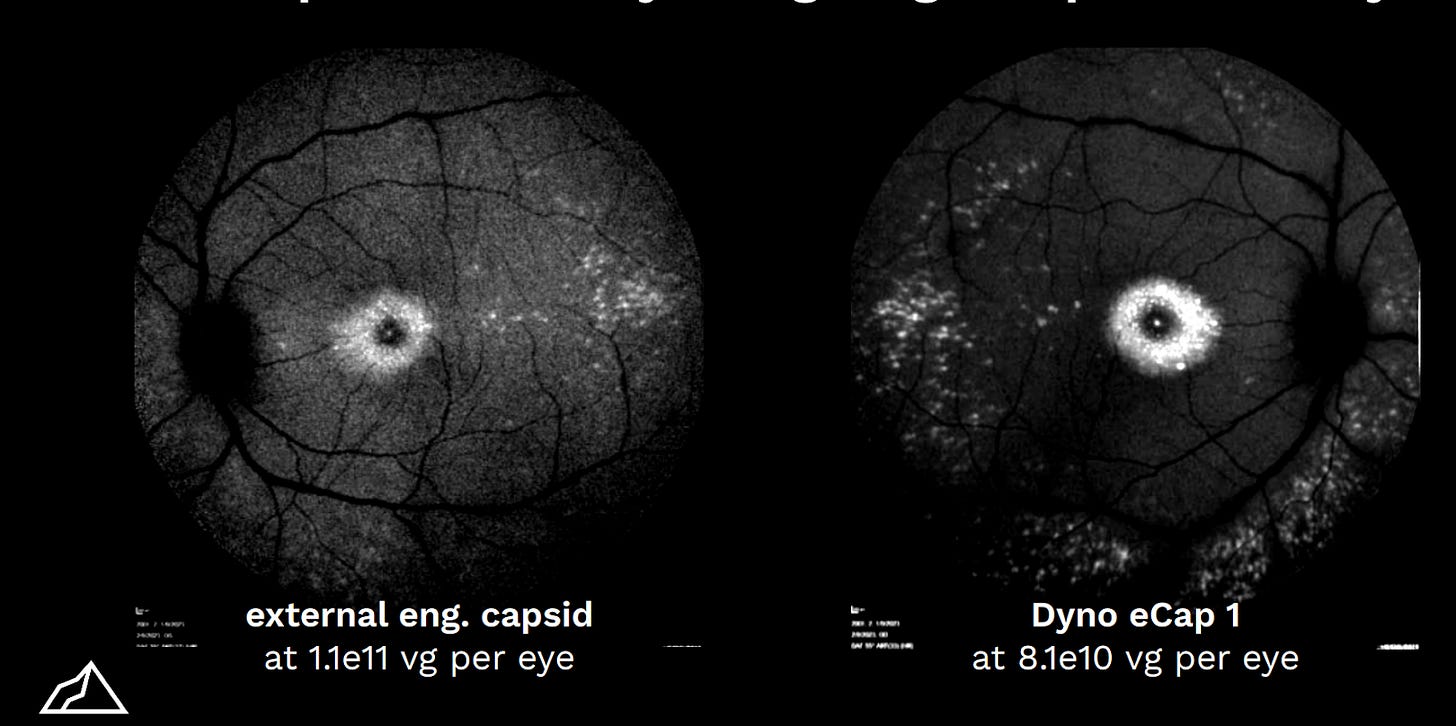
Despite their horizontal business strategy, Dyno is very much a product company. The company’s website now includes a product splash page, highlighting data for bCap1 and eCap1 to entice prospective partners. Their ideal would be for licensing to be as easy as filling out an application form—where each partner can benefit from their capsids by agreeing to standard terms.
Much like a tech company, Kelsic has worked to instill a culture of continuous improvement and product iteration—as the version numbers in the product names suggest. In order to make the best possible capsids, the platform engineers can’t rest on their laurels. AAV libraries are still being synthesized, DNA sequencers are still humming, and code is still being shipped.12
After college, I returned to my hometown of Seattle. There, I landed a job as an engineer/scientist in the Department of Genome Sciences at the University of Washington. As a discipline that blends biology and computer science, the department was an affiliate of the eScience Institute.
The central thesis of the eScience Institute was to “advance data-intensive discovery in all fields.” Beyond genome scientists, members included astronomists, atmospheric scientists, and earth scientists. In the 21st century, computers are reshaping the scientific method. As visionary scientist Jim Gray would put it, we’re in the “Fourth Paradigm” of the scientific revolution. First, we moved from pure empiricism to theory. Now, we’ve moved to computational and data-driven approaches to discovery.
While all fields benefit, I think that biology is the poster child. Why? It’s stating the obvious, but biology itself is literally digital in nature. Rather than a binary code, cells run on a quaternary code. That is not an analogy. As our instrumentation has improved, we’ve learned how to read that code and how to write it.
While other fields also have better instruments, bigger data sets, and more complex statistical tools than they used to, the feedback loop in biology is different. At Dyno, digital designs of AAV sequences are physically synthesized—bits to atoms. Each design is tracked using information stored in DNA barcodes—atoms to bits. Wash, rinse, repeat.
This type of vision comes from a new phenotype of biologist. Over the course of the 20th century, physicists flooded into the life sciences and jump-started the discipline of molecular biology. Now, their intellectual descendants—like Kelsic, Sinai, and Veres—are mixing and matching ideas across physics, math, computer science, and biology in entirely new ways.
Founders like Kelsic are also historical firsts in a different way. They are the first cohort of biotech founders who stewed over Paul Graham’s essays and watched people around them achieve startup success at the likes of Facebook and Instagram. They’ve seen what type of scale young technical founders can achieve.
The ambition is to make a true dent in the world of biotech—to go from Zero to One.
To do that, Dyno has a long way to go. The designs from their platform need to make their way into humans, and the humans need to be better off because of it. Value needs to be continually demonstrated to their partners. Their business model needs to show signs of scalable growth to their investors. The platform needs to keep cranking.
Balancing all of this is the ultimate puzzle, and Kelsic and the Dyno team are doing the best they can to solve it. If they do, gene therapy will never look the same.
Thanks for reading this essay on Dyno’s early efforts to revolutionize gene therapy.
If you don’t want to miss upcoming essays, you should consider subscribing for free to have them delivered to your inbox:
Until next time! 🧬
The other products are either cell-based therapies or ex vivo gene therapies that edit genes in cells that are isolated from a patient before being administered. An example of this is Casgevy, which made history as the first-ever FDA-approved CRISPR therapy on December 8th, 2023. The therapy works by editing a patient’s blood stem cells outside of their body.
While many of the ideas explored by these Caltech scientists have stood the test of time, others haven’t. Caltech recently removed Millikan’s name from several buildings on campus because of his prominent role in the American eugenics movement.
It’s worth noting that this video has gone viral (no pun intended), having now been viewed over 2.5M times. The leader author, Michael Baym, is now a professor at Harvard. Tami Lieberman is now a professor at MIT. This video and the full publication are used as teaching materials on microbial evolution in college classrooms around the world—I distinctly remember watching this video in my undergraduate microbiology course.
For those unfamiliar with George Church and his antics, consider reading this PNAS profile or Popular Science article, or watching him live on the Colbert Report.
The three most commonly used viral vectors for gene therapy are lentiviruses, adenoviruses, and AAVs. For a detailed delineation of their properties and different advantages, consider reading Viral vector platforms within the gene therapy landscape. AAVs hold tremendous promise as the most compact and least immunogenic vector.
A quick meta-point here: if you’re an aspiring biotech founder reading this, you’re ahead of the game. One of the best ways to learn about business is to study specific businesses in detail. Much like the Case Method, studying how founders start and run scientific companies should help strengthen your own ideas and thinking.
Pierce Ogden didn’t join Dyno as a co-founder. Instead, he helped launch Manifold Bio, where he currently serves as CSO. In fact, there was a third company that emerged from the same lab bay in the Church Lab: Nabla Bio.
Actually, each mutant has either two or four DNA barcodes, but for simplicity, thinking about a 1:1 mutant:barcode mapping is just fine.
There are three isoforms of the viral cap protein: VP1, VP2, and VP3. They form the final capsid in a 1:1:10 ratio.
For a much more comprehensive treatment of the computational difficulty of this problem, check out A primer on model-guided exploration of fitness landscapes for biological sequence design by Sinai and Kelsic.
The beauty of multiplexing also applies to in vivo studies: Dyno can test hundreds of thousands of capsid designs in a single primate, dramatically reducing the cost of these experiments.
Dyno has also open-sourced some of their internal software packages. Tech companies often do this to contribute back to the open-source communities they benefit from. It also signals to potential engineering hires that they are doing serious and high-quality work. This practice is much rarer in biotech.
This was a simply awesome exposition! Anyone not excited by the history and panoramas and potentials you show us are simply asleep! I can't imagine how much work you put into this. Thanks so much.
Hi Elliot, I have adult onset epilepsy. It could be easy to get discouraged at the prospect of leaving my wife a widow or my son without a dad. Instead, reading this I’m filled with optimism at the prospect of a capsid delivered gene based cure if I live another 10 years or so.
I’m incredibly thankful for the people at Dyno. Thanks to them for their work.
Elliot, I’m also thankful for your journalism. Thank you too!