Welcome to The Century of Biology! Each week, I post a highlight of a cutting-edge bioRxiv preprint, an essay about biotech, or an analysis of an exciting new startup. You can subscribe for free to have the next post delivered to your inbox:
Enjoy! 🧬
Overview
A new study from the Pritchard Lab at Stanford has been sweeping through the world of human genetics and genomics. It’s been the focus of discussion in lab meetings and calls between collaborators, and of course, on Science Twitter, ever since it was posted on bioRxiv. Here, I’m talking about the work led by Hakhamanesh Mostafavi describing a new model that explains the “Limited overlap of eQTLs and GWAS hits due to systematic differences in discovery.”
So what do the results of this study mean? Why has it generated so much conversation? My goal here is to provide context for this work and to unpack the model that was proposed to help answer these questions. Here’s where we’re going to go:
A 30,000 foot view of human genetics
GWAS primer
eQTLs
The new model
Future implications
Let’s jump in! 🧬
A 30,000 foot view of human genetics
The aptly named field of human genetics seeks to decode the relationship between the human genome and the traits that it encodes. This work involves identifying genetic variants—differences in the bases of DNA between individuals—and understanding how they lead to the wide range of human variation that we observe. For example, what genetic variants encode the enormous amount of variation in human height?
This type of question can be asked for practically any measurable human trait, but a major focus is to go “from variant to function in human disease genetics.” As Lappalainen and MacArthur point out, genetics is an enormously powerful tool for mechanistic research:
The human population, through explosive growth, has performed a comprehensive saturation mutagenesis experiment on itself. It is now the case that any single base substitution that is compatible with life is expected to be present somewhere among the nearly 8 billion living humans. Humanity has thus, in effect, done many of the natural experiments required to understand our own genotype-phenotype map; this leaves geneticists to catalog the outcomes of those experiments, and to leverage both observational and experimental approaches to understand the mechanisms by which variants alter biology.
I love the simplicity and elegance of this strategy. We need to carefully observe Nature, and let the variants tell us how disease biology works. Variants that are frequently observed for a given disease are like bread crumbs leading us toward an understanding of the genes that they are impacting. This helps to explain why drug targets with genetic evidence are twice as likely to lead to approved drugs.1
Another direct extension of human genetics is personal genomics. We can use our continually expanding catalog of annotated genetic variants to interpret individual genomes. Companies such as 23andMe and Color represent the first wave of this type of consumer offering.
GWAS primer
Okay, so we want to make the connection between genetic variants and human traits. How can we do that?
One of the major tools of modern human genetics is the Genome-wide Association Study (GWAS). The most common setup for a GWAS is to conduct a case-control study where two different groups are measured: a healthy (control) group, and a case group with the trait of interest—which is normally a disease.
The measurement in a GWAS is most often of single nucleotide polymorphisms (SNPs)—which are variants in individual bases of DNA (nucleotides). With the SNPs measured in both groups, the goal is to figure out which ones are most commonly associated with the case group. This is done with fairly standard statistical techniques, where a big linear regression model is fit with the trait as the response variable and the SNPs as the explanatory variables.2
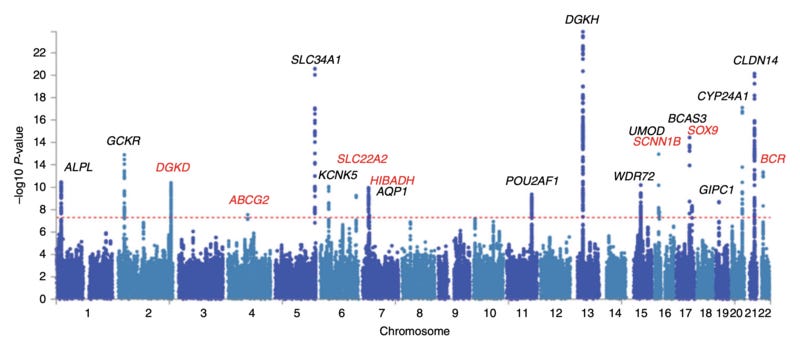
The Manhattan plot is the main way to display the results of a GWAS. It represents all of the SNP locations in the genome along the X-axis, and the p-value describing the significance of the association is shown on the Y-axis. The higher the peak, the more significant the association.
By this point, thousands of GWAS have been conducted. We have found genetic associations for a huge number of traits. For recent studies with massive sample sizes, we’ve even begun to account for nearly all of the SNP-based heritability for a trait. In other words, we’re reaching the limit of what we should be able to predict for a trait based on just genetics.
This sounds great! Problem solved? Do we now have a clear picture of disease genetics?
There has been a surprising result across nearly all GWAS: around 90% of variants found to be associated with traits fall outside of regions of the genome that actually encode genes. This introduces a serious wrinkle. The bread crumbs that were meant to lead us to disease genes have revealed a much more complicated picture. The variants are now thought to be changing how genes are regulated—dialed up or down—instead of directly modifying the proteins they encode.
eQTLs
Once you’ve grokked the idea behind GWAS and what has been found so far, eQTLs make a lot of sense. First of all, QTL stands for Quantitative Trait Locus—which is a section of DNA that is associated with a trait that varies continuously, like height. At the end of the day, a GWAS of height could be said to be finding hQTLS.
You can map QTLs for anything you can measure, pQTLs for protein levels, mQTLs for DNA methylation, so on and so forth. An eQTL is a genetic locus that leads to a difference in gene expression. Given that GWAS found so many noncoding variants that appear to be involved in regulating genes, this is a logical type of mapping to do.
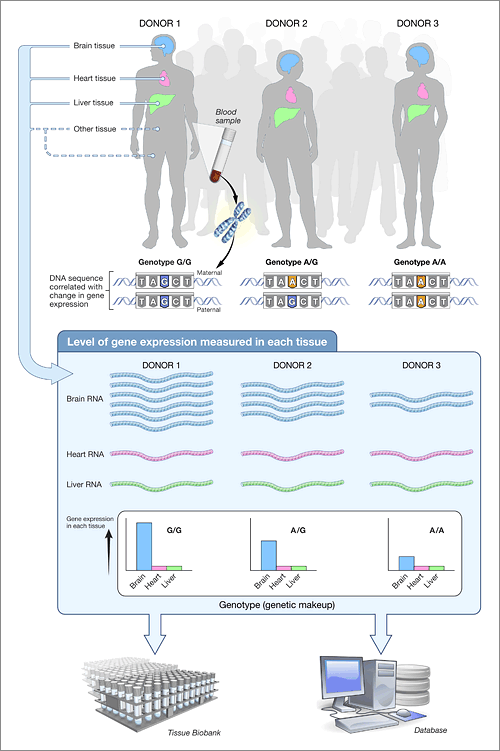
One of the flagship efforts in eQTL mapping has been the Genotype-Tissue Expression Project (GTEx), which analyzed massive biobanks of human tissues to look at how variants impacted gene expression. GTEx has detected millions of genetic variants significantly associated with changes in gene expression.
This catalog of variants can be used to figure out which genes are dialed up and down in different tissues in the body. It can also be useful for providing evidence for what GWAS variants are doing. By doing colocalization, it’s possible to overlap GWAS signals with eQTL signals—finding cases where there is a shared genetic variant. For the signals that overlap, it provides evidence that the variant is leading to impacting the trait by changing how a gene is expressed.
This is where the plot thickens again. As this new study points out, “just 43% of GWAS hits and a median of 21% of GWAS hits per trait, colocalized with eQTLs. Similarly, it has been estimated that only 11% of heritability for complex traits is mediated by gene expression in GTEx tissues.”
What explains this big disconnect?
The new model
One reason for the lack of overlap could be that we aren’t actually finding all of the eQTLs in the first place. Biology is really complicated. A variant could be changing gene expression only in specific cells, or at very specific time points. There has actually been evidence for this so far. I recently wrote about another preprint that is an example of this: they found thousands of eQTLs that were only detectable at single-cell resolution when immune cells became activated. Another bottleneck could be the tissue quality in GTEx, and the bias that comes from only being able to access adult postmortem samples.
While the explanation of context specificity makes intuitive sense, the new studies looking for eQTLs in different places have only added small amounts of overlap with GWAS variants. Now, this new study has proposed a model based on evolutionary theory to explain this.
The model is based on the fact that human traits are shaped by the forces of natural selection. Critically, it has been observed that selection acts against variants with a large effect size. This is called selective constraint.
Evolution is a slow process—selective constraint is like a stoplight where you are penalized for introducing too big of a change. This study looked for evidence of selective constraint in both GWAS variants and eQTLs. Interestingly, they found evidence for selective constraint in genes nearby GWAS variants, but not eQTLs.
A number of other features differed when they compared GWAS results and eQTLs. The model in this study proposes that this is the case because “selection affects the GWAS and eQTL assays differently, mostly preventing the discovery of eQTLs at important genes.”
The idea is that eQTL studies are powered to detect variants with a large effect on expression, and those variants are negatively selected against at the level of the trait. This model proposes a new explanation for the observed lack of overlap between the two types of studies so far.
Future implications
This model is a big deal for human genetics. It proposes that we should modify our expectations of the amount of overlap between two of the biggest types of studies in the field: GWAS and eQTL mapping.
We should continue to see colocalization serve as a useful tool for understanding GWAS variants, and look for QTLs in more specific contexts. With that being said, we should keep in mind how selective pressures impact the discovery power of different study designs.
The authors also highlight different tools that can help mitigate these shortcomings in identifying the function of GWAS variants. Approaches like the sequence-based models I’ve written about, MPRA experiments, and CRISPR screens can all help to analyze the variants that may fall outside of the discovery horizon of eQTL mapping no matter how large sample sizes become in the future.
At the end of the day, a combination of strategies will be required to go from variant to function in the post-GWAS era.
Thanks for reading this highlight of “Limited overlap of eQTLs and GWAS hits due to systematic differences in discovery.” If you don’t want to miss the next preprint highlight, biotech essay, or startup analysis, you can subscribe to have it delivered to your inbox for free:
Until next time! 🧬
A study describing this was published in 2015, and validated with additional modeling in 2019.
One technical point is that statistical power increases with sample size. Because of this, most GWA studies do not using DNA sequencing for measurement. Instead, they use SNP microarrays, which are much more scalable measurements that can rapidly detect the most common SNPs. The downside is that this biases discovery out of the gate, and misses larger structural variation.
Cool study, thanks for posting! So how large should be the fold change in expression to constitute an eQTL?
Thank you for this very interesting essay! Do you have a reading recommendation for some one who want to understand eQTL better? Thanks again!