All civilizations either become spacefaring or extinct.
- Carl Sagan
Introduction
As you may know, I’m pretty excited about the amount of progress happening in biology right now. Extraordinary advances in DNA sequencing, DNA synthesis, and gene editing technologies are radically shifting what is possible. I’ve been writing this newsletter for less than a year, and have covered the completion of the human genome, new breakthroughs for deep learning in genomics, studies perturbing every gene in the genome, and much, much more.
Outside of biology, one of the most exciting technological trends is humanity’s rapid re-acceleration in space exploration. In the time since the first moon landing in 1969, there has been a measurable stagnation in global ambitions for human space exploration. This trend has dramatically shifted in recent years. We are coming off of an incredible decade in space, where SpaceX has propelled us towards a future with fully reusable launch systems—forever altering the economics of space travel.
This shift in space economics has been followed by a new surge of venture capital into the area. The level of ambition in this new wave of commercial space startups is astonishing: companies like Relativity Space are aiming to create fully 3D printable launch vehicles (!!!), Rocket Lab is working to further commoditize space launches, and Planet is using space to change the resolution of our view of Earth. Other newcomers include Varda Space Industries, who is looking to use space factories to manufacture new types of products, and Hadrian, which is aiming to solidify the manufacturing supply chain that makes all of this innovation possible.
NASA has also puts its foot on the gas with the launch of the Artemis missions that aim to “collaborate with commercial and international partners and establish the first long-term presence on the Moon.” In combination with the Lunar Gateway being established, the goal is to “use what we learn on and around the Moon to take the next giant leap: sending the first astronauts to Mars.”
We could see humans on Mars as early as 2030. Space travel is becoming a reality. This is enormously consequential for the future of the human race. As Carl Sagan said, “all civilizations either become spacefaring or extinct.” The accelerated progress in space exploration intersects with the Biology Revolution in interesting ways. As I alluded to last week, plant synthetic biology could be essential for establishing a multiplanetary future.
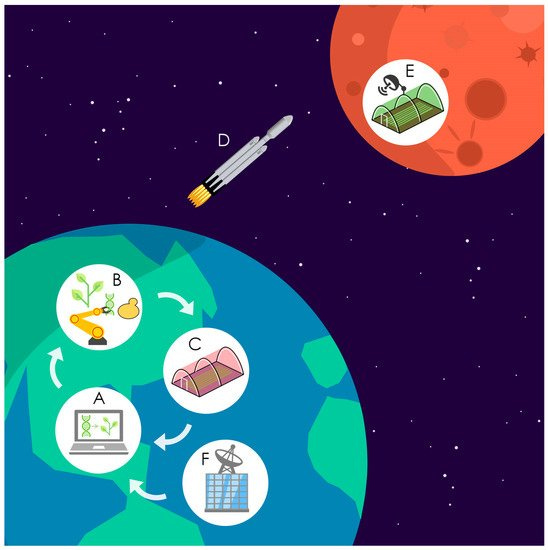
In a great paper on this topic, the authors argue that “in addition to generating oxygen, fixing carbon, and recycling waste and water, plants could play a critical role in producing food and biomass feedstock for the microbial manufacture of materials, chemicals, and medicines in long-term interplanetary outposts.” Achieving this will require continued advances in our ability to engineer biology.
Another crucial research goal is to understand the impact of space travel on human biology. Our bodies have evolved to contend with the stresses of our biosphere on Earth, but space travel presents an entirely new set of environmental assaults—such as greater radiation exposure and microgravity. Top researchers have begun to collect and use large-scale omics data to comprehensively analyze the impact of space on our physiology.1
Humans aren’t the only organisms that we have to consider when evaluating the impacts of space travel. While we are traveling on spaceships, microbes are traveling on us. Microgravity has been shown to alter bacterial growth patterns and kinetics, and radiation increases the frequency of mutations—in both cases creating opportunities for increased antimicrobial resistance—all in an environment where astronauts immune systems are compromised. The International Space Station (ISS) has a complex microbiome that we fundamentally alter, and that in turn alters us.
It’s important to be able to understand the genetic basis of antimicrobial resistance in space. A team of researchers carried out a study entitled “Machine learning algorithm to characterize antimicrobial resistance associated with the International Space Station surface microbiome” with the goal of addressing this question.
This work was led by Pedro Madrigal from Cambridge University, and the senior authors are Kasthuri Venkateswaran from the Jet Propulsion Laboratory at Caltech, and Afshin Beheshti from NASA Ames Research Center. Chris Mason, who has been one of the leaders in this field, was also an author on this study.2
Key Advances
In an effort to better understand microbes in space, NASA has begun to measure them at a higher resolution. A new NASA Microbial Tracking initiative got off the ground in 2015 with MT-1 when the SpaceX-5 Dragon delivered microbial sampling supplies to the ISS. This new initiative represented an important shift from previous microbial sampling in space. Instead of relying entirely on culture-based methods of sampling—where microbes are grown on plates into stable colonies—this project also used shotgun metagenomic sequencing.
Shotgun metagenomic sequencing is an approach that uses modern high-throughput DNA sequencing to measure the DNA present in a mixed sample of microbes. With the sample sequenced, it’s possible to use bioinformatics algorithms to deconvolve the aggregate sequencing reads back into separate metagenome-assembled genomes (MAGs).
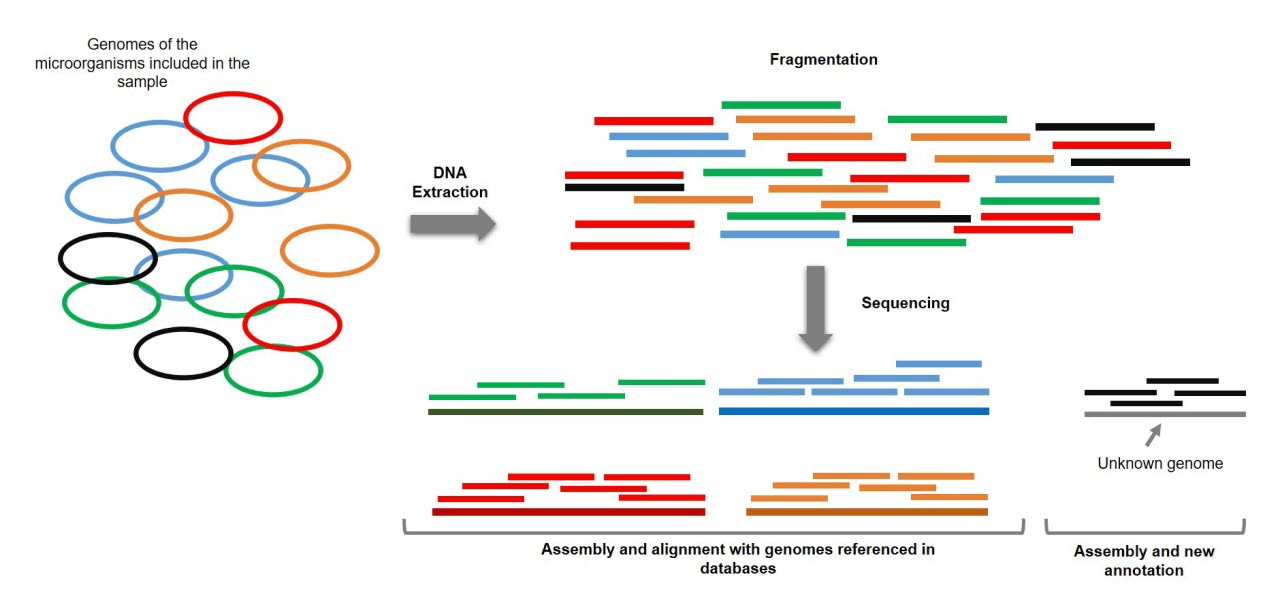
This type of shotgun sequencing is crucially important because it enables detection of microbes in the environment without culturing them, seeing as only 1-3% of microbes are able to be cultured. It’s worth noting that this type of approach has only been made possible by the incredible improvements in DNA sequencing, as well as in the hardware and software required to perform this type of analysis.
The dataset from MT-1 consists of “227 cultivable strains, 21 shotgun metagenome sequences, and 24 MAGs retrieved from the ISS environmental samples” which were collected “across three flights in eight different locations during 12 months.” The goal of this study was to detect the genes that contribute to antimicrobial resistance from this data.
Predicting antimicrobial resistance genes is a non-trivial computational problem, especially when dealing with metagenomic data. In order to tackle this problem, they decided to use a new tool called DeepARG. As the name suggests, DeepARG uses a deep learning model to predict antibiotic resistance genes (ARGs). The input to the model is a matrix representation of the distance between an input sequence and all known ARGs in databases.3 The value of deep learning for this application is that the model can approximate complex patterns in sequence space without needing the model builder to specify them upfront.
With richer data and a more powerful detection algorithm, they set out to discover what antimicrobial resistant genes are present on the ISS.
Results
After using DeepARG to predict antibiotic resistance genes, they asked a simple question: what is the distribution of these genes across different classes of antibiotics?
To start, they saw that beta-lactam antibiotics are the class of drug with the highest frequency of predicted read counts, as seen in Figure 2a above. This finding was consistent with prior work by the authors on analyzing antimicrobial resistance (AMR) genes in this data—as was the overall number of predicted read counts between studies (shown in Figure 2b above). This is problematic but important to understand, because beta-lactam drugs constitute one of the largest classes of antibiotics in use.4
The first part of this analysis asked questions about frequencies in transcripts across all reads in the metagenomic data. In order to ask questions about resistance in specific microbes, they assembled the reads into metagenomes and predicted AMR genes within each assembly.
As may be expected, the number of AMR genes was higher in the data from the second and third flights. There was another important pattern: a considerable number of AMR genes were found in several different locations for Kalamiella piersonii—a microbe potentially involved in causing urinary tract infections. Worryingly, “the potentially very pathogenic microbe E. bugandensis was found in location 2 (forward side panel wall of the Waste and Hygiene Compartment) in flight 1, presenting more than 40 ARGs.” They were also able to detect specific types of potential drug resistance for several microbes within the Pantoea species—which provided a higher level of resolution into observations made in their past analysis.
How well do these predictions represent reality? In order to address this question, they used an assay called disc diffusion to measure AMR in E. bugandensis and B. cereus, which are two of the species with the highest potential for pathogenicity.
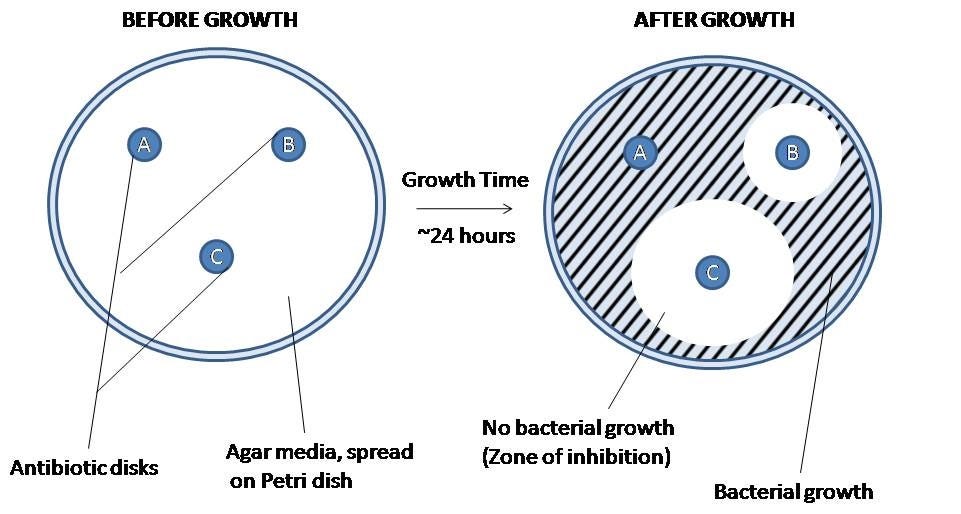
They compared the results of their assay to the computational predictions from DeepARG:
The key pattern in these heat maps is that the ARG count for these strains was highly correlated with the size of inhibitory zone and resistance classification. In other words, the model predictions were broadly validated by the experiments.
Final Thoughts
Space is a big, beautiful place. Despite the strange marketing campaigns of SaaS companies here on Earth, many dream of a future with robust space exploration. This beautiful collective dream is on the verge of becoming a reality. After decades of enormous innovation in computation, we have a fundamentally new toolkit for modeling and manipulating the world of atoms. It’s hard to not be excited about a new generation of scientists and engineers wielding this toolkit to build a multiplanetary future for our crazy, beautiful species.
Biotechnology will play an important role in space exploration. Relativity Space emphasizes the crucial importance of 3D printing macroscopic objects. In addition to rockets and larger infrastructure, we will need food, potable water, oxygen, medicines, and biomaterials. In the microscopic world, the only programmable system capable of 3d printing with atomic precision are cells.
Outside of our material needs, it will be essential to develop strategies to mitigate the environmental health risks posed by longer space missions. A critical component of this will be to monitor the changes over time to the environmental microbiome present on our space stations. This study addresses that challenge by using state-of-the-art DNA sequencing and machine learning to detect antimicrobial resistance genes on the International Space Station.
Thanks for reading this highlight of “Machine learning algorithm to characterize antimicrobial resistance associated with the International Space Station surface microbiome.” If you’ve enjoyed this post and don’t want to miss the next one, you can sign up to have them automatically delivered to your inbox:
Until next time! 🧬
One of the critical new resources in the field of space biology is the NASA GeneLab, which is a database worth exploring, especially if you find this research area compelling!
It’s worth checking out the Mason Lab’s ten-phase, 500-year plan for space colonization. Mason has also co-founded several companies, and is an advisor along with Afshin Beheshti to a space biotech startup called Ursa Bio.
For the ML crowd, the formulation is based on dissimilarity based classification where the key idea is that “sequences were represented and featured by their identity distances to known ARGs.” They cite a paper using this for medical imaging as a motivating example.
This result may be explained by the large number of possible ways that antibiotic resistance can develop against this class of antibiotics.