Machine brains and their discontents
A reflection on BenevolentAI and the role of AI in drug discovery
Welcome to The Century of Biology! This newsletter explores data, companies, and ideas from the frontier of biology. You can subscribe for free to have the next post delivered to your inbox:
Enjoy! 🧬
The current AI discourse can be confusing to follow. On one hand, the Twitter algorithm has decided that everybody’s feed needs at least three threads about “tHe ToP X THinGs YoU MiSSeD ThiS WeeK in Ai!! 🧵” every day. We’re on the verge of massive productivity gains! On the other hand, things might be moving too fast—AI could pose an existential threat to humanity.1
While everybody is arguing about whether it’s more likely that we’ll quadruple our GDP or cause an extinction event in the next five years, some people are marching along trying to apply AI to hard problems. The harder the problem, the more abundantly clear it is that AI is nowhere close to a panacea. One of these hard problems is drug discovery.
Recently, BenevolentAI—one of the clinical stage drug discovery companies most vocal about AI being their secret sauce—announced they are laying off 180 employees in a restructuring. This news came on the heels of their recent clinical failures—a case of “BenevolentAI, Cruel R&D.” Derek Lowe covered this story in his great In The Pipeline blog, saying:
“There are no existing AI/ML systems that mitigate clinical failure risks due to target choice or toxicology.”
This meme rapidly propagated throughout a substantial portion of my biotech network. In large part, I think this is explained by the fact that many inherently skeptical people in the life sciences are frustrated by AI evangelists overpromising and underdelivering. As Derek Lowe pointed out, BenevolentAI probably shouldn’t have originally claimed to have built a “bioscience machine brain.”
While AI certainly isn’t a panacea, it becomes more apparent each day that it is a powerful paradigm for modeling the immense complexity of biology. As I’ve previously argued, biology is the field in the world of atoms that has benefited the most from AI to date. AlphaFold is genuinely remarkable, and ML models are increasingly state-of-the-art across most prediction tasks in computational biology.
So, how should we reason about the juxtaposition of amazing research breakthroughs and lackluster clinical results?
I’ve had a lot of interesting conversations about this question this week, so I’ve decided to pause the piece I was initially working on and distill my current thoughts on this topic here. This is my version of “When you’ve given the same in-person advice 3 times, write a blog post.” Rather than advice, it’s an attempt to improve my own thinking about this.
I think part of the confusion here is around how drug discovery businesses are actually structured, and where AI is currently making the most progress in biology. We often talk about drug discovery as a very linear and defined process that looks something like this:

If Derek Lowe is right and no AI system delivers value for target identification—the very first step in the pipeline—how can it be useful for anything else? Reality is more nuanced. As biotech businesses become more specialized, I find that it can be useful to stratify biotech platforms into two buckets: target companies and modality companies.2 So far, AI advances have been more valuable for modality companies than for target companies. Let’s break down why that is the case.
Modality companies
In the simplest framework, the process of discovering drugs consists of identifying biological targets—nodes in a vast network of biochemical causality that play a critical role in disease onset—and using a therapeutic modality to interface with the target to restore human physiology to a healthy equilibrium. Modality businesses focus on the second half of this equation by building platforms to commercialize totally new modalities like engineered cell therapies or dramatically improving the engineering process for existing modalities like small molecules, big molecules (mainly antibodies), or nucleic acid therapeutics.
These are companies building hammers and searching for nails.
This problem is very amenable to AI. By focusing on a specific engineering problem, it’s possible to reduce the scope of biological complexity you’re tackling and create a relevant dataset for model creation. A new wave of tech-enabled biotech companies is pursuing this business model. One example is Dyno Therapeutics, which uses AI to engineer vastly improved Adeno-Associated Virus (AAV) vectors for drug delivery. They just released some really beautiful data at the ASGCT conference showing their progress:
This data shows the difference between AAV9 and the new Dyno bCap 1 delivery vector for pan-brain transduction. Dyno claims that bCap 1 shows a “100x improvement versus AAV9 in delivery to the central nervous system (CNS) and 10x detargeting of liver after intravenous (IV) dosing, as characterized across multiple non-human primate (NHP) species.” The new vector also has a 1x increase in production efficiency.
To be clear, this isn’t a pure AI win. It’s a demonstration of the 4-S model in action. Dyno has built a data platform using advances in high-throughput DNA sequencing and synthesis, creating a large-scale catalog of the AAV fitness landscape. This is the foundation for their ML models—which can be used to design substantially improved delivery vectors.
The structure of this modality-focused platform has led to a business model Dyno describes as “partnership-centric: We partner with gene therapy developers, providing them with the very best capsids so that they can invest their efforts at the leading edge of genetic medicine.” This is the same playbook that AbCellera is using for antibodies.
The list of modality-focused AI platforms is growing. BigHat Biosciences is using a similar toolbox to accelerate antibody design—and many new protein engineering companies are entering this market. Coding.bio uses AI to design better CAR-T therapies, and Mana Bio is looking to do the same for lipid nanoparticle design. This is just a small sampling to illustrate the activity in this space.
The rapid advances in AI-driven structural biology could accelerate progress toward the holy grail of rational drug design—designing small molecules based on atomic insights about the biological target. This could be true even for targets where we have historically had poor structural information. An example is Atomic AI, which aims to open up the world of RNA-targeting small-molecule drug discovery using its AI-based RNA structure engine.
I actually think it’s under-appreciated how close we are to commoditizing therapeutic modalities. This sounds hyperbolic, but even without AI, Contract Research Organizations (CROs) like WuXi are getting incredibly good at offering medicinal chemistry as a service. This is why it is possible for virtual biotechs to exist in the first place. And if we zoom out, what will the world look like if many of these AI-driven modality-focused platforms succeed over the next 10-20 years? As Derek Lowe would put it, what if we can actually compute our way to antibodies for everything?
In this future, the pendulum would swing back to the challenging problem of target identification.
Target companies
If we could design a medicine against any target, which targets should we be drugging in the first place? This is the question that target companies try to answer. Asset-centric biotech companies are built around specific insights into a small handful of targets or compounds. Another approach is to build a discovery platform based on a novel data source or technology for uncovering new targets that play a central role in disease progression. Increasingly, these businesses outsource the process of modality development to specialists like WuXi, AbCellera, or other service providers.
So far, stories like BenevolentAI’s underperformance have highlighted the fact that AI approaches to this portion of drug discovery are still nascent. On a technical level, this makes sense. Whereas Dyno can focus on building the best possible dataset for AAV biology, it’s a much harder problem to model all of human physiology.
For this reason, the true advantage in target discovery still lies in data novelty—or the novelty of new data-generating technology. AI will likely still provide analytical value, but its role will be secondary. Given the current state of technology, no AI system alone will provide enough of an advantage to build a generational platform.
Let’s look at a few examples. It’s hard to think of a more iconic measurement tool in biology than the microscope. There’s a good reason for this: it’s essential to understand the spatial organization of living systems at the cellular and tissue level. Eikon Therapeutics is betting that advances in live-cell super-resolution microscopy will provide a new window into disease biology—making it possible to find new drug targets and drugs for previously undruggable targets.3 Computation and AI will play a role in analyzing the petabytes of data they generate, but imaging is the platform's foundation. I’m generally very excited about the value new spatial technologies will bring to drug discovery.
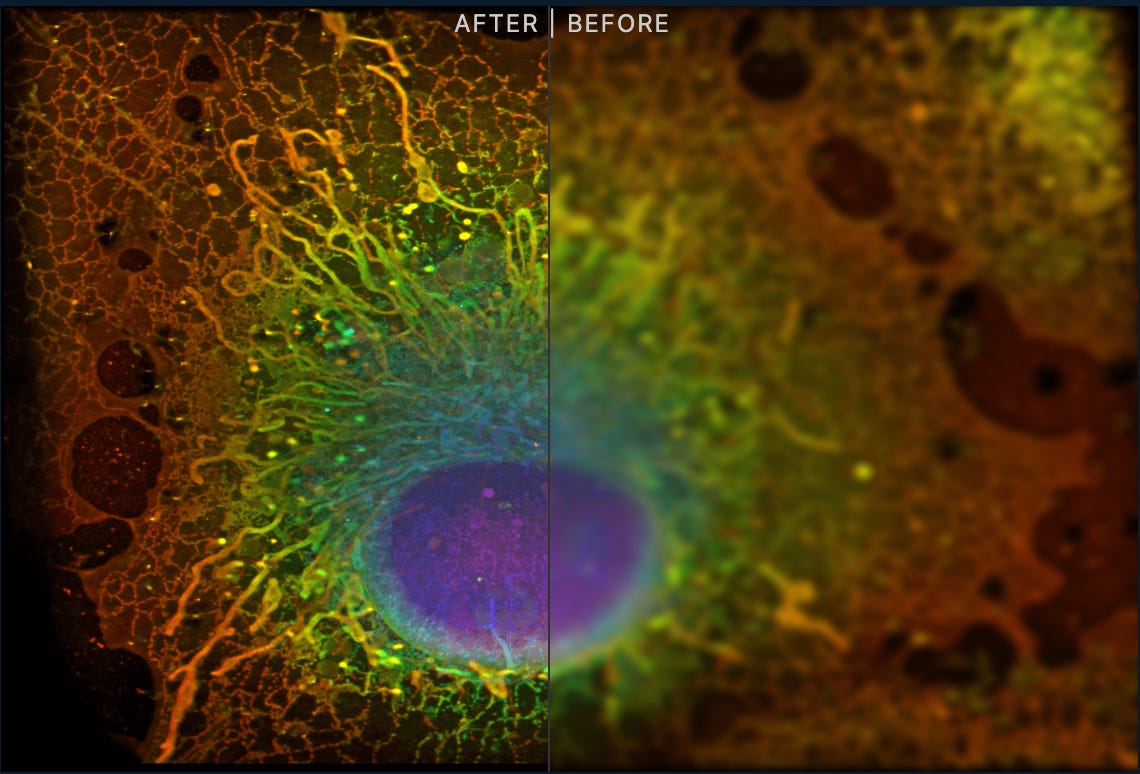
Another place to look for new biological insights is across the Tree of Life. In Extreme Biology, I highlighted the work that Fauna Bio is doing to build a novel biobank of hibernating mammals to uncover new drug targets for human diseases, including pulmonary disease, heart failure, obesity, and neurodegeneration. Again, the rich new data is central, but Fauna is building a machine learning platform to unlock its full value.
Fauna is modality agnostic. They say, “While our platform has the ability to directly predict new uses for small molecules, we are not limited to a single modality. We find the best genes to target for diseases with high unmet need and can identify genes for to target with a broad number of modalities, from RNAi technologies to antibodies.”
The choice of modality is the wrong parameter to fix—they are a target company.
Looking forward
Machine learning has delivered the most value on well-defined prediction problems with large datasets and tangible benchmarks so far. For computer vision, this was the ImageNet Moment. For proteins, the AlphaFold Moment was based on the Protein Data Bank and the CASP challenge. Unsurprisingly, the initial wins in AI-driven drug discovery have been primarily in modality engineering, where it’s easier to build high-quality datasets and measure progress.
The problem of target identification is still primarily rate-limited by our measurement technologies, the quality of our disease models, and the scarcity of clinical data. While AI will play an important role in analysis, companies that have built their entire platform around new models have struggled to deliver clinical value.4
It’s important to avoid indexing too heavily on the current state of technology when considering what the future might look like. In the fullness of time, achieving something like the Virtual Physiological Human should be a North Star for biomedical research. That will require better measurement technologies and more data, but it will also likely require trainable computational models capable of approximating complex non-linear functions… otherwise known as AI. We can’t continue with our current approach to “mechanistic descriptions” of biology indefinitely.
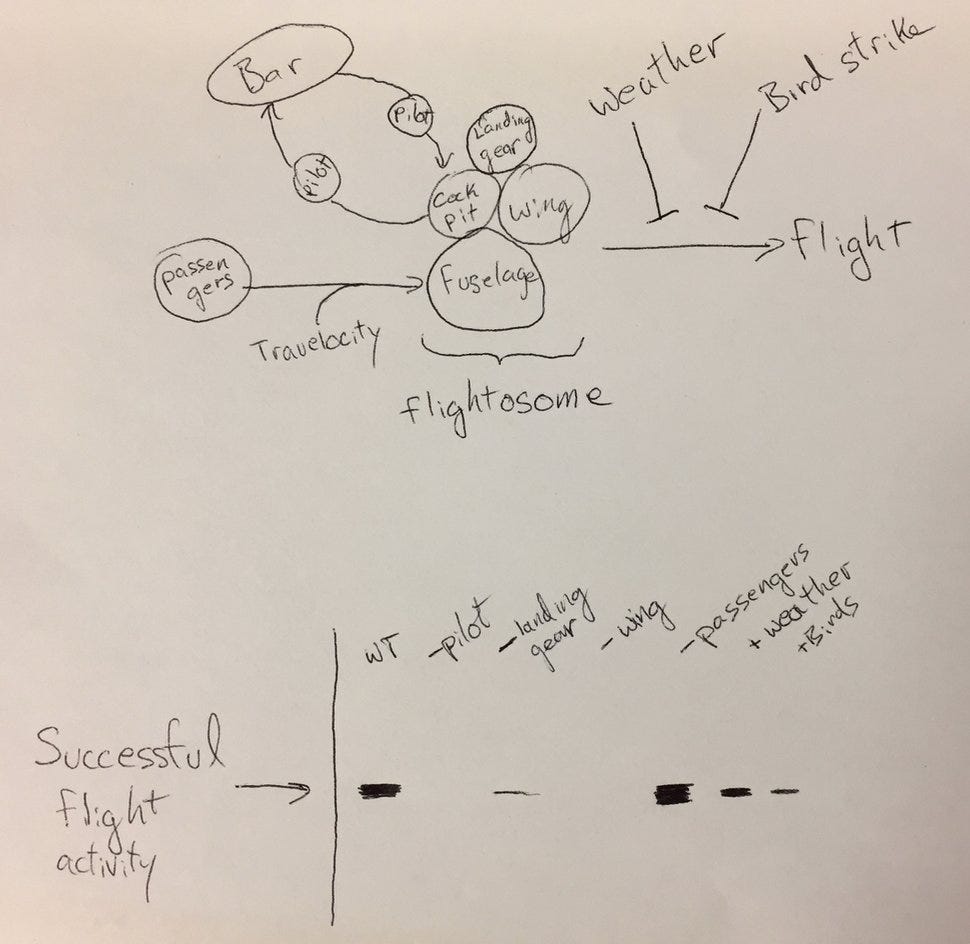
And what happens if we commoditize modality development and have a rich set of precise targets generated from our AI model of human physiology? We’ll still need to solve the enormous and messy clinical trial bottleneck. This is something I’m currently obsessed with, but that’s a story for another day.
Thanks for reading this essay about the promise and pitfalls of AI in drug discovery. If you don’t want to miss upcoming essays, you should consider subscribing for free to have them delivered to your inbox:
Until next time! 🧬
I’m deeply skeptical about the entire AI alignment discourse, but that argument is outside the scope of COB.
This obviously an imperfect simplification, but it can be a useful mental model.
This example also shows the shortcomings of the targets and modalities framework. Eikon is extremely well-financed, and while it’s difficult to analyze private companies, they seem also to be building out their own medicinal chemistry capabilities. As companies get larger, they can build competency across the entire pipeline to become a full-stack discovery company.
It will be interesting to track success rates for the broader set of AI-driven therapies making their way into the clinic—especially for Insilico Medicine, which also claims to do end-to-end drug discovery using AI.
Elliot, great piece! Would love to hear to your thoughts on the clinical trial bottleneck and potential solutions/companies that are tackling this massive problem. I really think solving the clinical trial problem will allow for a complete realignment of the pharmaceutical/biotech industry around transformational therapies, preventative medicine, and a focus on the health afflictions of the many not just the rare diseases of the few (still important).
During the last biotech boom in the 2000s I read where a consortium (or was it just Germany?) we're essentially building a human body with software that could simulate how novel drugs would interact and thereby sidestep some of the "clinical bottleneck". I haven't really heard much about it since--Im sure it was way to complex to tackle 20 years ago, probably even today, but is anyone working along these lines?