Welcome to The Century of Biology! Each week, I post a highlight of a cutting-edge bioRxiv preprint, an essay about biotech, or an analysis of an exciting new startup. You can subscribe for free to have the next post delivered to your inbox:
Enjoy! 🧬
When the completion of the Human Genome Project was announced, Bill Clinton said “genome science will have a real impact on all our lives -- and even more, on the lives of our children. It will revolutionize the diagnosis, prevention and treatment of most, if not all, human diseases.” While there is a certain political embellishment to this statement, it also accurately conveyed the hope of many scientists that this milestone would be transformative for human health.
Now over two decades later, we can think about what genomics has done so far. It has greatly improved our understanding of cancer—which is a fundamentally genetic disease—and has become a foundational resource and technology for many other areas of biomedical research. It’s also undoubtedly accelerated our understanding of human genetic variation and its role in health in disease. But why hasn’t the completion of a reference map of all of human DNA led to a more immediate windfall of far more new therapies and diagnostics? What do we need to understand in order to realize the promise of the Human Genome Project?
The answer is complex and multifaceted. A central reason is that there is rarely a one-to-one correspondence between knowing a sequence of DNA and understanding its function. When researchers analyze what genetic mutations are associated with disease, the majority fall outside of regions of the genome that encode genes. If they don’t encode genes, what do they do?
![[6 columns by 2 rows. The second row is a sideways view of the item in the first row. The first column (A) has four orange colored cells labelled 'A', 'B', 'C', and 'D'. The second column (B) has 8 cells, the bigger lower orange ones labelled '1A', '1B', '1C', and '1D'; the smaller pink ones on top labelled '1a', '1b', '1c', and '1d'. The third column (C) has 12 cells with the bottom, orange, labelled '2A' through '2D', the middle, red, labelled '2a' through '2d' and the top, pink, labelled '1a' through '1d'. The fourth column (D) has 16 cells, bottom layer, orange, is '2A' through '2D', top, pink, is '1a1' through '1d1', the middle alternates between larger red cells labelled '2a' through '2d' and smaller beige cells labelled '1a2' through '1d2'. The fifth column (E) has at the bottom orange cells labelled '3A' through '3D', at the top a cross of four pink cells labelled '1a1' through '1d1' and between the branches of the cross four beige cells labelled '1a2' through '1d2'. In the middle are large red cells labelled '2a' through '2d' and squished between the orange, beige, and red cells are dark red cells labelled '3a' through '3d'. The last column (F) is different. On the top is a clump of cells colored blue, green, pink, and orange. On the bottom on the left side is an arrow pointed up labelled 'developmental time'. Next to it is a 6 rowed drawing. The first row on the bottom extends the full width of the column and contains the word 'zygote', the row above is split in two, on the left 'AB' and on the left 'CD'. The third row is split evenly in 4: green 'A', blue 'B', orange 'C', and red 'D'. The fourth row is split evenly into 8 each shaded the same color as the unit below (though with different intensities). The fifth row is split evenly into 16 and the sixth row into 32 following the same pattern as the fourth row.] [6 columns by 2 rows. The second row is a sideways view of the item in the first row. The first column (A) has four orange colored cells labelled 'A', 'B', 'C', and 'D'. The second column (B) has 8 cells, the bigger lower orange ones labelled '1A', '1B', '1C', and '1D'; the smaller pink ones on top labelled '1a', '1b', '1c', and '1d'. The third column (C) has 12 cells with the bottom, orange, labelled '2A' through '2D', the middle, red, labelled '2a' through '2d' and the top, pink, labelled '1a' through '1d'. The fourth column (D) has 16 cells, bottom layer, orange, is '2A' through '2D', top, pink, is '1a1' through '1d1', the middle alternates between larger red cells labelled '2a' through '2d' and smaller beige cells labelled '1a2' through '1d2'. The fifth column (E) has at the bottom orange cells labelled '3A' through '3D', at the top a cross of four pink cells labelled '1a1' through '1d1' and between the branches of the cross four beige cells labelled '1a2' through '1d2'. In the middle are large red cells labelled '2a' through '2d' and squished between the orange, beige, and red cells are dark red cells labelled '3a' through '3d'. The last column (F) is different. On the top is a clump of cells colored blue, green, pink, and orange. On the bottom on the left side is an arrow pointed up labelled 'developmental time'. Next to it is a 6 rowed drawing. The first row on the bottom extends the full width of the column and contains the word 'zygote', the row above is split in two, on the left 'AB' and on the left 'CD'. The third row is split evenly in 4: green 'A', blue 'B', orange 'C', and red 'D'. The fourth row is split evenly into 8 each shaded the same color as the unit below (though with different intensities). The fifth row is split evenly into 16 and the sixth row into 32 following the same pattern as the fourth row.]](https://substackcdn.com/image/fetch/w_1456,c_limit,f_auto,q_auto:good,fl_progressive:steep/https%3A%2F%2Fbucketeer-e05bbc84-baa3-437e-9518-adb32be77984.s3.amazonaws.com%2Fpublic%2Fimages%2F8e0616ae-35da-4699-83e0-f23cb644dabe_2067x629.png)
In addition to genes, genomes encode a complex regulatory code. This code controls when genes are turned on and off. It is what enables genomes to contain the type of information necessary to guide a process as complex as organismal development. Because of this regulatory code, a single genome is capable of creating the enormous variety of cells that comprise the human body.
Gene regulation has been studied for a long time using many different approaches. More recently, sequencing-based technologies have enabled genome-wide profiling of many features involved in this process. This new data has led to one of the most active and interesting intersections of genomics and AI. Several research groups have now built highly accurate ML models that can quantitively predict various aspects of gene regulation directly from a sequence of DNA. This represents an important advance.
I’ve been really excited about this research direction. Last year, I wrote about some really impressive work directly predicting gene expression from DNA sequence. This type of work will be immediately useful in better understanding the regulatory code of the genome, and interpreting what some of the disease mutations that fall outside of genes are actually doing.
There is another tangible application of this work. Science is one side of a coin; the other side is engineering. If we can predict gene expression from DNA, can we also engineer DNA to encode specific expression patterns? This would effectively turn the field of synthetic biology into a more quantitative field. We could more systematically design genetic circuits, fine tuning our own building blocks instead of purely relying on regulatory components found in Nature.
This is starting to happen. I’ve previously highlighted some work in this direction, where researchers used generative ML models to design sequences of regulatory DNA to turn genes on or off. Now, in a beautiful new preprint, another group has designed cell-type specific enhancers. This work was led by Ibrahim I. Taskiran from the laboratory of Stein Aerts in Belgium.
So what does it actually mean to design a cell-type specific enhancer, and how did they do it?
Let’s define our terms. Genes have an architecture outside of the sequence that encodes a protein product. Upstream of this sequence is a promoter region, where the proteins necessary to transcribe the gene product physically bind.
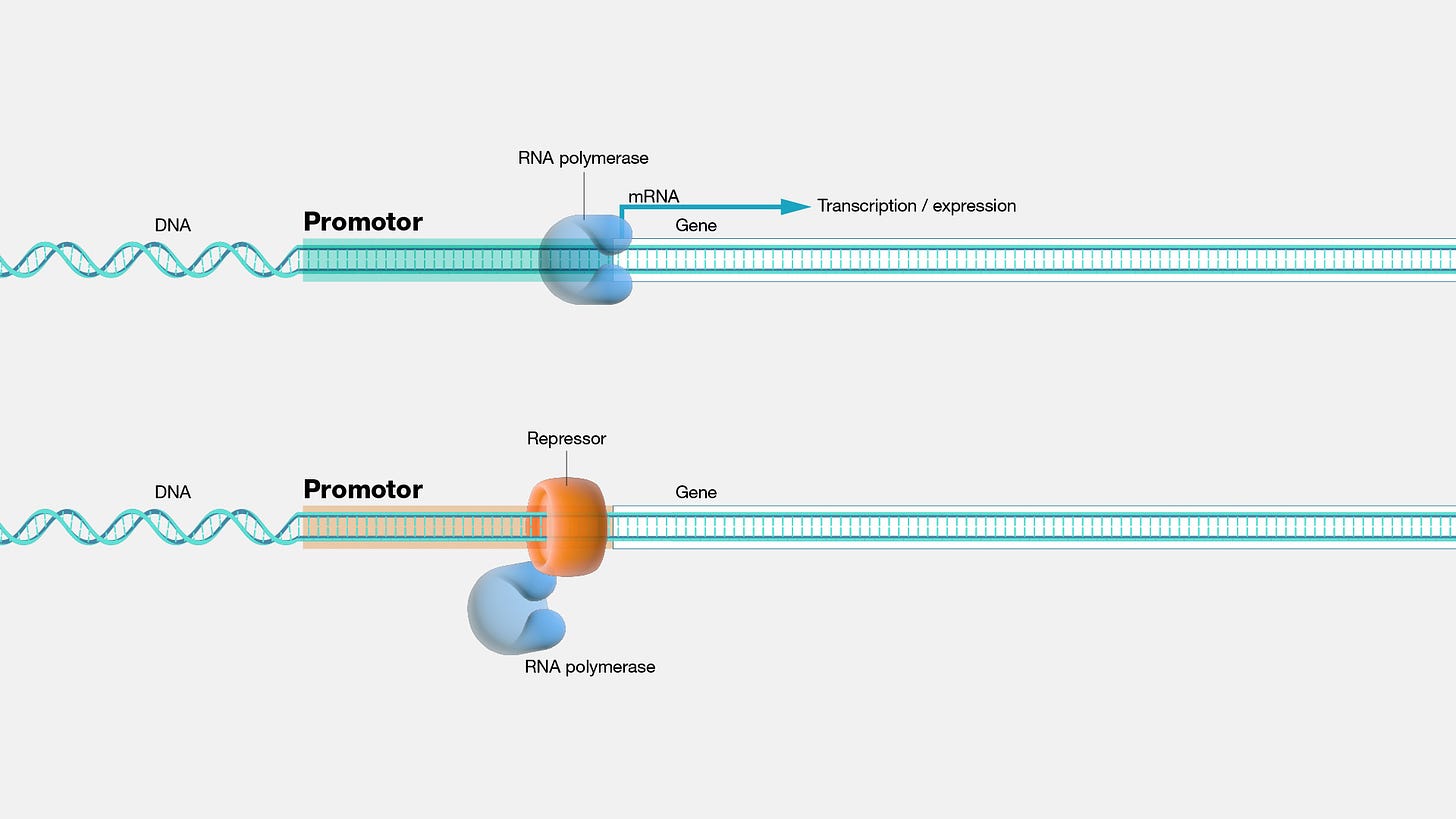
One of the central proteins in this process is RNA polymerase, which actually creates the RNA transcript. Transcription factors (TFs) are another essential class of proteins that bind promoters and regulate the transcription process. TFs also bind another type of regulatory sequence called enhancers. Enhancers are more poorly understood and harder to define, but the general view is that they “act as binding platforms for specific combinations of transcription factors.”
With these complex combinations of binding sequences, enhancers are able to control where genes are turned on in time and space. This can include regulating which cell types genes are expressed in. With an abundance of modern sequencing data, it has become possible to develop highly accurate machine learning (ML) models that can be used to tease apart these rules.
In this study, they used these models to guide the design of totally new enhancer sequences that expressed genes only in specific cells. They tried three different design strategies, and tested their performance using fruit flies and human cells. The experimental setup was straightforward:
They would design a new enhancer sequence, stick it into a fly, and check if it actually led to cell-type specific expression of their reporter gene using microscopy.
The first design strategy is equally elegant. The process would begin by generating 500 random bases of DNA. …ATCGATTA… Next, they performed saturation mutagenesis, where each base in the sequence would be modified. For each possible mutation, a prediction would be made using the deep learning model of enhancer activity.1 The mutation with the highest score for turning on their cell type of interest would be picked. This process would simply be carried out in a repeated fashion until the enhancer sequence had high predictions for their target.
It turned out that this worked really well. Amazingly, it would only take 15 mutations to go from the minimum score to the maximum score. As the authors point out, this may be an interesting insight into the natural evolution of enhancers, showing how regulatory sequences can emerge fairly quickly. It is also an impressive feat of engineering to so rapidly create functional enhancers, because the sequences worked:
Above, we can see the microscopy images looking for the reporter gene in the fly brain. The dashed circles represent the cells where the gene should be expressed. There is a clear difference between the positive and negative predictions for enhancer sequences.
As I mentioned, this study also demonstrated two other design strategies:
I’m not going to dive too deep into these strategies, because they are both slightly more complex ways to achieve the same result: designed enhancer sequences that worked in both fruit flies and human cells. One relied on embedding specific TF binding sequences (called motifs), the other used a GAN to systematically generate new enhancers.2
What is the take home message? This study is a compelling example of the immense promise at the intersection of AI and biology. It demonstrates where ML can have the highest impact: as a powerful tool to guide empirical engineering. Complex regulatory DNA sequences were designed purely in the world of bits. When they were manifested in the world of atoms, they behaved as expected.
Let’s extrapolate for a second. What if we developed a model that could not only design cell-type specific expression, but could design sequences that would drive gene expression in response to signals from the cellular environment. What if we put some of these enhancers into an engineered cell therapy that detected disease and expressed antibodies or mRNA therapies and released them to treat the condition?
I, for one, am excited for our sci-fi future.
Short and sweet this week! Thanks for reading this highlight of this exciting preprint. There won’t be a post next week, as I’ll be busy working on a larger piece that’s coming soon. You can subscribe to make sure you don’t miss it:
Until next time! 🧬
The model used for the fly sequences was DeepFlyBrain (great name), which was introduced in an important paper from the same group.
Thanks for sharing this artcile! In addition, AI technology can help us discover diverse and rare antibodies. You can learn more about AI technology by clicking https://ai.creative-biolabs.com/ai-based-one-stop-antibody-discovery-platform.htm
You have been using https://app.wombo.art/ for some of these images! (Me too.)