Life is a series of natural and spontaneous changes. Don't resist them—that only creates sorrow. Let reality be reality. Let things flow naturally forward in whatever way they like.
- Lao Tzu
Introduction
It seems like the Tao of Biotechnology is to learn to repurpose and harness the power of biology rather than to try to impose our will on it with force. Realizing this philosophy is only possible with a deeper knowledge of how basic biology works. In the early 20th century, one of the key tools used to introduce new DNA mutations was X-ray radiation. Model organisms such as fruit flies would be blasted with radiation to create new variants and map genes along chromosomes. Over time, we have carefully catalogued a variety of naturally occurring molecular tools that can be used to introduce DNA modifications. This toolbox includes systems like CRISPR—a discovered component of the bacterial immune system—that can be used to make programmed DNA edits.
The history of cancer treatment mirrors that of DNA modification. Because cancer is a genetic disease, one way to treat it is to destroy the DNA of cancer cells. This has been done by using radiation to shatter DNA—just like how we created fruit fly variants—for over a hundred years. The history of chemotherapy begins with literal warfare. Based on observations during World War I that mustard gas suppressed blood production, scientists developed an intravenous treatment for suppressing cancer using similar compounds.
One of the most impactful conceptual advancements in cancer therapy has been the realization that the human immune system can be harnessed to recognize and kill cancer cells. We have discovered that components of the bacterial immune system—like CRISPR—can be used to edit DNA. Now, we have learned that our own immune system is one of the most powerful and precise cancer medicines. In reflecting on this transition, one of the pioneers of cancer immunotherapy named James Allison said “In the 1980s, my laboratory did work on how the T cells of the immune system, which are the attack cells, latch onto the cells infected with viruses and bacteria and ultimately kill them. That research led me to think that the immune system could be unleashed to kill cancers.”
Advances in gene editing and cancer therapy have become much more precise and effective as we have learned to leverage our understanding of sophisticated evolved processes instead of assaulting cells with wartime compounds.1 We can achieve our desired outcomes by systematically engineering components of these processes to tip them towards an intended state. One beautiful example of this is chimeric antigen receptor (CAR) T cell therapy, which works by engineering a patients own T cells to produce a new receptor that targets them towards cancer cells.
We are only beginning to scratch the surface of what is possible with cell-based therapies. After the initial success of several cell-based therapies, researchers are now exploring ways to optimize their efficacy and safety, scale manufacturing, and even produce CAR T cells in vivo. In terms of optimizing efficacy, one engineering direction has been to explore further optimization of the co-stimulatory domains of CAR T cells—which are responsible for recognizing the second type of signal needed by a T cell to become activated.
Recent efforts to optimize these domains have primarily focused on testing different co-stimulatory domains found in natural T cells. A recent preprint entitled “Exploring the rules of chimeric antigen receptor phenotypic output using combinatorial signaling motif libraries and machine learning” took a fundamentally different approach. This study attempted to build a model to explain how signaling motifs—the basic building blocks of the co-stimulatory domains—can be combined in new ways to engineer better T cell phenotypes.
This study was led by Kyle Daniels, and the senior authors are Simone Bianco from Altos Labs2 and Wendell Lim from UCSF.
Key Advances
The defining characteristic of a CAR T cell is its engineered T cell receptor (TCR). TCRs are protein complexes present on the surface of T cells that are able to recognize and bind to molecules called antigens that initiate an immune response.
The engineered receptors present on CAR T cells are chimeric—the fusion of two or more genes—because they are designed to recognize and bind to antigens as well as to lead directly to activation when bound. A natural T cell in the immune system without a chimeric receptor requires two signals for activation: 1) antigen binding, and 2) a co-stimulatory interaction with molecules expressed by the antigen presenting cell. Successful “second generation” CAR T cells have incorporated co-stimulatory domains, which has improved their clinical effectiveness.
How can we better engineer co-stimulatory domains? This is a fundamental challenge. The authors point out that “a major goal in synthetic biology is to predictably generate new cell phenotypes by altering receptor composition.” In order to tackle this problem, the authors decided to strip down the domains to their constituent elements: the individual signaling motifs that are ultimately composed together into a receptor domain. They put forward the analogy that individual motifs are “words” whereas full co-stimulatory domains are “sentences.”
For their study, they used 12 signaling motifs and 1 spacer motif for a total of 13 motifs. They created a library testing every combination of up to three motifs possible, resulting in 2,379 (13 + 13^2 + 13^3) unique combinations. This library was used to perform a screen in T cells stimulated by the presence of Nalm 6 leukemia cells in order to explore the phenotypes generated by the different receptors.
There were two phenotypes of particular interest in this study. First, cytotoxicity is a measurement of the amount of target cells killed. Second, stemness is a T cell phenotype (measured by the presence of specific cell surface markers) that resembles the plasticity of a stem cell. Both phenotypes are associated with more effective CAR therapy. The large number of receptor combinations in their initial screen resulted in a wide range of observed values for both phenotypes.
In order to perform a more high resolution screen, they took a random subset of ~250 cars, and screened them on an array with four pulses of the stimulatory immune cells.
The next step in their work reflects an interesting change taking place in how science is done, so it is worth briefly thinking about what we are trying to accomplish when we model data. In 2001, the prominent statistician Leo Breiman argued that two cultures of statistical modeling had formed. The “data modeling” culture primarily assumes data are generated by a stochastic process that can be modeled and understood with certain statistical assumptions. In practice, scientists doing data modeling use models such as linear or logistic regression in combination with their hypotheses and prior knowledge about the domain to approximate the underlying function represented by their data.
At the turn of the century, Leo was beginning to see the formation of the “algorithmic modeling” culture, which took a fundamentally different approach to statistical modeling. This culture makes no assumptions about the underlying data generating function, and simply aims to use algorithmic techniques to create a predictive model.
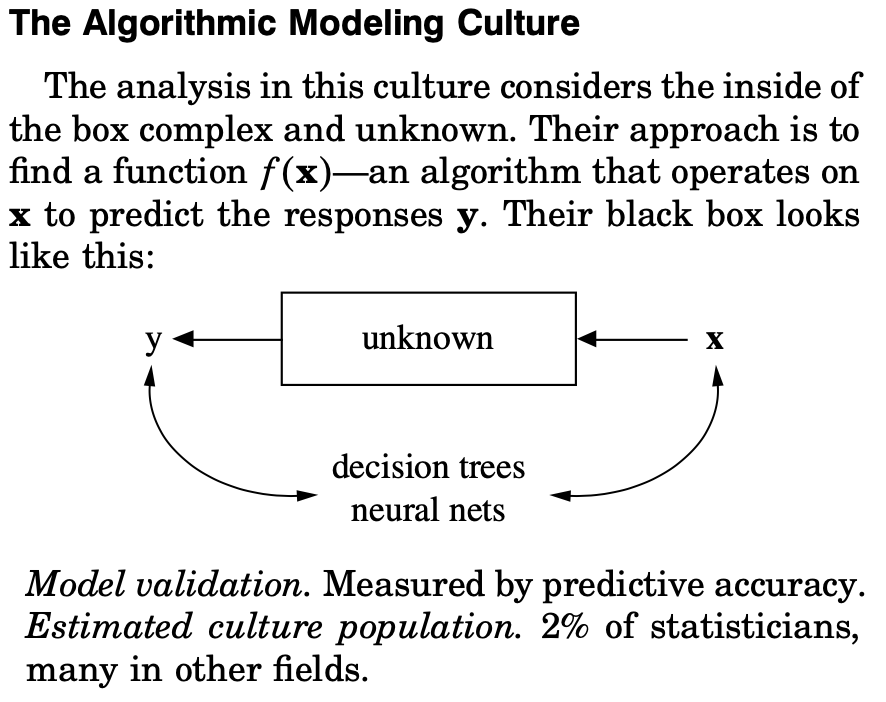
With breakthroughs in machine learning, the size of the population of scientists practicing algorithmic modeling has swelled relative to Breiman’s early estimations. This study is a clear example of using an algorithmic modeling approach to understand and engineer biology. The first thing that the authors did with the results of their arrayed screen was to train a neural network to predict the T cell phenotypes based on the receptor combinations.
While the arrayed screening data set wasn’t enormous, their models were “able to capture much of the relationship between signaling motif composition and phenotype, with R2 values of approximately 0.7-0.9.” With their predictive model, they were then able to effectively simulate the phenotypic response from each of the combinations present in their total combinatorial library of 2,379 receptor combinations. Using this approach, they worked to systematically reverse engineer the underlying grammar of how the signaling “words” (motifs) are composed to form “sentences” (receptors with phenotypic consequences) by exploring model predictions.
Results
What are important aspects of grammar? The analysis in this study focused on three fundamental components: word meaning, word combination, and word order. What does a given motif typically associate with? Do certain combinations of motifs drive certain phenotypes? Does their order within the receptor domain matter?
The figure above represents their findings about which pairs of motifs most effectively led to T cell cytotoxicity and stemness. It also appeared that the order within a domain did play a crucial role for some motifs:
Importantly, the grammar derived from their in silico simulations appear to be consistent with physical reality. Engineered receptors based on model predictions led to more cytotoxicity in vitro, and even demonstrably improved tumor clearance in vivo:
One of the reasons that I love computational biology is that it is a way for us to translate all of the progress from the world of bits back into the world of atoms. It is incredibly gratifying to see the predictions of an algorithmic model actually born out in molecular behavior.
Final Thoughts
Evolution is arguably the most beautiful generative process in existence. Over incomprehensible time scales, it has sculpted life on Earth into the abundance of diverse and complex patterns that we observe today. Living systems are chaotic, messy, and redundant—while simultaneously being capable of exquisite molecular precision that dwarves the capability of anything we can engineer. How can we develop tools and technology to exert any control over biology? As Feng Zhang says, “I think the way forward is to stay humble and to look to Nature for inspiration.”
This new study is inspiring. It represents the use of an algorithmic modeling approach to decipher the underlying grammar of how motifs combine together into receptor domains. By understanding this molecular language, we may be able to better engineer cell-based therapies to effectively treat disease. It reminds me of a quote by Demis Hassabis about this type of approach: “Biology is likely far too complex and messy to ever be encapsulated as a simple set of neat mathematical equations. But just as mathematics turned out to be the right description language for physics, biology may turn out to be the perfect type of regime for the application of AI.”
Thanks for reading this highlight of “Exploring the rules of chimeric antigen receptor phenotypic output using combinatorial signaling motif libraries and machine learning.” If you’ve enjoyed this post and don’t want to miss the next one, you can sign up to have them automatically delivered to your inbox:
Until next time! 🧬
To be clear, the current standard of care in cancer treatment is for the most part still primarily radiation or chemotherapy. However, while nothing is inevitable in technology development, it is likely that immunotherapy will only continue to gain ground as it matures and improves.
After the recent emergence from stealth mode for this new “rejuvenation programming” company with $3 billion in funding, an enormous number of prominent scientists announced publicly on Twitter that they were joining as principal investigators. It seemed a statistical certainty that at some point I would be writing about the work of somebody now at Altos, and it turns out that was correct!
I had bookmarked this previously and glad that I came back to it today. Really enjoyed this read. Thanks!