Another protein engineering breakthrough
A new protein design method from the Baker Lab for binding targets
The proteins that exist on Earth evolved to solve the problems faced by natural evolution. For example, replicating the genome. But we face new challenges today. If we had a million years to wait, new proteins might evolve to solve those challenges. But we don’t have millions of years to wait. Instead, with computational protein design, we can design new proteins to address these challenges today.
- David Baker
Overview
We are living through a renaissance in the field of protein design and engineering. This field focuses on developing the fundamental technologies and design techniques to create new proteins never before seen in Nature. It is hard to identify problems that this doesn’t apply to, because proteins are the primary machines harnessed by cells and living systems. Protein design holds the promise to create new molecular tools to serve as advanced therapies, universal flu vaccines, building blocks for materials science, and optimized solar energy capture machines.
For many years, researchers around the world have been working to solve two foundational challenges in the field: protein design and protein folding. The protein design problem consists of starting with a desired protein structure and determining the sequence that will fold into that conformation. Protein folding is the inverse problem: the goal is to predict the structure that a given amino acid sequence will fold into.
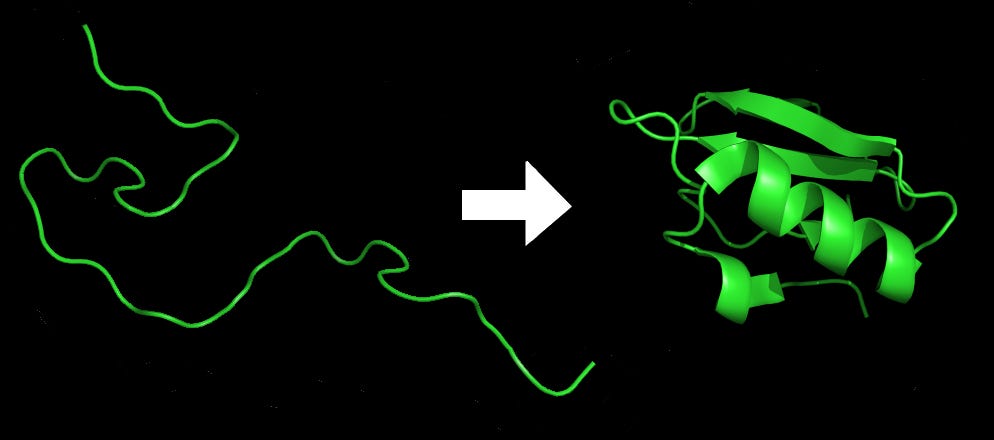
One of the pioneering groups in this field is the Baker Lab and the broader Institute for Protein Design at the University of Washington. This group created the de facto software suite for the field called Rosetta, and has always been a prominent contender in the Critical Assessment of protein Structure Prediction (CASP) competition.
Recently, CASP attracted the attention and efforts of Google DeepMind, which I’ve written a bit about before. At CASP14 in 2020, DeepMind’s performance was so impressive even relative to CASP13 that the organizers of the competition issued a press release declaring that the structure prediction problem has effectively been solved. It is hard to overstate how profoundly sci-fi and cool this is: it is arguably the first Nobel-level breakthrough for AI in natural science.
Apparently, this breakthrough has only caused the field of protein design to progress at a faster rate and to aim for even harder problems. This week, I’m highlighting a new preprint from the Baker Lab entitled “Robust de novo design of protein binding proteins from target structural information alone” which was led by Longxing Cao and Brian Coventry. This paper presents a new general design framework for building proteins by working backwards from information about the structure of the protein they are supposed to bind.
Key Advances
As I’ve mentioned, proteins are the workhorses of cells and living systems, which is why protein design holds the promise of being a universally applicable tool for biotechnologists. Protein-protein interactions are a central part of how proteins accomplish most biological functions. For this reason, techniques for impacting these interactions (such as blocking or regulating) are the foundation for therapeutics and biotechnology at large.
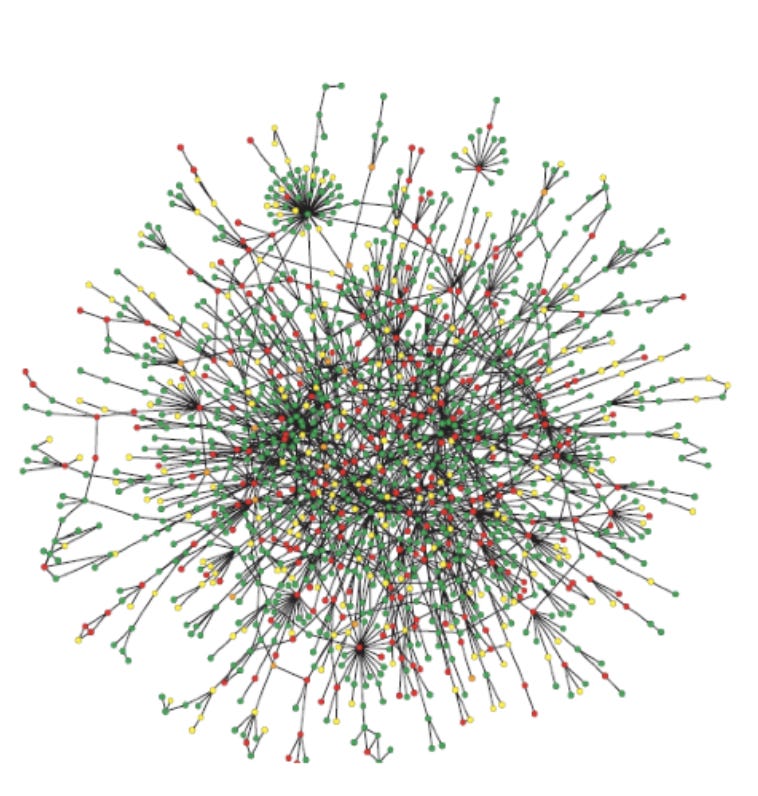
It is challenging to design new proteins that bind to specific protein targets. There are several factors that make this problem experimentally intractable, but one of the most important is the enormous search space of possible protein structures and binding modes. Computational protein design aims to leverage fundamental principles of protein chemistry and physics to systematically generate new protein sequences for experimental testing.
Methods exist for generating designs that target specific protein surface locations, but typically rely on additional information, and many proteins still can’t be can’t be effectively targeted. The Baker Lab set out to change this, with the ambitious goal of aiming to “develop a general approach to design of high affinity binders to arbitrary protein targets.”
They aimed to address two major challenges:
First, in the general case, there are no clear sidechain interactions or secondary structure packing arrangements that can mediate strong interactions with the target; instead there are a very large number of individually very weak possible interactions. Second, the number of ways of choosing from these numerous weak interactions to incorporate into a single binding protein is combinatorially large, and any given protein backbone is unlikely to be able to simultaneously present sidechains that can encompass any preselected subset of these interactions.
Again, you can see the way this is framed as a search problem. Starting from the structure of the target that you want to bind to, you have to carefully work backwards and think about all of the possible ways to design a viable solution. Previous approaches have looked for specific surfaces to start from, but that isn’t always viable. So what is a pragmatic way to go about this search? The authors of this preprint provide the following analogy to build up intuition for their proposed design framework:
To motivate our approach, consider the simple analogy of a very difficult climbing wall with only a few good footholds or handholds distant from each other. Previous “hotspot” based approaches correspond to focusing on routes involving these footholds/handholds, but this greatly limits the possibilities and there may be no way to connect them into a successful route. An alternative is to first, identify all possible handholds and footholds, no matter how poor, second, have thousands of climbers select subsets of these, and try to climb the wall, third, identify those routes that were most promising, and fourth, have a second group of climbers explore them in detail.
I’m not going to go into intensive detail here about the mechanics of their new design framework for two reasons: 1) it is very technically involved, 2) the preprint does a fantastic job walking through the design of their framework and detailing why they made they choices they did along the way. The figures also give a good visual intuition for the problem and their solution:
Overall, the key take home messages at a conceptual level are: 1) they set out to solve a really challenging but practically important problem central to biotechnology, and 2) created a design framework for efficiently searching through the enormous space of possible proteins and binding mechanisms, attempting to reduce the computational complexity of the problem as much as possible at every turn.
Results
In order to demonstrate the general nature of their new design method, the authors “selected thirteen native proteins of considerable current interest spanning a wide range of shapes and biological functions” which included human proteins involved in signaling, and pathogen surface proteins. Binding proteins targeting either class of protein would be of immense therapeutic interest.
The experimental strategy for testing the new designs was to encode a large number (15,000 to 100,000) of designs into an oligo array, and then clone them into a yeast system where they would be expressed on the surface of the cells. Fluorescently labeled targets were added, and flow cytometry was used to enrich for the designs that enabled successful binding. In order to determine which designed sequences were successful, every population of cells in the enrichment process was deep sequenced, making it possible to quantify the frequency of all designs at each iteration of enrichment.
On top of that, the team “generated high resolution footprints of the binding surface by sorting site saturation mutagenesis libraries (SSMs) in which every residue was substituted with each of the 20 amino acids one at a time.” This type of strategy helps to identify the specific relationship between each amino acid in the sequence and the final protein structure. Even further, this paper presents several crystal structures and co-crystal structures providing the highest resolution look into the designed binders that is possible.
The new design framework introduced in this preprint was able to successfully generate protein binders for all of the experimental targets selected. Here are a few graphical examples:
There is a crucial aspect of this project that extends beyond the successfully designed binders that were experimentally validated. The magnitude of the data set generated is an incredible resource for further refinement of the design strategy. The field of protein design has established a highly iterative feedback loop for empirically evaluating the performance of its methods that I think is unique in computational biology, and is a large part of why the field has progressed so rapidly in recent years.
Final Thoughts
It is an incredibly exciting time for computational protein science. There is essentially no limit to the applications that protein design could enable, considering the fact that the enormous diversity of life on Earth is based on naturally evolved proteins that have sampled a tiny fraction of the space of possible proteins. Protein design could also be a critical driver for the field of synthetic biology. Proteins designed with specific roles such as programmable switches or nanocages present an entirely new set of tools that can be composed into higher-level circuits and systems.
Another important downstream consequence of protein design is that it unlocks the potential for truly distributed manufacturing of biotechnology:
Unlike antibodies, the designed proteins can be expressed solubly in E. coli at high levels and are thermostable, and hence could form the basis for a next generation of lower cost protein therapeutics. More generally, the ability to rapidly and robustly design high affinity binders to arbitrary protein targets could transform the many areas of biotechnology and medicine that rely on affinity reagents.
It sounds like science fiction, but imagine this scenario: a novel pathogen has emerged, presenting the threat of another global pandemic. Computational protein designers rapidly design binders to various protein targets on the surface of the pathogen. These could be diagnostics, or even vaccine candidates for a rapid challenge trial. The best performing designs are made available online. Your local CVS downloads the designs, and starts synthesizing them right away to make them available. This is obviously speculative and not directly around the corner, but highlights the incredible potential introduced by the simplicity of the synthesis process for these designed proteins.1
Thanks for reading this highlight of “Robust de novo design of protein binding proteins from target structural information alone”. If you’ve enjoyed reading this and would be interested in getting more highlights of open-access papers in your inbox, you should consider subscribing:
That’s all for now, have a great Friday! 🧬
P.S.
Given the somewhat variable nature of my current research and work responsibilities, I’m shifting away from the explicit cadence of posting each Sunday. I might publish more some weeks, and less others.
Generally, the important concept here is that biotechnology could be treated like 3D printers: sharing digital designs on the Internet, and performing manufacturing/synthesis locally. There is no more impressive distributed manufacturing system than life on Earth!