Welcome to The Century of Biology! This newsletter explores data, companies, and ideas from the frontier of biology. You can subscribe for free to have the next post delivered to your inbox:
Enjoy! 🧬
AI is transforming the digital world. Machines can now interpret complex images and human language. They can also generate beautiful images and language—effectively propelling us into a world of Endless Media. While this will forever change our digital lives, the physical world hasn’t yet been impacted in the same way. One major exception has been biology. Here, I’ll make the following claim:
Biology is the most powerful way to transform the physical world using AI.
To understand why this is the case, it’s important to examine the underlying technology that we are talking about. AI is in the middle of a revolution. After several false starts and AI winters, things are finally starting to really work. AI now sits at the core of many digital products, including systems as widely used as Google Search. The major breakthrough driving this revolution is called deep learning.
Deep learning is an example of a biologically inspired algorithm—the models are called artificial neural networks. They are comprised of individual neurons that are connected in a series of layers. Models are trained to perform tasks using example data, rather than being explicitly programmed. The training process tunes the weights between neurons. The key insight in the field has been that scaling training data and the number of neurons and layers in the networks can achieve amazing results. More is different.
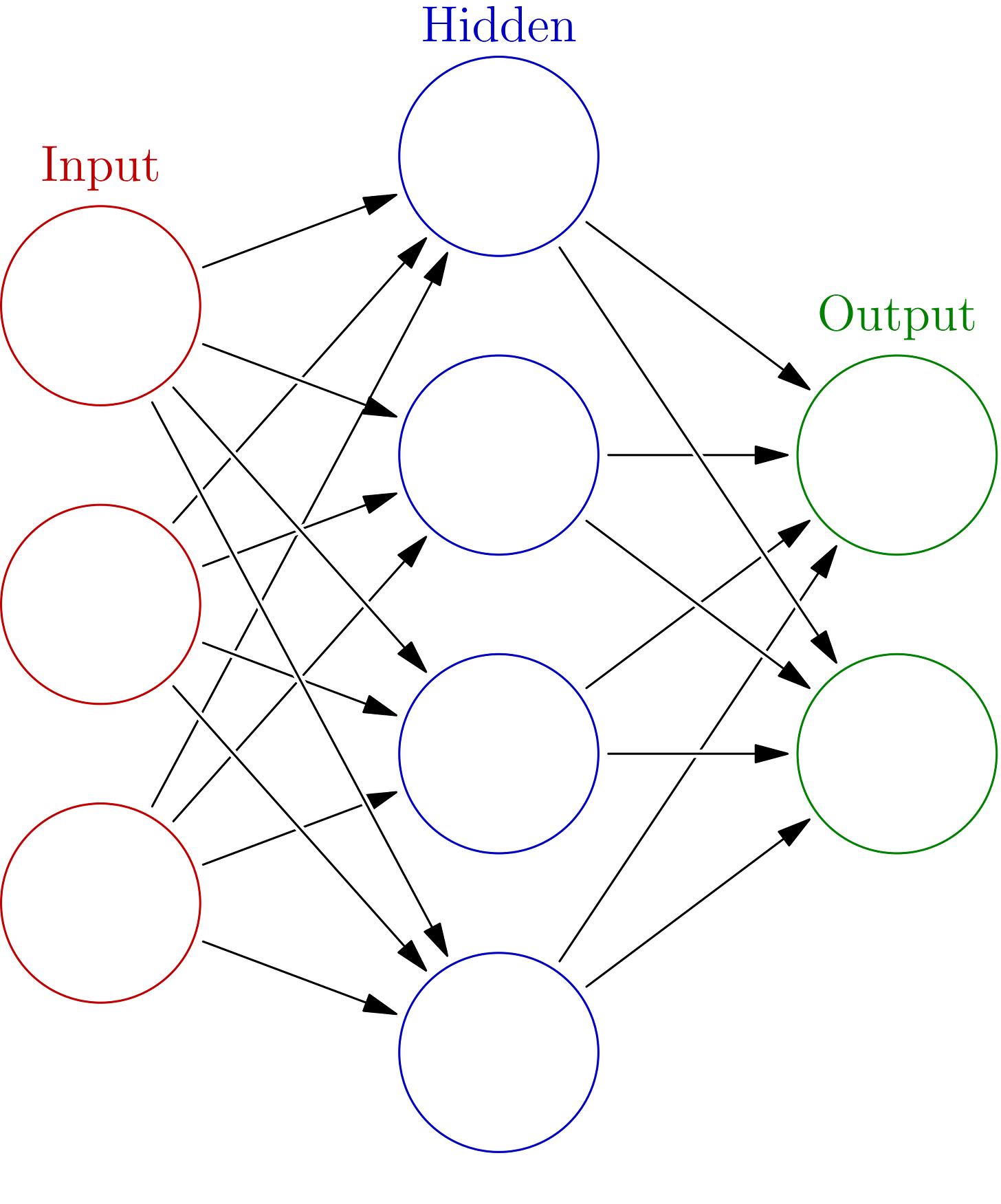
Another key detail of this model is that there are nonlinear activation functions between nodes in the network. While this seems like a small mathematical detail, it is an important departure from the classical linear models that dominate science and statistics. There is no clear linear relationship between an image and its appropriate label. By introducing nonlinearity into the picture, neural networks are capable of approximating incredibly complex functions. In fact, there are theorems that show how neural networks are capable of approximating practically any function given sufficient data and size.
Deep learning has effectively introduced a new programming paradigm. For problems like image recognition where it is practically impossible for a human to code a function that accounts for every possible edge case, we can instead train a computer to learn the approximate solution using data. Andrej Karpathy has called this process Programming 2.0.
This paradigm has produced genuinely incredible results in the digital world. I’ve been excited about AI since I started programming, but had no idea that Github Copilot would be writing nearly half of my code by 2023. I also didn’t fully anticipate the ability to generate beautiful art from text prompts using a system like DALL·E 2. As the wise Yogi Berra once said, “it’s tough to make predictions, especially about the future.”
Results have been much more mixed in the physical world. Two examples are the efforts in robotics and self-driving cars. For robotics, there has been a lot of excitement about a branch of deep learning called reinforcement learning. Progress has been much slower than people initially expected, especially for deployment outside of academic labs. OpenAI—which has produced many of the amazing systems I’ve mentioned so far—shut down their robotics program in 2021. Similarly, self-driving cars have been predicted to be only a few years away since 2012.
What explains this difference between bits and atoms? Deep learning always arrives at approximate solutions. This fuzziness works well for simulating our visual systems, or generating language—fuzziness is a lot more problematic when running inside of complex car software. In the digital world, I can always press the escape key if Copilot’s code suggestion is wrong, or generate a new image if I’m not satisfied with the first one.
In the fullness of time, I expect that these are solvable problems. Robots and self-driving cars both hold incredible promise. However, my argument is that biology is a much more natural fit for AI. In the life sciences, the fuzziness of neural networks is a feature, not a bug. Demis Hassabis, the CEO of DeepMind, made the following claim:
At its most fundamental level, I think biology can be thought of as an information processing system, albeit an extraordinarily complex and dynamic one. Taking this perspective implies there may be a common underlying structure between biology and information science - an isomorphic mapping between the two - hence the name of the company. Biology is likely far too complex and messy to ever be encapsulated as a simple set of neat mathematical equations. But just as mathematics turned out to be the right description language for physics, biology may turn out to be the perfect type of regime for the application of AI.
Evidence is quickly building in favor of this thesis. At the level of macromolecules, deep learning is already the state of the art for modeling DNA, RNA, and proteins. This is also true for predicting more complex functions at the level of cells and tissues. Biological systems are incredibly complex and nonlinear—which makes it possible for deep learning solutions to substantially outperform models that we attempt to specify by hand with math or code.
Biology has another essential ingredient for AI—an abundance of data. As I’ve argued in my Sequencing, Synthesis, Scale, Software series, we are living through a historically unprecedented time period where our instruments for decoding living systems are improving more rapidly than even Moore’s Law. As a result, biology is well on its way to being the largest data generator on the planet. This converges beautifully with the AI revolution, because the primary ingredient for making these models produce magical results is Internet-scale data.
Another important advantage for AI in biology is that living systems are much more resilient to error and noise than human engineered systems. Biological adaptation is essential for survival. Living systems are far more flexible and malleable. Human engineering often relies on “nine nines” of precision (99.999999999%) to effectively operate. Unlike self-driving cars, AI can have a massive impact on therapeutics and synthetic biology before it ever reaches this level of accuracy.
This is all very promising, because biology is the most effective way to transform the physical world. Biology literally terraformed our planet and established a global biosphere of organisms, including us. Biology is capable of planetary-scale distributed manufacturing. I’m talking about Viriditas, the “constant pressure, pushing toward pattern. A tendency in matter to evolve into ever more complex forms. It's a kind of pattern gravity, a holy greening power we call viriditas, and it is the driving force in the cosmos. Life, you see.”
As we develop increasingly powerful AI models of biology, we become stewards of this vast and enormously powerful system. This holds enormous potential for improving the physical world. Biology is capable of operating on the scale necessary to mitigate the consequences of climate change, and can move us towards more sustainable and resilient means of production. Of course, as biological systems, this will also benefit the health of our own bodies.
For proteins—which are the building blocks of biological systems—we are already seeing incredible progress. New AI models for proteins have been said to be “transforming the field of biomolecular structure prediction and design.” What will this look like as we move from proteins, to cells, and even organisms? I’m not sure, but I think that a Personal Biomaker or Anatomical Compiler—both of which seem more tangible in the AI era—would have an even bigger impact on the world than ChatGPT or its predecessors.
As the underlying science progresses, there will be enormous business opportunities. What types of business models will make sense for AI-first biology companies? One of the most knowledgeable AI investors,
, has advocated for building what he calls a “full-stack ML company.” The core idea is that the best way to capture the full economic value of a new AI model is to directly integrate it into a product, instead of licensing it to a company that creates a product with it.From this perspective, AI-first therapeutics companies (Recursion, Exscientia, etc.) are one of the most promising approaches to monetizing ML predictions. If AI can actually make better drugs faster, the path to enormous value creation is imminently clear. There will be many opportunities to create deeply integrated AI-driven biology companies even beyond drugs. AI has the potential to play an important role in accelerating R&D timelines and driving down costs for all types of bioproducts. While there will almost certainly be a lot of noise—and a rush of capital into the space—there is also a real chance for generational companies to emerge at this intersection.
Last year,
and I made several investments in cutting-edge companies using AI to accelerate biotechnology. This year, it's time to help these companies tell their stories. When meeting these brilliant founders who are dedicating their lives to building highly impactful solutions to planetary problems, it's hard not to feel optimistic. I want to share some of this optimism. Hopefully these upcoming in-depth stories will help to convince you that…Biology is the most powerful way to transform the physical world using AI.
Thanks for reading this essay about the power of AI in biology. As always, thanks to my wonderful editor Kelda. If you don’t want to miss the upcoming essays about cutting-edge AI-first biotech companies, you should consider subscribing for free to have them delivered to your inbox:
Until next time! 🧬
Really looking forward to these new stories, gonna make for fascinating reading!
Excited to hear more about the invested companies! Enjoying the articles!