Welcome to The Century of Biology! This newsletter explores data, companies, and ideas from the frontier of biology. Today, and I teamed up to tell the story of Atomic AI. You can subscribe for free to have the next post delivered to your inbox:
Enjoy! 🧬
Atomic AI: Unlocking RNA
One view of recent history is that we’ve been in a Great Stagnation since 1971. Starting with the Industrial Revolution and ending in that notorious year, humans made dizzying advances in our ability to shape Atoms into ever more powerful configurations. Then, quite abruptly, we stopped. Or rather, we shifted our innovative efforts to the world of Bits. To many, this signaled the end of real progress. The internet is useful, but Where is My Flying Car?
Now, with the dramatic rise of companies like Tesla, SpaceX, Anduril, and Moderna, this line of thinking goes, we may finally be shifting our efforts back to where they should have been all along: the physical world.
This binary battle between Bits and Atoms is not how we view the world at Not Boring. We’d bet that if you zoom out, this Bits-focused interlude will be an Archimedes lever for the progress made in the decades and centuries to come. It’s not Bits versus Atoms. The most impactful progress will come at the intersection of Bits and Atoms.
Atomic AI is a perfect case study. Atoms and Bits are right there in the name.
Atomic AI is building the foundation for AI-driven RNA drug discovery. It uses deep learning to predict the structure of RNA molecules in order to identify druggable targets. Over time, the engine they are building will also be used to design new RNA-based medicines. That engine is based on CEO Raphael Townshend’s scientific advancements in predicting the structure of RNA, which were important enough to land on the cover of Science magazine.
With his breakthrough in RNA structure prediction, Raphael had a choice: continue the work in academia or start a company to accelerate both research and commercialization. In 2021, he chose to start a company: Atomic AI. So far, it seems like a smart choice.
The company’s cross-functional team of AI researchers and structural biologists is moving quickly to build the world’s largest database of RNA structures, locate druggable targets within them, and design new RNA-based therapeutics. In 2022, the company raised a $7 million Seed round from investors including 8VC, Greylock, Factory, AIX Ventures, and AME Cloud Ventures.
Today, Atomic AI is announcing a $35 million Series A led by Playground Global with participation from its Seed investors plus Nat Friedman, Patrick Hsu, and Not Boring Capital. Atomic AI is precisely the kind of company we want to back. We’ll explain why in today’s piece. As a teaser, Atomic AI has one of the best answers to our “So What?” question of any company we’ve met.
If Atomic AI is successful, it will unlock the potential of RNA-targeting and RNA-based medicine. That might mean cures for cancer, more efficient, effective, and safer vaccines, and the opportunity to save and improve millions of lives. Many of the diseases Atomic AI is going after are currently undruggable.
Atomic AI’s capabilities read like a sci-fi novel. The company opens up futuristic possibilities for how we treat diseases, but it’s rooted in the history of progress across Bits and Atoms. Without the advances in deep learning over the past 30 years, it wouldn’t be possible to understand RNA at the atomic level with precision, speed, and scale. Attempts to date have been slow, manual, laborious games of whack-a-mole. And without the advances in understanding the building blocks of life over the past 70 years, AI would be missing its most powerful way to transform the physical world.
It’s the latest in a long tradition of advances in understanding the structure of the molecules that make us who we are so that scientists can improve how we function.
Chapter 1: Structure and Function
DNA’s double helical structure is one of the most iconic and widely recognized scientific images of all time.
This outsized level of recognition is warranted. Since the publication of the double helix in 1953, biology has been irreversibly transformed. With the structure of DNA solved, a domino cascade of foundational discoveries followed. The timeline of these rapid discoveries reveals a deep truth about living systems: structure is inextricably linked to function.
The fact that DNA is a double helix with two separable strands gave direct clues into how information was replicated between cells in the body, and ultimately between parents and their offspring. By 1957, Francis Crick presented on the central dogma of molecular biology, which describes the flow of information from DNA to RNA to proteins. Only a year later, scientists empirically demonstrated the mechanism of DNA replication.
By the middle of the 1960’s, the genetic code—how heritable information is actually stored in DNA—was cracked. It took slightly longer than a decade for the discovery of the double helix to completely change our understanding of biology.
The double helix is considered to be the starting point for the field of structural biology. The speed at which massive problems in genetics were solved based on the double helix caused a ripple effect throughout the life sciences. It became possible to work at the atomic scale. Atomic structures of molecules and proteins inside of cells gave scientists valuable clues about their function.
The molecular biology revolution has transformed our view of living systems. Diseases are no longer viewed as unexplainable mysteries, but as molecular programs gone awry. If crops underperform, farmers reach to genetically modified organisms as their primary solution. Answers about ancestry and health risks are waiting to be read out from our DNA by consumer offerings. The entire biotechnology sector—which now produces north of $500 billion in annual revenue in the U.S. alone—is a result of this paradigm shift.
The pharmaceutical industry—which has incumbents that have been around for centuries—required disruption to adapt to the new molecular world order. One of the most iconic stories of this era is the journey that Joshua Boger took to form Vertex Pharmaceuticals, which Barry Werth chronicled in The Billion-Dollar Molecule.
With an impressive background as a chemist, Joshua Boger started his career as a scientist at Merck & Co. in 1978. From the very outset, Boger arrived at Merck with an iconoclastic attitude and a unique view of how drug discovery should work. At the time, drug makers were primarily focused on making new molecules—the structure of the drug targets that molecules actually bound to was a secondary consideration. Boger thought in reverse.
He was fascinated by the power of structural biology, and wanted to use knowledge of the structure of drug targets to guide the design process of new therapies. If knowing the molecular structure of DNA transformed genetics, shouldn’t the same revolution be possible within drug discovery?
In his very first project at Merck, Boger used information from X-ray structures of enzymes to design a renin inhibitor that was 1,000 times more potent than Merck’s existing drug. An analogy here is that drug discovery is like finding a key (drug) that fits into a lock (drug target). Boger was intensely focused on using the structure of the lock to accelerate this process.
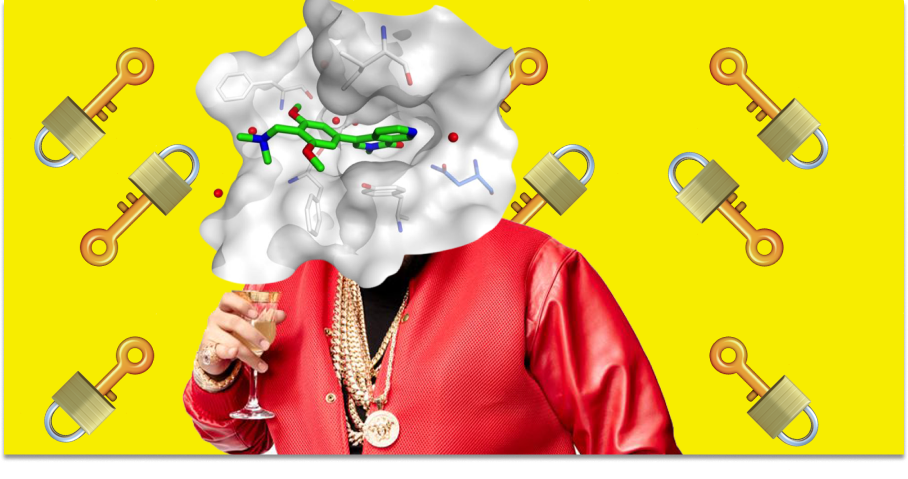
Boger left Merck to found Vertex Pharmaceuticals in 1989. The goal of Vertex was to place rational drug design—the intensive computer modeling of target structures and the chemical binding of drugs—at the center of the drug discovery process. This transition was a major success. Both Vertex and the broader rational design thesis have had a substantial impact on the drug discovery industry. By leveraging structural biology, Vertex developed the first drug to treat the underlying genetic cause of cystic fibrosis. Vertex has been one of the top performing stocks in the S&P 500—it has compounded at 14.3% since its 1993 IPO—and its influence has been felt far beyond Wall Street. Effectively all major pharma companies have worked hard to expand their efforts in structure-based drug design.
The broad success of structural biology as a discipline extends beyond the double helix and the advent of rational drug design. Over the years, many technologies rapidly improved. It became easier to produce proteins, crystallize them, and solve their structures. X-ray based techniques became much easier and more accurate, and computers got much faster. Entirely new technologies like electron cryogenic electron microscopy came on the scene. As researchers solved more and more structures, they poured them into the Worldwide Protein Data Bank (PBD).
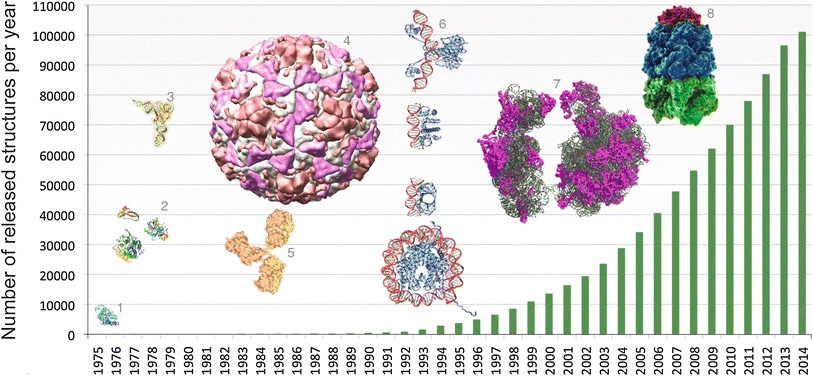
As the PDB grew, scientists began to wonder if it would be possible to solve the longstanding problem of protein structure prediction. Would it be possible to learn the rules of biophysics that dictate the shape that a linear string of amino acids—the building blocks of proteins—will fold into?
Even with improvements to measurement tools, experimentally solving protein structures is more of an art than a science. Countless hours of expert work are still spent tweaking and refining models. Being able to computationally predict accurate protein structures could accelerate structural biology and take the field to another level. Every two years, scientists participated in the Critical Assessment of protein Structure Prediction (CASP) challenge to benchmark progress on this problem.
The field steadily marched forward over the years, until DeepMind made an entry and things started to get really interesting really fast.
Chapter 2: AI’s Bio Breakthrough
AI is starting to produce truly magical results—whether in the world of images, language, or games—but to do any of this, it needs a massive amount of data. State-of-the-art models like GPT-3 are trained on Internet-scale data, ingesting all of the words on the Web in order to produce interesting outputs.
Most fields in the natural sciences don’t have Internet-scale data, or anywhere close to it. At the London-based AI research lab DeepMind, Demis Hassabis was acutely aware of this challenge. With a background as a child chess prodigy, game developer, and academic neuroscientist (seriously), Hassabis had built DeepMind into a world-class AI lab. After his team built AI models that demonstrated superhuman performance at computer games, Google purchased the company for north of $500 million in 2014.
But while beating computer games and selling for half a billion dollars were impressive feats, this initial success didn’t meet Hassabis’s ultimate goal of using AI to solve real-world problems. DeepMind needed to find a meaningful and challenging scientific problem that would also be amenable to AI.
Where to look? Historically, deep learning has produced incredible results for well-benchmarked problems with large amounts of data. One of the major inflection points for the adoption of the technology was when a deep learning model dominated the ImageNet computer vision challenge in 2012, blowing other approaches out of the water. This challenge was a perfect opportunity because there was a massive amount of labeled training images, and incredibly clear metrics for success.
After searching for problems with similar characteristics, Hassabis and DeepMind found the CASP protein challenge. It met all the criteria. There was a large amount of annotated protein data for training, and a clearly defined and challenging prediction problem. Could DeepMind produce a moment equivalent to the ImageNet challenge, but on a major open problem in the life sciences?
The answer was yes.
In 2018, DeepMind entered the contest and won by a sizable margin. By the next contest in 2020, they produced such a dominant performance that their model—called AlphaFold—is widely considered to have solved the problem of structure prediction. The success with AlphaFold landed Hassabis and John Jumper the 2023 Breakthrough Prize in the Life Sciences.
Being able to predict the structure of proteins is a big deal for biotechnology. As we’ve seen, there is a deep connection between structure and function in biology. With these models, it’s now possible to explore the total space of protein structures on a scale that was previously unimaginable. In this renaissance, approaches to designing proteins with entirely new functions—like enzymes that degrade plastic, or universal flu vaccines—have also dramatically improved. This massive step change in modeling has been said to be “transforming the field of biomolecular structure prediction and design.”
Structure leads to function, and new understanding of structures leads to new bursts of productive activity. With protein structure prediction effectively solved, we are now seeing an explosion of academic and commercial efforts to design new protein-based drugs, vaccines, and biomaterials.
In his 1986 book Engines of Creation: The Coming Era of Nanotechnology, the scientist Eric Drexler envisioned a future where matter could be controlled and programmed at the atomic scale. For the most part, this vision hasn’t happened—with the exception being biology. While the “universal assemblers” that Drexler imagined don’t exist (yet), protein engineers are using AI to design molecular machines with atomic precision in labs all around the world, right now.
Based on the success of AI for a problem as challenging as structure prediction, Hassabis articulated a compelling vision for the future at the intersection of AI and biology:
At its most fundamental level, I think biology can be thought of as an information processing system, albeit an extraordinarily complex and dynamic one. Taking this perspective implies there may be a common underlying structure between biology and information science - an isomorphic mapping between the two - hence the name of the company. Biology is likely far too complex and messy to ever be encapsulated as a simple set of neat mathematical equations. But just as mathematics turned out to be the right description language for physics, biology may turn out to be the perfect type of regime for the application of AI.
Despite this enthusiasm, there were still important open questions to be addressed. The quality, centralized organization, and scale of data for protein structures is an anomaly in the life sciences. Living systems are indeed extraordinarily complex, experiments are bespoke and highly specific, and the data collected is noisy. If deep learning required the Protein Data Bank to achieve success in protein structure prediction, would it ever work for other challenging problems with far less data?
Here, a talented computer scientist whose curiosity had propelled him into structural biology—and an internship on the AlphaFold team—had a very strong opinion about a new frontier for AI and biology. His name was Raphael, and he was convinced that despite the lack of available data for the problem, RNA structure prediction would be the next chapter of the Atomic revolution.
Chapter 3: Raphael’s Journey to Atomic AI
Raphaël John Lamarre Townshend was born in Montreal, Quebec into a family of engineers of all stripes: civil engineers, mechanical engineers, electrical engineers. The family was so full of engineers that his parents gave their son the name Raphaël in hopes that he would become an artist. He did not.
Instead, a self-described “huge nerd and introvert” whose family shuttled back and forth between Montreal and Silicon Valley, Raphael spent most of his free time during high school at Menlo School in the computer lab. For fun, he tried to program his own version of the popular game Civilization. In a juicy bit of foreshadowing, he started a Diplomacy Club, pulling teachers and fellow students in to play the strategy board game that Meta’s Cicero would master with AI last year.
When it was time to go to college, Raphael decided to stay in California, attending Berkeley to study Electrical Engineering and Computer Science with a concentration in Statistical Learning. He stayed for graduate school, too, attending Stanford to get a PhD in Computer Science in the Stanford AI Lab.
A lot of Raphael’s early work was in computer vision, specifically on self-driving cars, 3D vision, and large-scale geolocation. But while he viewed self-driving as an interesting and important problem, he had an epiphany: “I realized that a lot of people had the same skill set and were working on the same problem. I could work on it too, but I’d probably just be a drop in the bucket.”
Raphael didn’t want to be a drop in the bucket. He wanted to differentiate himself. So, taking inspiration from Open Philanthropy, he applied three criteria in his search for the best use of his time:
Importance
Neglectedness
Tractability
In other words, which high-impact problems were very few people working on that he could actually help solve? Which lock did his key fit best? Or as he put it, “I wanted to cast a wide net and figure out where my AI hammer could do the most damage.”
He explored computer networking, genomics, and a host of other areas before, like Hassabis, finding his target in structural biology. It was chance meeting a prepared mind. As part of his PhD program, Raphael rotated through departments, and one rotation took him to the laboratory of Ron Dror. During the rotation, he didn’t do any AI at all, he just tried to understand structural biology. And the more he learned, the more he realized that it was exactly what he was looking for:
Importance: Opportunity to design drugs that could save millions of lives.
Neglectedness: “There were like three people doing ML in structural biology at the time.”
Tractability: Structural biology had huge amounts of homogenous data that lent itself well to machine learning.
Raphael dumped computer vision and started working at the intersection of structural biology and AI, atoms and bits. His key clicked.
By May 2018, Raphael contributed to his first structural biology paper, Molecular mechanism of GPCR-mediated arrestin activation, which was published in the prestigious journal Nature. The following summer, he ventured out from the biology lab and into the heart of AI research with an internship on DeepMind’s AlphaFold team in London in the summer of 2019, an extremely important period on the path to AlphaFold 2.
There, he experienced first-hand how a team of AI experts with little training in biology were able to solve the protein structure prediction problem that had taunted scientists for half a century. The magic, he recognized, was in how the organization was set up, or, to make it more coherent with our narrative, in DeepMind’s structure.
Specifically, two features stood out to Raphael:
Tight Iteration Cycles. Within two days of starting, he was able to spin up projects that used 1000s of GPUs. The team moved much more quickly than an academic lab.
Team-Based Science. DeepMind put together teams of ten world-class people who could focus on problems for several years. That wasn’t possible in academia or pharma.
He also saw one drawback to DeepMind’s approach: they didn’t have their own wet labs in-house. So when they had to get new data from external labs, iteration times slowed significantly, dragged down by the secretiveness and legal overhead of working with outsiders from within a very large organization. Noted.
Back at Stanford after the internship, Raphael returned his focus to the challenge of RNA structure prediction. Most people didn’t think that the problem was a good fit for AI. Other groups had steered away from the problem because of the lack of RNA structure data. Biologists have historically spent little time deeply thinking about RNA structure, assuming RNA was mainly a floppy string of bases carrying a message from DNA for the production of proteins. Thankfully, Raphael ignored everybody and got to work.
If we think back to the Protein Data Bank, DeepMind and the CASP community had over 100,000 protein structures to train models with. Raphael had only 18 RNA structures to work with. Despite this serious data scarcity, Raphael developed extremely clever techniques to produce a state-of-the-art deep learning model for RNA structure prediction. In August 2021, his work, Geometric deep learning of RNA structures, landed on the cover of Science Magazine, an honor few scientists ever experience in their career.
The core insight that Raphael had was that techniques from geometric deep learning would make it possible to represent the 3D coordinates of molecules in a way that was rotationally equivariant. In plain English, for any transformation to the 3D shape of the input structure, the output would be equivalently transformed. This highly creative machine learning advance made it possible to learn a state-of-the-art model with only 18 structures, a feat that most people in the field wouldn’t have considered possible. When thinking back on the initial study, Playground Global general partner Jory Bell felt that “the AI alone was impressive enough that Atomic was investable even without a data platform.”
From here, Raphael had a decision to make. This type of discovery and acknowledgment made him a shoo-in for a top faculty position at a research university. As he watched the RNA revolution unfold, it was clear that this type of technology had profound commercial promise—should he start a company instead? The model represented a potential solution to one of the major bottlenecks for targeting and designing RNA. It could potentially be a new superpower for drug designers targeting RNA, and for engineers developing the next generation of advanced therapeutics.
Ultimately, his experience at DeepMind convinced him that he needed to start a startup, because it was the only way to build an organization with the tight iteration cycles and team-based science required to crack the problem.
By starting his own company, Raphael could build a more tightly integrated version of what DeepMind had built with AlphaFold. He could bring the wet lab in-house and put machine learning engineers in the same room as structural biologists. He could capitalize on RNA’s perfect fit with AI. You can’t sequence proteins, and synthesizing them is very difficult. But you can sequence and synthesize RNA, generating large amounts of data to feed the models in a tight, virtuous cycle.
When all of that clicked two years ago, he told us, he knew in his gut: “If I don’t take this bet, I’m going to regret it.”
He took the bet. It’s called Atomic AI.
Chapter 4: Atomic AI Vision
During COVID, something happened that would have been practically impossible to predict—the #mRNA hashtag went viral on Twitter. As Pfizer / BioNTech and Moderna deployed their vaccines that used messenger RNA molecules to program the cellular production of COVID antigens, molecular biology became a topic of dinner table conversation around the world.
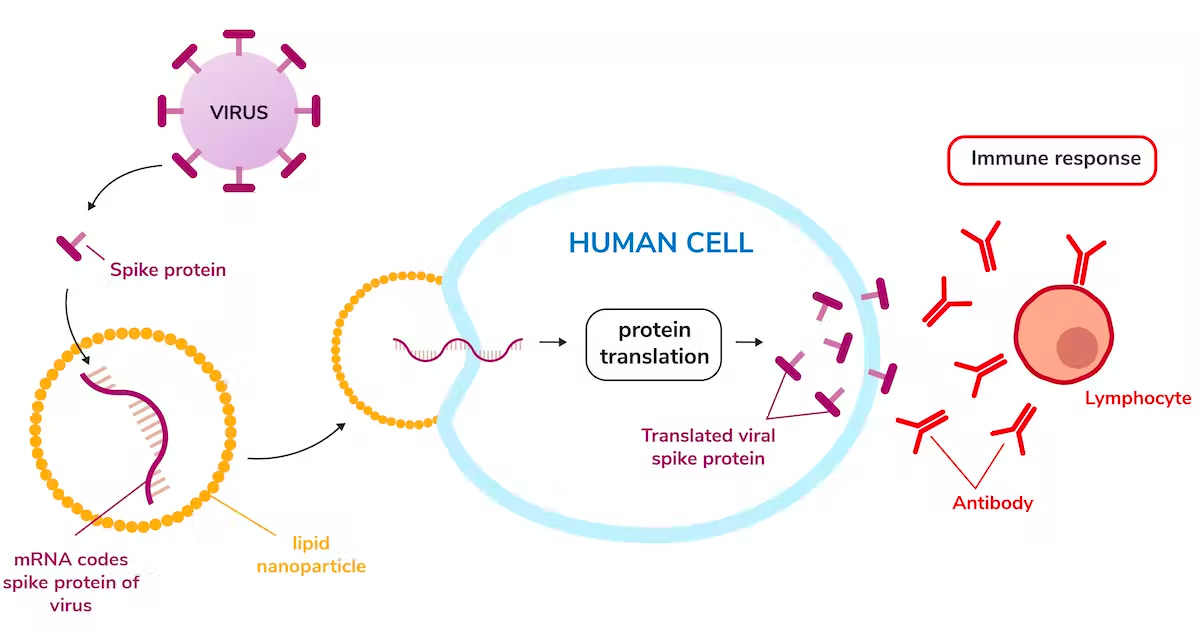
The rapid design and deployment of mRNA-based COVID vaccines was a breakthrough moment for a technology that was several decades in the making. Because of years of painstaking research followed by intensive commercial R&D, companies like Moderna had the infrastructure in place to computationally design vaccines in a matter of hours—and to physically manufacture them at scale as soon as the emergency approval was granted.
This story highlights two key properties of mRNA:
mRNA is Programmable. It’s possible to encode any desired protein product in a messenger RNA sequence. As soon as molecular information about the COVID virus was available, new vaccines could be quickly designed with existing technology.
mRNA Production is a Platform. The infrastructure built to synthesize and manufacture one mRNA vaccine can immediately be reused for another.
The success of mRNA-based COVID vaccines generated a renewed interest in the elegance and utility of this therapeutic modality. Moderna has already produced initial evidence that their platform won’t be a one-hit wonder with promising trial results for cancer vaccines.
The centrality of RNA within the flow of information inside of cells also makes it a promising therapeutic target. The logic is the inverse of mRNA vaccines—which introduce RNA molecules to prompt protein production. One of the primary goals of RNA-targeting medicines is to inhibit the translation of RNA molecules into proteins that cause disease—stopping the process completely in its tracks before it ever develops.
Just like mRNA vaccines, there are extremely strong tailwinds for RNA-targeting medicines. In August 2020, the FDA approved Evrysdi, the first oral medication for Spinal Muscular Atrophy (SMA). SMA is a debilitating genetic disease that causes the loss of motor neurons necessary for movement. Patients with SMA present with severe weakness and muscle loss. The only other approved treatment for SMA is an antisense nucleotide therapy that requires a painful spinal injection to administer. Evrysdi on the other hand, is a small molecule that disrupts the RNA transcript encoding the message of the mutated gene. As a small molecule, the drug can be sold as “little pills in bottles with white cotton on top” as Joshua Boger of Vertex liked to say, which is a massive change for SMA patients.
This example shows some of the beauty of RNA-targeting small molecules. There are diseases where protein targets are undruggable. It is sometimes possible to develop therapies for these diseases using complex biologic therapies, but these approaches can come with drawbacks. Targeting RNA opens up a totally new druggable space for small molecules to bind, bringing them back into the equation.
RNA is poised to play a central role in the future of medicine—both as a drug target and as a modality—but in order for this revolution to fully take off, we need a much deeper understanding of RNA structure. As we’ve seen with the story of the double helix and Vertex’s success with rational drug design, structure and function can’t be separated in biology. This is no different for RNA. In a recent review on RNA-targeting drug discovery, leaders in the field wrote, “an accurate model of RNA structure is key to the design or discovery of small molecules that modulate its function.”
Two paragraphs later, Raphael’s model is highlighted as the state-of-the-art AI approach to RNA structure prediction. Through a combination of careful problem selection, extremely hard work, and a nose for high impact, Raphael found himself again at the intersection of two intertwining threads—the AI revolution and the RNA revolution.
Now, Atomic AI is on a mission to bring atomic precision to the world of RNA drug discovery.
Atomic AI is built from the ground up based on Raphael’s scientific expertise and his experience seeing what it took to create a scientific breakthrough like AlphaFold. To start, the foundation of Atomic AI is a world-class team of scientists with deep experience in both machine learning and RNA biology. In order to establish a culture with in-depth collaboration across disciplines, the team works out of a lab in the SF Biohub where they can freely whiteboard ideas and test their predictions at the bench.
The deep integration between the dry lab (AI researchers) and wet lab (RNA biologists) is one of the defining characteristics of the company. Atomic AI is a world-class instantiation of the Sequencing, Synthesis, Scale, Software model. Here’s how:
Sequencing. With a rapid decline in DNA sequencing costs (faster than Moore’s Law), there is an abundance of information about potential genetic targets. Sequencing also makes it possible to generate a large amount of data for DNA, or for any molecule that can be copied into DNA—such as RNA.
Synthesis. DNA synthesis costs have also declined exponentially, large numbers of DNA and RNA molecules can be rapidly produced in the lab for testing.
Scale. Blending Sequencing and Synthesis enables the generation of datasets at an entirely new Scale.
Software. The Scale of data requires a totally new software stack, especially AI models capable of capturing complex biological relationships.
The entire company is structured around this deep integration, and works across every layer of the measurement and modeling stack. While the AI model Raphael originally developed is already state-of-the-art, Atomic is an engine precisely designed to take RNA drug discovery to the next level.
This engine has the potential to bring the power of rational structure-based design to the world of RNA drug discovery. The implications are enormous. The undruggable could become druggable. By targeting RNA, undruggable cancer drug targets like MYC and KRAS—which are overexpressed in 75% of cancers—can be revisited and potentially blocked before the protein is ever even produced. This example isn’t cherry picked. Currently, small molecules and antibodies only target 0.05% of the human genome—meaning that most diseases don’t have defined drug-binding sites.
Atomic AI is working to change this fact. By leveraging the immense progress we’ve made in the world of Bits, the team is building a springboard to launch the entire world of RNA medicines into a new stratosphere. What if we could rapidly design therapies for undruggable diseases with Atomic precision?
Chapter 5: The Business of Billion-Dollar Molecules
Not every scientific breakthrough, however, is an investable opportunity. Watson and Crick raised no venture funding in their hunt for the structure of DNA. But we believe that Atomic AI has the potential to build a multi-billion dollar business by implementing a modern techbio business model.
To appreciate what’s different about the modern techbio business model, it’s useful to understand the traditional biotech model.
Crypto is often likened to a casino, but long before Satoshi dropped his Bitcoin whitepaper, biotech investing was the ultimate slot machine. Asset-centric biotech companies with a promising therapy and success in Phase I and/or Phase II trials often go public in order to fund more expensive Phase III trials, and investors gamble on the success of the therapy in those trials. Results are close to binary. If it passes Phase III, the stock soars. If it fails, the stock tanks. Single asset plays are even riskier at the early stages.
The risk can be worth it because the outcomes can be enormous. The highest grossing drug in the world, AbbVie’s Humira did $19.8 billion in revenue in 2020 alone. Pfizer’s Lipitor has generated over $150 billion in lifetime sales, and still generates $2 billion per year despite its patent expiring in 2011. With its mRNA-based COVID vaccines, Moderna generated $12.2 billion in net income on $18.5 billion of revenues in 2021.
Source: Fierce Pharma
As a result, the sensible approach to investing in biotech companies, whether in the public markets or a venture portfolio, has been to build a diversified portfolio of bets. Many will fail, but the ones that make it can carry the portfolio many times over.
Atomic AI’s model is different, and we think superior, in a few ways.
Internalize the Portfolio. Instead of developing one therapeutic and becoming a piece of a portfolio (or a big pharma acquisition target), Atomic AI plans to develop its own internal portfolio of hundreds of druggable RNA structures and therapeutic designs in the next few years. Importantly, the structure discovery engine makes it possible to work on RNA targets that were not druggable before Atomic AI.
Increased Precision, Lower Cost. By better understanding the structure of RNA molecules, Atomic AI should see a higher success rate among its targets, and by using AI in place of teams of scientists working through trial and error, it should be able to identify those targets and design RNA-based therapeutics more quickly and cheaply.
Atomic AI is an example of a platform company, which we described in Techbio Taxonomy: “Platform companies focus on a technological approach or system that should enable them to make multiple new drugs.” While they might be less familiar to the biotech uninitiated, platform companies are nothing new—The Column Group generated a 397% IRR from 2007-2015 investing in “drug discovery companies with unique scientific platforms.” What makes techbio startups like Atomic AI unique is their deep integration.
In Atomic AI’s case, deep integration is built into the company’s … errr … DNA. The ability to build an organization that combines computation and wet lab for faster iteration cycles is why Raphael founded Atomic AI in the first place. As he explains it, “We’re convinced that the smoother we can make the tight integration cycle of wet lab to computation, the faster we can move.”
That should mean identifying more druggable structures and designing better RNA-based drugs more quickly and accurately. That’s the core of Atomic AI’s business model innovation, and we’ll go back to the casino analogy to explain why it’s so powerful.
A single-asset biotech company equates to putting all of your chips on black. Atomic AI’s technology changes the strategy by making it possible to place hundreds of smaller bets, creating a more diversified portfolio of options. Importantly, each bet requires fewer chips because of the scaling properties of the platform. Over time the goal will be to prove the thesis that these bets each also have a higher probability of success by operating with atomic precision. Ultimately, with the same chip stack, Atomic AI should be able to get an order of magnitude more diversification and upside.
Of course, bringing those options to fruition by going through the entire drug development process is overwhelmingly expensive for a Series A company. It can take 10-15 years and an average of $985 million to bring a drug to market. Multiply that by 100 and you can see that the $35 million Atomic AI raised in its Series A falls a little bit short.
Instead, the company plans to partner with pharma companies for the clinical development of its early assets in the near-term. These partnerships generate revenue in three ways:
Upfront Payments. Typically $5-10 million per target, with multi-target licensing agreements reaching as high as $190 million (Arrakis x Roche).
Milestone Payments. Pharma companies pay licensees as drugs reach certain development, regulatory, and milestones. These can be as high as $2 billion (Skyhawk x Genentech), but can take many years to hit and are often back-loaded.
Royalties. If a drug makes it to market, licensees can earn royalties on sales, typically in the ~5-10% range (although sometimes royalties can be much higher).
To give a sense of the opportunity, Evrysdi—the small-molecule RNA-targeting drug for SMA we looked at earlier—generated $500M of revenue in 2021 and is projected to generate $1.7B of revenue a year by 2024. Assuming the drug is in market for ten years before its patent expires, upfront, milestone, and royalty payments on a single drug like Risdiplam could potentially be worth nearly $2 billion in revenue for Atomic AI over the life of the drug.
Upfront: $7.5 million upfront payment to partner on the target.
Milestone Payments: Assuming $500 million, which is conservative.
Royalty Payments: Assuming 10% royalties, could reach $1.5 billion over ten years.
Atomic AI hopes to identify hundreds of druggable RNA structures—and ultimately small molecule assets—over time. Even getting to upfront payments on 20-50 targets would provide enough revenue to scale the business in a capital efficient manner, and getting into milestone and royalty payments on multiple assets would create a massive business.
Additionally, because its structure database is useful for both targeting and designing RNA, it should also be able to generate revenue by partnering with pharma companies to design better RNA-based therapies.
As an example, it might help design drugs with more efficient payloads—packing as much RNA into each drug as possible—to bring down the cost of manufacturing. As another, it might help design mRNA vaccines that don’t need to be kept cold, like Moderna’s COVID vaccine does, increasing stability and lowering logistics costs.
The combination of RNA-targeting drugs and therapeutic RNA design diversifies Atomic AI’s bets even further, and in a way builds products with two tranches of risk:
Zero-to-One: Develop RNA-targeting small molecule drugs against targets that were undruggable before Atomic AI unlocked the structure of RNA.
Scale: Improve many properties—such as efficiency and stability—of existing RNA-based therapeutics.
Getting these revenue-generating engines humming will mean that Atomic AI can produce cash flows in the short-term which it can invest in tightening its loops and growing its capabilities without relying on the good graces of lady luck or VCs.
Over time, the Atomic AI vision is to expand ownership of the drug discovery pipeline as the platform matures. This is a path towards becoming a generational company and landing among the fifteen biotech companies with market caps north of $100 billion (Vertex is #16 at $80B and Moderna is #19 at $75B).
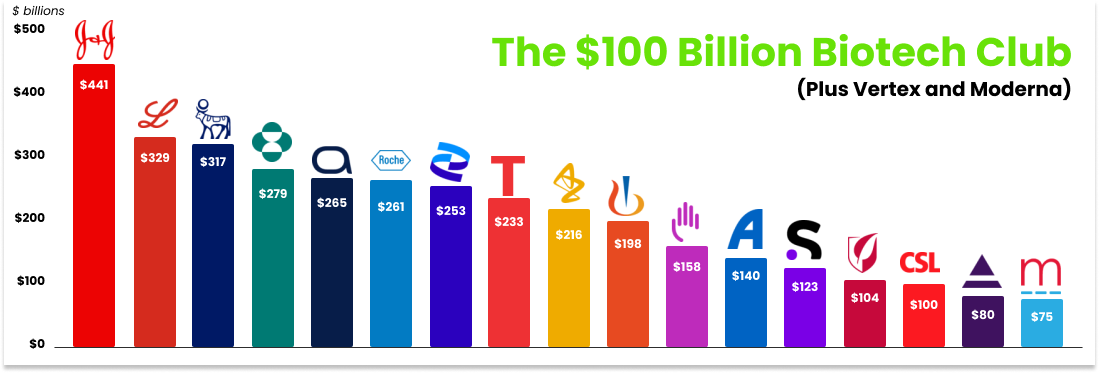
We’re excited about the potential for a full-stack future, but it’s not required for Atomic AI to become a multi-billion dollar company or a 100x investment for Not Boring Capital.
Chapter 6: Atomic AI Answers the Three Questions
When we’re considering an investment at Not Boring Capital, we ask three questions:
How hard is it?
How much of the value it creates can it capture?
So what?
Atomic AI answers all three questions with flying colors.
How hard is it?
Atomic AI is textbook Hard Startup. It sits at the intersection of Bits and Atoms and pushes the frontier on both fronts. The core science was so challenging that it landed on the cover of Science. The AI model was able to do something similar to what DeepMind did for proteins with a lot less data.
In one of the pieces that helped crystallize our Hard Startup thesis, When Tailwinds Vanish, Founders Fund’s John Luttig wrote that there are four risks “VCs will continue to be uniquely good at taking”:
R&D risk – can this technology be built?
Founder risk – can this team build it?
Vision risk – can this idea become huge?
Macro risk – will this startup win in 2030’s political, economic, and competitive climate?
Here, too, Atomic AI stands out. Atomic AI has built, and continues to iterate on, cutting edge technology. There’s no founder or team better suited to solving this particular problem. If and when they do, there are paths to $10 billion+ and $100 billion+ outcomes. And there will always be a market for more effective drugs – the Nasdaq Biotechnology Index has dramatically outperformed the Nasdaq over the past year, returning 6.5% while the broader index fell 21.2%.
Of course, hard means risks. For Atomic AI specifically, the biggest open risk is that they’ve yet to actually license any assets to pharma companies. The first such agreement will be a big milestone. Once licensed, there’s the risk that its designs don’t yield results, which would limit revenue to upfront payments instead of more lucrative milestone and royalty payments, and significantly hamper the company’s ability to sign future licensing agreements. Even in the success case, it will be many years before Atomic AI sees milestone and royalty payments.
Beyond licensing, a big risk awaits in the future, when Atomic AI shifts its focus from licensing to owning more of the drug discovery and development process. That shift will require a lot of capital and skills that the company doesn’t currently possess.
Finally, there’s the existential risk facing any AI company, and maybe any company at all, that AGI obviates the need for vertical-specific applications of the technology. What if we get one model to rule them all? This isn’t a risk we’re too concerned about—and it’s not specific to Atomic AI—but it’s worth mentioning.
How much of the value it creates can it capture?
This is an easy one to answer, and we’ve discussed it at length. If Atomic AI is able to discover RNA structures druggable by small molecules and license the assets to pharma companies, it will be able to generate anywhere from $5 million to $2 billion per drug, depending on how much value it actually creates. If its work leads to drugs that come to market and are commercially successful, it will get a cut of that success while its partners take on the high costs of drug development. If it can identify RNA-based drug designs that are cheaper, more efficient, and more stable, partners will pay for those attributes.
The question remains as to whether they’ll be able to take more of the process in-house, and capture more of the value, over time, but as discussed, we believe that’s the difference between a $10 billion and $100 billion outcome.
So what?
If Atomic AI succeeds, will it bend the world’s trajectory upwards in a meaningful way?
Here, the answer is a resounding yes. Before Atomic AI was even a glimmer in his eye, Raphael sought out a challenge that, if solved, would be important. He found it in structural biology, and ultimately in RNA structure prediction.
If Atomic AI is successful, it might help cure cancer by enabling drugs that target the MYC and KRAS genes involved in over 75% of cancers. Other therapeutics it designs might help cure or treat any number of currently untreatable diseases that cause human suffering and death. Even diseases that are currently treatable through surgery or injection might be treated more painlessly through more effective pills. Even in a mild success case, Atomic AI can help solve cold chain or cost challenges that limit the effectiveness and reach of existing mRNA therapeutics.
As Raphael explained to us, finding the right locks “may be the key to saving millions of lives.”
All told, Atomic AI is exactly the type of company that we want to back at Not Boring Capital, and exactly the kind of story that we want to tell. We expect that this will be the first of many times that we write about Atomic AI as Raphael and the team knock down milestones over the next decade and beyond.
Thanks for reading this essay about Atomic AI. Thanks to Packy for writing this with me, to Raphael for letting us come on the Atomic AI journey, and to Dan for editing! If you don’t want to miss the next post, you should consider subscribing for free to have them delivered to your inbox:
Until next time! 🧬
Very thorough article and my hunch is you've potentially found a futuristic method of printing money and helping massive amounts of people.
Can small investors get involved?